Intuitionistic fuzzy solid assignment problems: a software-based approach
- Original Article
- Published: 23 May 2019
- Volume 10 , pages 661–675, ( 2019 )

Cite this article
- P. Senthil Kumar ORCID: orcid.org/0000-0003-4317-1021 1
310 Accesses
41 Citations
2 Altmetric
Explore all metrics
This paper sustains a sound mathematical and computing background. In this paper, the software-based approach for solving intuitionistic fuzzy solid assignment problem (IFSAP) is presented. The IFSAP is formulated and it is solved by using Lingo 17.0 software tool. Theorems related to IFSAP is proved. The IFSAP and its crisp solid assignment problem both are solved at a time and their optimal solution is obtained. In addition, the optimal objective values of both the IFSAP and its crisp solid assignment problem (SAP) are estimated with the help of substituting the optimal solution(s) to their respective decision variables in the objective functions. Some new and important results are proposed. To illustrate the efficiency of the proposed method the numerical example is presented. The reliability of the proposed results are verified by using the numerical example. Strengths and weakness of the paper is mentioned. The novelty of the analysis is given into a coherent, concise, and meaningful manner of analysis. Social issue (real-life problem) is converted into a mathematical model and it is solved by the proposed method. At the end, the advantages of the proposed algorithm is explained.
This is a preview of subscription content, log in via an institution to check access.
Access this article
Subscribe and save.
- Get 10 units per month
- Download Article/Chapter or eBook
- 1 Unit = 1 Article or 1 Chapter
- Cancel anytime
Price includes VAT (Russian Federation)
Instant access to the full article PDF.
Rent this article via DeepDyve
Institutional subscriptions
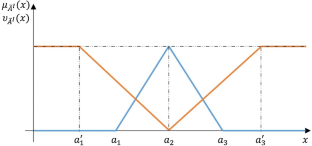
Similar content being viewed by others
A Distinct Method for Solving Fuzzy Assignment Problems Using Generalized Quadrilateral Fuzzy Numbers
Linear Programming with Interval Type-2 Fuzzy Constraints
Solving fully fuzzy multi-objective linear programming problem using nearest interval approximation of fuzzy number and interval programming.
Abhishekh, Nishad AK (2018) A novel ranking approach to solving fully LR-intuitionistic fuzzy transportation problems. New Math Nat Comput 15(01):95–112. https://doi.org/10.1142/s1793005719500066
Article MathSciNet Google Scholar
Aggarwal S, Gupta C (2017) Sensitive analysis in intuitionistic fuzzy solid transportation problem. Int J Fuzzy Syst. https://doi.org/10.1007/s40815-016-0292-8
Article Google Scholar
Angelov PP (1997) Optimization in an intuitionistic fuzzy environment. Fuzzy Sets Syst 86(3):299–306. https://doi.org/10.1016/S0165-0114(96)00009-7
Article MATH MathSciNet Google Scholar
Anuradha D (2015) On solving fuzzy solid assignment problems. Int Res J Eng Technol 2(5):322–325
Google Scholar
Anuradha D, Pandian P (2012) A new method for finding an optimal solution to solid assignment problems. Int J Eng Res Appl 2(3):1614–1618
Atanassov K (1983) Intuitionistic fuzzy sets, VII ITKR’s Session, Sofia, June 1983 (Deposed in Central Sci.—Techn. Library of Bulg. Acad. of Sci., 1697/84) (in Bulgarian)
Atanassov KT (1999) Intuitionistic fuzzy sets: theory and applications. Physica-Verlag, Heidelberg
Book Google Scholar
Burillo P, Bustince H, Mohedano V (1994) Some definitions of intuitionistic fuzzy number-first properties. In: Proceedings of the first workshop on fuzzy based expert system, Sofia, Bulgaria, pp 53–55
Dinagar DS, Palanivel K (2009) The transportation problem in fuzzy environment. Int J Algorithms Comput Math 2(3):65–71
MATH Google Scholar
Faudzi S, Abdul-Rahman S, Abd Rahman R (2018) An assignment problem and its application in education domain: a review and potential path. Adv Oper Res 2018:Article ID 8958393. https://doi.org/10.1155/2018/8958393
Gotmare AD, Khot PG (2016) Solution of a fuzzy assignment problem by using Branch-and-Bound technique with application of linguistic variable. Int J Comput Technol 15(4):6649–6653
Habib M (2018) Handbook of research on investigations in artificial life research and development. IGI Global, Hershey, pp 1–501. https://doi.org/10.4018/978-1-5225-5396-0
Hossen MA, Akther A (2017) Divide row minima and subtract column minima technique for solving assignment problems. Int J Adv Res 5(12):1323–1329. https://doi.org/10.21474/IJAR01/6089
Hu K-J (2017) Fuzzy goal programming technique for solving flexible assignment problem in PCB assembly line. J Inf Optim Sci 38(3–4):423–442. https://doi.org/10.1080/02522667.2016.1187922
Hussain RJ, Kumar PS (2012a) Algorithmic approach for solving intuitionistic fuzzy transportation problem. Appl Math Sci 6:3981–3989
MATH MathSciNet Google Scholar
Hussain RJ, Kumar PS (2012b) The transportation problem in an intuitionistic fuzzy environment. Int J Math Res 4:411–420
Hussain RJ, Kumar PS (2012c) The transportation problem with the aid of triangular intuitionistic fuzzy numbers. In: Proceedings in international conference on mathematical modeling and applied soft computing (MMASC-2012), vol 1. Coimbatore Institute of Technology, pp 819–825
Hussain RJ, Kumar PS (2013) An optimal more-for-less solution of mixed constraints intuitionistic fuzzy transportation problems. Int J Contemp Math Sci 8:565–576. https://doi.org/10.12988/ijcms.2013.13056
Jinshuai Y, Jin L, Yi W, Tong W, Zhanqiang L (2017) Optimization of weapon-target assignment problem by intuitionistic fuzzy genetic algorithm. In: MATEC Web of conferences, vol 128, p 02004. EDP Sciences. https://doi.org/10.1051/matecconf/201712802004
Kadhem D (2017) Heuristic solution approaches to the solid assignment problem (Doctoral dissertation, University of Essex)
Khalifa HA, Al-Shabi M (2018) An interactive approach for solving fuzzy multi-objective assignment problems. J Adv Math Comput Sci 28(6):1–12. https://doi.org/10.9734/JAMCS/2018/44339
Kuhn HW (1955) The Hungarian method for the assignment problem. Naval Res Logist Q 2(1–2):83–97. https://doi.org/10.1002/nav.3800020109
Kumar PS (2016a) PSK method for solving type-1 and type-3 fuzzy transportation problems. Int J Fuzzy Syst Appl 5(4):121–146. https://doi.org/10.4018/IJFSA.2016100106
Kumar PS (2016b) A simple method for solving type-2 and type-4 fuzzy transportation problems. Int J Fuzzy Logic Intell Syst 16(4):225–237. https://doi.org/10.5391/IJFIS.2016.16.4.225
Kumar PS (2017a) PSK method for solving type-1 and type-3 fuzzy transportation problems. In: Fuzzy systems: concepts, methodologies, tools, and applications. IGI Global, Hershey, pp 367–392. https://doi.org/10.4018/978-1-5225-1908-9.ch017
Kumar PS (2017b) Algorithmic approach for solving allocation problems under intuitionistic fuzzy environment, PhD thesis, Jamal Mohamed College, affiliated to the Bharathidasan University, Tiruchirappalli, India. http://hdl.handle.net/10603/209151
Kumar PS (2018a) Search for an optimal solution to vague traffic problems using the PSK method. In: Habib M (ed) Handbook of research on investigations in artificial life research and development. IGI Global, Hershey, pp 219–257. https://doi.org/10.4018/978-1-5225-5396-0.ch011
Chapter Google Scholar
Kumar PS (2018b) A note on ‘a new approach for solving intuitionistic fuzzy transportation problem of type-2’. Int J Logist Syst Manag 29(1):102–129. https://econpapers.repec.org/article/idsijlsma/v_3a29_3ay_3a2018_3ai_3a1_3ap_3a102-129.htm
Kumar PS (2018c) Linear programming approach for solving balanced and unbalanced intuitionistic fuzzy transportation problems. Int J Oper Res Inf Syst 9(2):73–100. https://doi.org/10.4018/IJORIS.2018040104
Kumar PS (2018d) A simple and efficient algorithm for solving type-1 intuitionistic fuzzy solid transportation problems. Int J Oper Res Inf Syst 9(3):90–122. https://doi.org/10.4018/IJORIS.2018070105
Kumar PS (2018e) PSK method for solving intuitionistic fuzzy solid transportation problems. Int J Fuzzy Syst Appl 7(4):62–99. https://doi.org/10.4018/ijfsa.2018100104
Kumar PS (2019a) Integer programming approach for solving solid and intuitionistic fuzzy solid assignment problems. Int J Syst Assur Eng Manag (under review)
Kumar PS (2019b) Algorithms for solving the optimization problems using fuzzy and intuitionistic fuzzy set. Int J Syst Assur Eng Manag (Under review, minor revision)
Kumar PS (2019c) PSK method for solving mixed and type-4 intuitionistic fuzzy solid transportation problems. Int J Oper Res Inf Syst 10(2):20–53. https://doi.org/10.4018/IJORIS.2019040102
Kumar PS (2019d) Software-based approach for solving intuitionistic fuzzy solid transportation problems. Int J Appl Manag Sci (under review)
Kumar PS (2019e) Discovery of a new kind of type-2 fuzzy and type-2 intuitionistic fuzzy transportation problems and identification of its optimal solutions. Int J Oper Res Inf Syst (under review)
Kumar PS (2019f) Linear programming method for solving solid and intuitionistic fuzzy solid transportation problems. Int J Syst Assur Eng Manag (under review)
Kumar PS (2019g) Intuitionistic fuzzy zero point method for solving type-2 intuitionistic fuzzy transportation problem. Int J Oper Res (article in press)
Kumar PS (2019h) Using the PSK method discovery of a solution to uncertain transportation problems and determination of the solution to the special case of type-2 fuzzy transportation problems. Complex & Intelligent Systems (under review)
Kumar PS (2020a) The PSK method for solving fully intuitionistic fuzzy assignment problems with some software tools. Theoretical and applied mathematics in international business. IGI Global, Hershey (accepted)
Kumar PS (2020b) Computationally simple and efficient method for solving real-life mixed intuitionistic fuzzy solid assignment problems. Int J Fuzzy Syst Appl (accepted)
Kumar PS (2020c) Developing a new approach to solve solid assignment problems under intuitionistic fuzzy environment. Int Journal of Fuzzy Syst Appl 9(1),Article 1 (accepted)
Kumar PS, Hussain RJ (2014a) A systematic approach for solving mixed intuitionistic fuzzy transportation problems. Int J Pure Appl Math 92:181–190. https://doi.org/10.12732/ijpam.v92i2.4
Article MATH Google Scholar
Kumar PS, Hussain RJ (2014b) A method for finding an optimal solution of an assignment problem under mixed intuitionistic fuzzy environment. In: Proceedings in international conference on mathematical sciences (ICMS-2014). Elsevier, Sathyabama University, pp 417–421, ISBN-978-93-5107-261-4
Kumar PS, Hussain RJ (2014c) New algorithm for solving mixed intuitionistic fuzzy assignment problem. Elixir Appl Math 73:25971–25977
Kumar PS, Hussain RJ (2014d) A method for solving balanced intuitionistic fuzzy assignment problem. Int J Eng Res Appl 4(3):897–903
Kumar PS, Hussain RJ (2015) A method for solving unbalanced intuitionistic fuzzy transportation problems. Notes Inst Fuzzy Sets 21(3):54–65
Kumar PS, Hussain RJ (2016a) Computationally simple approach for solving fully intuitionistic fuzzy real life transportation problems. Int J Syst Assur Eng Manag 7(1):90–101. https://EconPapers.repec.org/RePEc:spr:ijsaem:v:7:y:2016:i:1:d:10.1007_s13198-014-0334-2 . https://doi.org/10.1007/s13198-014-0334-2
Kumar PS, Hussain RJ (2016b) A simple method for solving fully intuitionistic fuzzy real life assignment problem. Int J Oper Res Inf Syst 7(2):39–61. https://EconPapers.repec.org/RePEc:igg:joris0:v:7:y:2016:i:2:p:39-61 https://doi.org/10.4018/IJORIS.2016040103
Kumar PS, Hussain RJ (2016c) An algorithm for solving unbalanced intuitionistic fuzzy assignment problem using triangular intuitionistic fuzzy number. J Fuzzy Math 24(2):289–302
Kumar N, Shukla D (2016) Resource management through fuzzy assignment problem in cloud computing environment. In: Proceedings of the second international conference on information and communication technology for competitive strategies. ACM, p 87. https://doi.org/10.1145/2905055.2905146
Malini P, Ananthanarayanan M (2016) Solving fuzzy assignment problem using ranking of generalized trapezoidal fuzzy numbers. Indian J Sci Technol 9(20):1–4. https://doi.org/10.17485/ijst/2016/v9i20/88691
Mohideen SI, Kumar PS (2010) A comparative study on transportation problem in fuzzy environment. Int J Math Res 2:151–158
Muralidaran C, Venkateswarlu B (2017) A method for solving equally spread symmetric fuzzy assignment problems using branch and bound technique (14th ICSET-2017). IOP Conf Ser Mater Sci Eng 263(4):042123. https://doi.org/10.1088/1757-899x/263/4/042123
Nagoorgani A, Kavikumar J, Mohamed VN, Shamsidah A. N (2016) A labeling algorithm for solving intuitionistic fuzzy optimal assignment problems. In: 2016 IEEE international conference on fuzzy systems (FUZZ-IEEE). IEEE, Vancouver, BC, Canada, pp 1621–1627). https://doi.org/10.1109/fuzz-ieee.2016.7737884
Ozor PA, Chigozirim PO, Odukwe AO, Ume JI, Ozoegwu CG (2017) Application of the assignment technique to optimisation of solid waste management in Enugu region. Int J Environ Waste Manag 19(1):52–73
Pierskalla WP (1967) The tri-substitution method for the three-dimensional assignment problem. CORS J 5:71–81
Saini RK, Sangal A, Prakash O (2018) Fuzzy transportation problem with generalized triangular-trapezoidal fuzzy number. In: Soft computing: theories and applications. Springer, Singapore, pp 723–734. https://doi.org/10.1007/978-981-10-5687-1_64
Sam’an M, Surarso B, Zaki S (2018) A modified algorithm for full fuzzy transportation problem with simple additive weighting. In: 2018 International conference on information and communications technology (ICOIACT). IEEE, pp 684–688. https://doi.org/10.1109/icoiact.2018.8350745
Thakre TA, Chaudhari OK, Dhawade NR (2018) Placement of staff in LIC using fuzzy assignment problem. Int J Math Trends Technol 53(4):259–266
Varghese A, Kuriakose S (2012) Centroid of an intuitionistic fuzzy number. Notes Inst Fuzzy Sets 18:19–24
Vinoliah EM, Ganesan K (2018) Fuzzy optimal solution for a fuzzy assignment problem with octagonal fuzzy numbers. J Phys Conf Ser 1000(1):012016
Zadeh LA (1965) Fuzzy sets. Inf Control 8:338–353
Download references
Acknowledgements
The author is grateful to anonymous referees for their constructive as well as helpful suggestions and comments to revise the paper in the present form.
Author information
Authors and affiliations.
Department of Humanities and Sciences, Navodaya Institute of Technology, Bijanagera Road, P.B. No. 26, Navodaya Nagar, Raichur, Karnataka, 584103, India
P. Senthil Kumar
You can also search for this author in PubMed Google Scholar
Corresponding author
Correspondence to P. Senthil Kumar .
Additional information
Publisher's note.
Springer Nature remains neutral with regard to jurisdictional claims in published maps and institutional affiliations.
Rights and permissions
Reprints and permissions
About this article
Kumar, P.S. Intuitionistic fuzzy solid assignment problems: a software-based approach. Int J Syst Assur Eng Manag 10 , 661–675 (2019). https://doi.org/10.1007/s13198-019-00794-w
Download citation
Received : 24 February 2018
Revised : 17 April 2019
Published : 23 May 2019
Issue Date : August 2019
DOI : https://doi.org/10.1007/s13198-019-00794-w
Share this article
Anyone you share the following link with will be able to read this content:
Sorry, a shareable link is not currently available for this article.
Provided by the Springer Nature SharedIt content-sharing initiative
- Intuitionistic fuzzy set
- Intuitionistic fuzzy number
- Triangular intuitionistic fuzzy number
- Assignment problem
- Solid assignment problem
- Intuitionistic fuzzy solid assignment problem
- Software-based approach
- Optimal assignment
- Optimal solution
- Find a journal
- Publish with us
- Track your research
- Corpus ID: 123806572
A Method for Solving Balanced Intuitionistic Fuzzy Assignment Problem
- P. Senthil Kumar , R. J. Hussain
- Published 2014
- Mathematics, Computer Science
19 Citations
New algorithm for solving mixed intuitionistic fuzzy assignment problem, an algorithm for solving unbalanced intuitionistic fuzzy assignment problem using triangular intuitionistic fuzzy number, a simple method for solving fully intuitionistic fuzzy real life assignment problem, optimization of fuzzy k-objective fractional assignment problem using fuzzy programming model, the psk method for solving fully intuitionistic fuzzy assignment problems with some software tools, intuitionistic fuzzy solid assignment problems: a software-based approach, algorithms for solving the optimization problems using fuzzy and intuitionistic fuzzy set, psk method for solving intuitionistic fuzzy solid transportation problems, a simple and efficient algorithm for solving type-1 intuitionistic fuzzy solid transportation problems, an integer solution in intuitionistic transportation problem with application in agriculture, 21 references, a general approach for solving assignment problems involving with fuzzy cost coefficients, fuzzy assignment problem with generalized fuzzy numbers, a labeling algorithm for the fuzzy assignment problem, an optimal more-for-less solution of mixed constraints intuitionistic fuzzy transportation problems, assignment and travelling salesman problems with coefficients as lr fuzzy parameters.
- Highly Influential
An analysis of a decomposition heuristic for the assignment problem
The alternating basis algorithm for assignment problems, solving the assignment problem by relaxation, the optimal assignment problem., related papers.
Showing 1 through 3 of 0 Related Papers
Information
- Author Services

Initiatives
You are accessing a machine-readable page. In order to be human-readable, please install an RSS reader.
All articles published by MDPI are made immediately available worldwide under an open access license. No special permission is required to reuse all or part of the article published by MDPI, including figures and tables. For articles published under an open access Creative Common CC BY license, any part of the article may be reused without permission provided that the original article is clearly cited. For more information, please refer to https://www.mdpi.com/openaccess .
Feature papers represent the most advanced research with significant potential for high impact in the field. A Feature Paper should be a substantial original Article that involves several techniques or approaches, provides an outlook for future research directions and describes possible research applications.
Feature papers are submitted upon individual invitation or recommendation by the scientific editors and must receive positive feedback from the reviewers.
Editor’s Choice articles are based on recommendations by the scientific editors of MDPI journals from around the world. Editors select a small number of articles recently published in the journal that they believe will be particularly interesting to readers, or important in the respective research area. The aim is to provide a snapshot of some of the most exciting work published in the various research areas of the journal.
Original Submission Date Received: .
- Active Journals
- Find a Journal
- Proceedings Series
- For Authors
- For Reviewers
- For Editors
- For Librarians
- For Publishers
- For Societies
- For Conference Organizers
- Open Access Policy
- Institutional Open Access Program
- Special Issues Guidelines
- Editorial Process
- Research and Publication Ethics
- Article Processing Charges
- Testimonials
- Preprints.org
- SciProfiles
- Encyclopedia
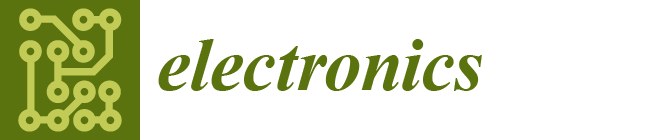
Article Menu
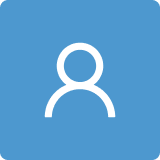
- Subscribe SciFeed
- Recommended Articles
- Google Scholar
- on Google Scholar
- Table of Contents
Find support for a specific problem in the support section of our website.
Please let us know what you think of our products and services.
Visit our dedicated information section to learn more about MDPI.
JSmol Viewer
Aggregators used in fuzzy control—a review.
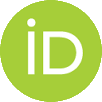
1. Introduction
- Provide an accurate and detailed summary of the current state of re-examination of aggregation functions;
- Cover theoretical advances, practical applications, and empirical research in one comprehensive document;
- Highlight the importance of this research and its results for a better understanding and application of aggregation functions;
- Synthesise findings from different studies to identify common themes, patterns, and trends;
- Integrate insights from different research areas to provide a broad perspective and holistic view of the field.
- Section 2 (Preliminaries)—basic assumptions and definitions to remind and facilitate the concept of aggregation and others related to this paper;
- Section 3 (Materials and Methods)—an approach to article search and selection;
- Section 4 (Results)—a synthetic summary of the selected publications and their collation;
- Section 5 (Discussion)—analysis and review of the results;
- Section 6 (Conclusions)—presents conclusions and a summary of the scope of the selected articles.
2. Preliminaries
2.1. basic definitions.
- f(a, a, a, …, a) = a and f(b, b, b, …, b) = b;-*
- for x, y argument values x ≤ y implies f (x) ≤ f (y) for all x, y ∈ I n .
2.2. Classification and General Properties
2.2.1. main classes.
- Conjunctive;
- Disjunctive;
2.2.2. Main Properties
2.2.3. comparability, 2.2.4. continuity and stability, 2.3. main families, 2.3.1. min and max, 2.3.2. means, 2.3.3. medians, 2.3.4. choquet and sugeno integrals, 2.4. selection of aggregation function.
- Select an aggregation function that must match the semantics of the aggregation procedure;
- Choose the right member of the class/family that does what it is supposed to do—produce the right output for the input.
- the least-squares norm ( p = 2)
- the latest absolute deviation norm ( p = 1):
- the Chebyshev norm ( p = ∞):
- or their weighted analogues, like:
3. Materials and Methods
3.1. dataset and devices, 3.2. methods, 4.1. scope of topics in the publications, 4.2. early attempts, 4.3. late attempts.
- In the first stage, the optimal charging of each PEV is calculated using the Bee algorithm as the aggregator optimizer;
- In the second stage, the aggregated power is distributed among electric vehicles using a fuzzy controller.
5. Discussion
5.1. limitations of current approaches to aggregators.
- Selection of aggregator functions: its subjectivity and lack of standardization—there is no universally accepted standard for the selection of aggregation operators, which may lead to inconsistencies between different systems and applications;
- Computational complexity that limits performance and scalability, especially in real-time fuzzy control applications where fast response times are important, and as the number of input data increases, the computational burden of some aggregation methods may become too high;
- Potential loss of information: oversimplification, especially when using simple aggregation methods such as minimum or maximum operators, which may result in less accurate or less diverse resulting control;
- Nonlinearity and uncertainty: some aggregation methods may introduce nonlinearity into the system, making the behavior of a fuzzy control system more difficult to predict and analyze, as well as suboptimal performance in uncertain environments;
- Context dependence: lack of adaptability in dynamic environments where the relationships between inputs and outputs may change over time;
- Input interdependence: ignoring the interconnectedness of inputs can lead to incorrect control decisions;
- The complexity of designing and tuning aggregation operators can be high and time-consuming, especially when tuning for a specific application domain;
- Integration with other systems: aggregation methods in fuzzy control systems may not be easily integrated with other control or decision-making systems;
- Objectively assessing the performance of different aggregation operators can be difficult, placing high demands on comparison and selection of the most appropriate one for a particular application.
5.2. Directions of Further Studies on Aggregators
- Adaptive aggregation methods: developing aggregation operators that adapt to changing contexts or operating conditions in real time based on the current or predicted state of the system;
- Learning-based aggregation: implementing machine-learning techniques (neural networks, reinforcement learning) to learn optimal aggregation strategies from data to improve the adaptability and performance of fuzzy control systems;
- Complexity reduction: designing more computationally efficient aggregation algorithms that reduce the computational load, especially for real-time applications and systems with a large number of inputs, but also developing simplified aggregation models as a trade-off between computational efficiency and the accuracy of the aggregated result (sufficient aggregator instead of optimal aggregator);
- Creating aggregation operators resistant to uncertainty (e.g., based on the integration of concepts from the probabilistic and interval approaches);
- Development of nonlinear aggregation techniques that can better capture the complex relationships between inputs while maintaining computational feasibility;
- Correlation-aware aggregation: create multidimensional aggregation operators that consider common distributions of input data, providing a more accurate representation of their common behavior;
- Multi-criteria and multi-objective aggregation: development of multi-criteria decision-making (MCDM) techniques with fuzzy aggregation to handle scenarios with many conflicting goals or criteria—Pareto-optimal aggregation also applies here;
- Hybrid control systems: combining fuzzy aggregation methods with other control strategies;
- Development of aggregator performance metrics and benchmarking frameworks to objectively evaluate and compare different aggregation operators in terms of accuracy, robustness, computational efficiency, and adaptability in various practical applications.
6. Conclusions
Author contributions, data availability statement, conflicts of interest.
- Klir, G.J.; Folger, T.A. Fuzzy Sets, Uncertainty and Information. Prentice Hall: Englewood Cliffs, NJ, USA, 1988. [ Google Scholar ]
- Ventura Silva, L.; Barbosa, P.; Marinho, R.; Brito, A. Security and privacy awared at a aggregation on cloud computing. J. Internet Serv. Appl. 2018 , 9 , 6:1–6:13. [ Google Scholar ] [ CrossRef ]
- Zheng, Y.; Wang, D. Multi-Criteria Ranking: Next Generation of Multi-Criteria Recommendation Framework. IEEE Access 2022 , 10 , 90715–90725. [ Google Scholar ] [ CrossRef ]
- Chen, Z.-S.; Zhu, Z.; Wang, X.-J.; Chiclana, F.; Herrera-Viedma, E.; Skibniewski, M.J. Multiobjective Optimization-Based Collective Opinion Generation with Fairness Concern. IEEE Trans. Syst. ManCybern. Syst. 2023 , 53 , 5729–5741. [ Google Scholar ] [ CrossRef ]
- Amorim, M.; Dimuro, G.; Borges, E.; Dalmazo, B.L.; Marco-Detchart, C.; Lucca, G.; Bustince, H. Systematic Review of Aggregation Functions Applied to Image Edge Detection. Axioms 2023 , 12 , 330. [ Google Scholar ] [ CrossRef ]
- Sartori, J.; de Bem, R.; Pereira Dimuro, G.; Lucca, G. The Role of Aggregation Functions on Transformers and ViTs Self-Attention for Classification. In Proceedings of the 2023 36th SIBGRAPI Conference on Graphics, Patterns and Images (SIBGRAPI), Rio Grande, Brazil, 6–9 November 2023; pp. 97–102. [ Google Scholar ]
- Prokopowicz, P.; Ślęzak, D. Ordered Fuzzy Numbers: Definitions and Operations. In Theory and Applications of Ordered Fuzzy Numbers ; Studies in Fuzziness and Soft, Computing; Prokopowicz, P., Czerniak, J., Mikołajewski, D., Apiecionek, Ł., Ślęzak, D., Eds.; Springer: Cham, Switzerland, 2017; Volume 356. [ Google Scholar ] [ CrossRef ]
- Prokopowicz, P.; Golsefid, S.M.M. Aggregation Operator for Ordered Fuzzy Numbers Concerning the Direction. In Artificial Intelligence and Soft Computing. ICAISC 2014 ; Rutkowski, L., Korytkowski, M., Scherer, R., Tadeusiewicz, R., Zadeh, L.A., Zurada, J.M., Eds.; Lecture Notes in Computer Science; Springer: Cham, Switzerland, 2014; Volume 8467. [ Google Scholar ] [ CrossRef ]
- Senapati, T.; Chen, G.; Mesiar, R.; Yager, R.R. Intuitionistic Fuzzy Geometric Aggregation Operators in the Framework of Aczel-Alsina Triangular Norms and Their Application to Multiple Attribute Decision Making. Expert Syst. Appl. 2023 , 212 , 118832. [ Google Scholar ] [ CrossRef ]
- Seliga, A.; Hriňáková, K.; Seligova, I. Positively Homogeneous and Super-/Sub- Additive Aggregation Functions. Fuzzy Sets Syst. 2022 , 451 , 385–397. [ Google Scholar ] [ CrossRef ]
- Karczmarek, P.; Dolecki, M.; Powroźnik, P.; Lagodowski, Z.; Gregosiewicz, A.; Gałka, Ł.; Pedrycz, W.; Czerwiński, D.; Jonak, K. Quadrature-Inspired Generalized Choquet Integral in an Application to Classification Problems. IEEE Access 2023 , 11 , 124676–124689. [ Google Scholar ] [ CrossRef ]
- Beliakov, G.; Bustince Sola, H.; Calvo Sanchez, T. A Practical Guide to Averaging Functions. In Studies in Fuzziness and Soft Computing ; Springer: NewYork, NY, USA, 2016; Volume 329. [ Google Scholar ] [ CrossRef ]
- Prokopowicz, P. Processing Direction with Ordered Fuzzy Numbers. In Theory and Applications of Ordered Fuzzy Numbers ; Studies in Fuzziness and Soft, Computing; Prokopowicz, P., Czerniak, J., Mikołajewski, D., Apiecionek, Ł., Ślęzak, D., Eds.; Springer: Cham, Switzerland, 2017; Volume 356. [ Google Scholar ] [ CrossRef ]
- Zimmermann, H.-J. Fuzzy Set Theory—And Its Applications ; Kluwer: Boston, MA, USA, 1996. [ Google Scholar ]
- Huber, P.J. Robust Statistics ; Wiley: New York, NY, USA, 2003. [ Google Scholar ]
- Maronna, R.; Martin, R.; Yohai, V. Robust Statistics: Theory and Methods. Wiley: New York, NY, USA, 2006. [ Google Scholar ]
- Rousseeuw, P.J.; Leroy, A.M. Robust Regression and Outlier Detection ; Wiley: NewYork, NY, USA, 2003. [ Google Scholar ]
- Yager, R.R.; Beliakov, G. OWA operators in regression problems. IEEE Trans. Fuzzy Syst. 2010 , 18 , 106–113. [ Google Scholar ] [ CrossRef ]
- Beliakov, G.; James, S.; Li, G. Learning Choquet-integral based metrics for semisupervised clustering. IEEE Trans. Fuzzy Syst. 2011 , 19 , 562–574. [ Google Scholar ] [ CrossRef ]
- Beliakov, G.; Calvo, T.; James, S. Aggregation of preferences in recommender systems. In Recommender Systems Handbook ; Kantor, P.B., Ricci, F., Rokach, L., Shapira, B., Eds.; Springer: Berlin/Heidelberg, Germany, 2010. [ Google Scholar ]
- Beliakov, G.; James, S. Citation-based journal ranks: The use of fuzzy measures. Fuzzy Sets Syst. 2011 , 167 , 101–119. [ Google Scholar ] [ CrossRef ]
- Kaymak, U.; van Nauta Lemke, H.R. Selecting an aggregation operator for fuzzy decision making. In Proceedings of the 1994 IEEE 3rd International Fuzzy Systems Conference, Orlando, FL, USA, 26–29 June 1994; pp. 1418–1422. [ Google Scholar ]
- Ravi, V.; Reddy, P.J.; Dutta, D. Application of fuzzy nonlinear goal programming to a refinery model. Comput. Chem. Eng. 1998 , 22 , 709–712. [ Google Scholar ] [ CrossRef ]
- Kumar, R.; Stover, J.A. The CINET fuzzy classifier: Formal background and enhancements. In Proceedings of the 1999 IEEE International Symposium on Intelligent Control Intelligent Systems and Semiotics (Cat. No.99CH37014), Cambridge, MA, USA, 17 September 1999; pp. 314–319. [ Google Scholar ] [ CrossRef ]
- Fernández Salido, J.M.; Murakami, S. On β-Precision aggregation. Fuzzy Sets Syst. 2003 , 139 , 547–558. [ Google Scholar ] [ CrossRef ]
- De Cristofaro, E.; Bohli, J.-M.; Westhoff, D. FAIR: Fuzzy-based aggregation providing in-network resilience forreal-time wireless sensor networks. In Proceedings of the WiSec‘09: Proceedings of the Second ACM Conference on Wireless Network Security, Zurich, Switzerland, 16–18 March 2009; pp. 253–260. [ Google Scholar ] [ CrossRef ]
- Han, S.; Han, S.; Sezaki, K. Development of an Optimal Vehicle-to-Grid Aggregator for Frequency Regulation. IEEE Trans. Smart Grid 2010 , 1 , 65–72. [ Google Scholar ] [ CrossRef ]
- Kasi, V.R.; Thirugnanam, K.; Kumar, P.; Majhi, S. Node identification for placing EVs and Pas in a distribution network. In Proceedings of the 2014 IEEE PES General Meeting|Conference& Exposition, National Harbor, MD, USA, 27–31 July 2014; pp. 1–5. [ Google Scholar ] [ CrossRef ]
- Fattahi Bandpey, M.; Gorgani Firouzjah, K. Two-stage charging strategy of plug-in electric vehicles based on fuzzy control. Comput. Oper. Res. 2018 , 96 , 236–243. [ Google Scholar ] [ CrossRef ]
- Song, D.; Shao, Y.; Zou, S.; Zhao, X.; Li, S.; Ma, Z. Fuzzy-Logic-Based Adaptive Internal Model Control for Load FrequencyControl Systems with Electric Vehicles. In Proceedings of the 39th Chinese Control Conference (CCC), Shenyang, China, 27–29 July 2020; pp. 1987–1993. [ Google Scholar ] [ CrossRef ]
- Oshnoei, A.; Kheradmandi, M.; Muyeen, S.M. Robust Control Scheme for Distributed Battery Energy Storage Systems in Load Frequency Control. IEEE Trans. Power Syst. 2020 , 35 , 4781–4791. [ Google Scholar ] [ CrossRef ]
- Mesiar, R.; Kolesarova, A. On the fuzzy set theory and aggregation functions: History and some recent advances. Iran. J. Fuzzy Syst. 2018 , 15 , 1–12. [ Google Scholar ]
- Wątróbski, J.; Jankowski, J.; Ziemba, P.; Karczmarczyk, A.; Zioło, M. Generalised framework for multi-criteria method selection. Omega 2019 , 86 , 107–124. [ Google Scholar ] [ CrossRef ]
- Faizi, S.; Rashid, T.; Sałabun, W.; Zafar, S.; Wątróbski, J. Decision making with uncertainty using hesitant fuzzy sets. Int. J. Fuzzy Syst. 2018 , 20 , 93–103. [ Google Scholar ] [ CrossRef ]
- Li, Y.; Dong, J. Fault Detection for Discrete-Time Interval Type-2 Takagi–Sugeno Fuzzy Systems Using H−/L∞ Unknown Input Observer and Zonotopic Analysis. IEEE Trans. Fuzzy Syst. 2024 , 32 , 846–858. [ Google Scholar ] [ CrossRef ]
Click here to enlarge figure
Family | Name | Feature | Application |
---|---|---|---|
Minimum (AND) and maximum (OR) functions | Minimum | Selects the smallest value from a set of membership degrees | The most commonly used conjunction (AND) function. |
Maximum | Selects the biggest value from a set of membership degrees | The most commonly used disjunction (OR) function. | |
Summation functions | Summation | Degrees of membership are added together, which can lead to values greater than 1. | Basic approach |
Probabilistic sum | Limits the result to the interval [0,1]. | More sophisticated approach than basic summation | |
Algebraic functions | Algebraic product | Multiplies degrees of membership | Works well for simple models with a small number of inputs. |
Constrained sum | Ensures that the result does not exceed 1 | System model with saturation or cut-off. | |
Weighted functions | Weighted average | Assigns different weights to different degrees of membership and then calculates their weighted average | When heavier weighted inputs have a greater impact on the average. |
Weighted sum | Similar to the sum method, but each value is multiplied by its weight before addition | When inputs have more weight they have a greater impact on their total.The weighted sum method is one of the best-known algorithms for multi-criteria analysis. | |
Specialised functions | T-norms (Triangular norms) | In addition to algebraic product and minimum, they also include other t-norms, such as the Lukasiewicz t-norm | Used to model conjunction in fuzzy systems |
S-norms (Triangular conorms) | In addition to the maximum and probabilistic sum, they also include other s-norms, such as the Lukasiewicz s-norm | Used to model alternatives in fuzzy systems |
Area | Application | Description |
---|---|---|
Control systems | Industrial process control | Aggregation functions are used to combine different control variables to ensure stability and optimum performance of control systems, such as temperature, pressure, or flow control. |
Robot control | Aggregation functions combine data from different sensors, such as cameras or tactile sensors, to enable robots to make complex decisions in real time. | |
Expert systems and decision-making systems | Financial systems | Aggregation functions are used to analyse risk, combine different financial indicators, and predict market trends. |
Medical diagnosis | Aggregation functions combine different symptoms and test results to help diagnose diseases. | |
Recommendation systems | Product recommendations | Aggregation functions combine different product features and user preferences to generate personalised recommendations. |
Content recommendations | Aggregation functions combine data on viewed content, ratings, and user preferences to recommend e.g., new books, games, films, etc. | |
Intelligent transport systems, fleet management, and logistic chain management | Navigation and route planning | Aggregation functions combine data from different sources such as traffic, weather conditions, and user preferences to optimise routes and minimise travel time. |
Fleet management | Aggregation functions combine information on vehicle condition, routes, and schedules to optimise management and maintenance. | |
Resource and energy management | Energy management systems | Aggregation functions combine data on energy consumption, weather forecasts, and the availability of renewable energy sources to optimise energy management and distribution. |
Smart grids | Aggregation functions combine data from different metering points to manage energy flows and ensure grid stability. | |
Pattern recognition and classification systems | Image-recognitionsystems | Aggregation functions are used to combine the results of different image-processing algorithms to improve the accuracy of object recognition. |
Data-analysis systems | Aggregation functions combine different metrics and measures to classify and interpret large datasets. | |
Security and monitoring | Security systems | Aggregation functions combine data from various sensors such as cameras, motion sensors, and alarm systems to detect threats and unauthorised entry. |
Environmental monitoring | Aggregation functions combine data from air, water and soil quality sensors to monitor the state of the environment and detect pollution. | |
Agricultural decision support systems | Farm Management | Aggregation functions are used to combine weather, soil, plant, and resource-management data to help farmers make planting, irrigation, and fertilisation decisions. |
Plant-health monitoring | Aggregation functions combine data from sensors, satellite imagery, and drones to monitor plant health and detect potential problems. |
The statements, opinions and data contained in all publications are solely those of the individual author(s) and contributor(s) and not of MDPI and/or the editor(s). MDPI and/or the editor(s) disclaim responsibility for any injury to people or property resulting from any ideas, methods, instructions or products referred to in the content. |
Share and Cite
Kozielski, M.; Prokopowicz, P.; Mikołajewski, D. Aggregators Used in Fuzzy Control—A Review. Electronics 2024 , 13 , 3251. https://doi.org/10.3390/electronics13163251
Kozielski M, Prokopowicz P, Mikołajewski D. Aggregators Used in Fuzzy Control—A Review. Electronics . 2024; 13(16):3251. https://doi.org/10.3390/electronics13163251
Kozielski, Mirosław, Piotr Prokopowicz, and Dariusz Mikołajewski. 2024. "Aggregators Used in Fuzzy Control—A Review" Electronics 13, no. 16: 3251. https://doi.org/10.3390/electronics13163251
Article Metrics
Further information, mdpi initiatives, follow mdpi.
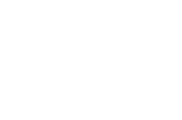
Subscribe to receive issue release notifications and newsletters from MDPI journals
Academia.edu no longer supports Internet Explorer.
To browse Academia.edu and the wider internet faster and more securely, please take a few seconds to upgrade your browser .
Enter the email address you signed up with and we'll email you a reset link.
- We're Hiring!
- Help Center
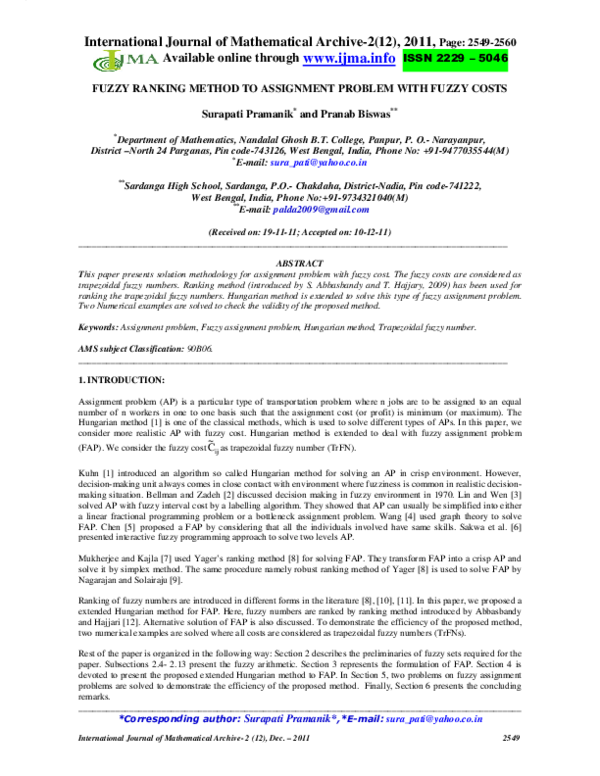
• Fuzzy Ranking Method to Assignment Problem with Fuzzy Costs

2012, International Journal of Mathematical Archive ( …
This paper presents solution methodology for assignment problem with fuzzy cost. The fuzzy costs are considered as trapezoidal fuzzy numbers. Ranking method (introduced by S. Abbasbandy and T. Hajjary, 2009) has been used for ranking the trapezoidal fuzzy ...
Related Papers
Journal of science - Misurata
Mohamed muamer
In this paper, we deal with solving a fuzzy Assignment Problem (FAP), in this problem C denotes the cost for assigning the n jobs to the n workers and C has been considered to be triangular fuzzy numbers. The Hungarian method is used for solving FAP by using ranking function for fuzzy costs. A numerical example is considered by incorporating a fuzzy numbers into the costs.
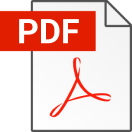
Supriya Kar
To solve the problems of Engineering and Management Science Generalized Assignment Problem (GAP) plays a very important role. The GAP is a classical example of a difficult combinatorial optimization problem that has received considerable attention over the years due to its widespread applications. In many instances it appears as a substructure in more complicated models, including routing problems, facility location models, knapsack problems, computer networking applications etc. Recently, Fuzzy Generalized Assignment Problem (FGAP) became very popular because in real life, data may not be known with certainty. So, to consider uncertainty in real life situations fuzzy data instead of crisp data is more advantageous. In this paper, cost for assigning the j-th job to the i-th person is taken as triangular fuzzy numbers. Further we have put a restriction on the total available cost which makes the problem more realistic and general. The problem is solved by modified Fuzzy Extremum Diff...
anchal choudhary
I n the literature, there are various methods to solve assignment problems in which parameters are represented by triangular or trapezoidal fuzzy numbers. This paper presents an assignment problem with fuzzy costs, where the objective is to minimize the cost. Here each fuzzy cost is assumed as trapezoidal fuzzy number. A new ranking method has been used for ranking the fuzzy trapezoidal numbers. The fuzzy assignment problem has been transformed into a crisp one using ranking function and solved by branch and bound method. A numerical example is provided to demonstrate the proposed approach.
K. Ruth Isabels
This paper presents an assignment problem with fuzzy costs, where the objective is to minimize the cost. Here each fuzzy cost is assumed as triangular or trapezoidal fuzzy number. Yager’s ranking method has been used for ranking the fuzzy numbers. The fuzzy assignment problem has been transformed into a crisp one, using linguistic variables and solved by Hungarian technique. The use of linguistic variables helps to convert qualitative data into quantitative data which will be effective in dealing with fuzzy assignment problems of qualitative nature. A numerical example is provided to demonstrate the proposed approach.
IAEME PUBLICATION
IAEME Publication
The Fuzzy Assignment Problem (FAP) is a classic combinatorial optimization problem that has received a lot of attention. FAP has a wide range of uses. We suggest a new algorithm that combines to solve the FAP in this paper. Each column is maximized during the optimization process, and the best choice with the lowest cost is selected. The proposed method follows a standard methodology, is simple to execute, and takes less effort to compute. An order to obtain the best solution, the assignment problem is specifically solved here. We looked at how well trapezoidal fuzzy numbers performed. Then, to convert crisp numbers, we use the robust ranking method for trapezoidal fuzzy numbers. The optimality of the result provided by this new method is clarified by a numerical example.
Journal of the Operational Research Society
iaeme iaeme
Bulletin of Electrical Engineering and Informatics
The assignment problem is a famous problem in combinatorial optimization where several objects (tasks) are assigned to different entities (workers) with the goal of minimizing the total assignment cost. In real life, this problem often arises in many practical applications with uncertain data. Hence, this data (the assignment cost) is usually presented as fuzzy numbers. In this paper, the assignment problem is considered with trapezoidal fuzzy parameters and solved using the novel Dhouib-Matrix-AP1 (DM-AP1) heuristic. In fact, this research work presents the first application of the DM-AP1 heuristic to the fuzzy assignment problem, and a step-by-step application of DM-AP1 is detailed for more clarity. DM-AP1 is composed of three simple steps and repeated only once in n iterations. Moreover, DM-AP1 is enhanced with two techniques: a ranking function to order the trapezoidal fuzzy numbers and the min descriptive statistical metric to navigate through the research space. DM-AP1 is developed under the Python programming language and generates a convivial assignment network diagram plan.
research.ijais.org
Surapati Pramanik
INTERNATIONAL JOURNAL OF COMPUTERS & TECHNOLOGY
Anil Gotmare
In this paper Branch and bound technique is applied to assignment problem with fuzzy cost with objective to minimise cost, fuzzy cost is assumed as triangular fuzzy number, Yager’s ranking method has been used for ranking the fuzzy numbers, after transforming assignment problem into a crisp one using linguistic variables, assignment problem is solved by branch and bound technique. To deal with fuzzy assignment problem with qualitative data, linguistic variable helps to convert qualitative data into quantitative data.
Loading Preview
Sorry, preview is currently unavailable. You can download the paper by clicking the button above.
RELATED PAPERS
SRMS Journal of Mathmetical Science
Anju Khandelwal
www.ijera.com
Dr. P. Senthil Kumar (PSK), M.Sc., B.Ed., M.Phil., PGDCA., PGDAOR., Ph.D.,
Soft Computing
Laxminarayan Sahoo
International Journal of Computer Applications
Prof. Ravi Shankar Nowpada
Atul Kumar Tiwari, Anunay Tiwari, Cherian Samuel & Satish Kumar Pandey
TJPRC Publication
International Journal of Computer …
Sophia Porchelvi
American Journal of Applied Sciences
Dimitris Askounis
International Journal of Operations Research and Information Systems (IJORIS)
Dr. P. Senthil Kumar (PSK), M.Sc., B.Ed., M.Phil., PGDCA., PGDAOR., Ph.D., , Jahir Hussain
Journal of Physics: Conference Series
Nur Insani, SH.MH Dhini
International Journal of Fuzzy System Applications (IJFSA)
George Rigopoulos
Applied Mathematical Modelling
International Journal of Computing Science and Mathematics
Thanh Thi Nguyen
- We're Hiring!
- Help Center
- Find new research papers in:
- Health Sciences
- Earth Sciences
- Cognitive Science
- Mathematics
- Computer Science
- Academia ©2024

IMAGES
COMMENTS
PDF | In this paper, we deal with solving a fuzzy Assignment Problem (FAP), in this problem C denotes the cost for assigning the n jobs to the n workers... | Find, read and cite all the research ...
Assignment problem is the biggest significant problem in decisionmaking. In this paper, a novel technique is planned to discover the best possible solution to a balanced fuzzy assignment problem ...
PDF | We solve a fully fuzzy assignment problem (FAP) where the costs are triangular fuzzy numbers. The FAP has gained its importance in the recent... | Find, read and cite all the research you ...
Step 1: Construct the intuitionistic fuzzy solid assign-ment problems. The intuitionistic fuzzy solid assignment problem is generally an assignment problem in which, the assignment time/costs/profits only are intuitionistic fuzzy numbers. Step 2: Now, calculate the ranking index (see Varghese and Kuriakose (2012) ranking procedure) for every ...
By the max-min criterion suggested by Bellman and Zadeh[3], the fuzzy assignment problem can be treated as a mixed integer nonlinear programming problem. Lin and Wen [13] investigated a fuzzy assignment problem in which the cost depends on the quality of the job.
The fuzzy assignment problem has been transformed into crisp assignment problem in the LPP form and solved by using LINGO 9.0. Numerical examples show that the fuzzy ranking method offers an effective way for handling the fuzzy assignment problem.
The fuzzy cost is measured as generalized fuzzy number. We developed the classical algorithm using fundamental theorems of fuzzy assignment problem to obtain minimum fuzzy cost and also for the variations in the fuzzy assignment problem. Proposed algorithms are illustrated with an example.
In this paper, some fuzzy assignment problems and fuzzy travelling salesman problems are chosen which cannot be solved by using the fore-mentioned method. Two new methods are proposed for solving such type of fuzzy assignment problems and fuzzy travelling salesman problems.
The fuzzy cost is measured as generalized fuzzy number. We developed the classical algorithm using fundamental theorems of fuzzy assignment problem to obtain minimum fuzzy cost and also for the variations in the fuzzy assignment problem. Proposed algorithms are illustrated with an example.
Abstract—Assignment problem represents a special case of linear programming problem used for allocating resources (mostly workforce) in an optimal way, it is a highly useful tool for operation and project managers for optimizing costs. In this paper a new approach is given to solve the balanced fuzzy assignment problem (FAP). Using this approach, we get an improved solution compared to the ...
Abstract: In this paper we introduce a new approach to solve fuzzy assignment problem namely, ones assignment method. Considering each fuzzy cost as a triangular fuzzy numbers the fuzzy assignment problem has been transformed into crisp values by using linguistic variables and ranking techniques and then it is solved by ones assignment method. The proposed method is a systematic procedure ...
In this paper, the fuzzy assignment problem is formulated to crisp assignment problem using Magnitude Ranking technique and Hungarian method has been applied to find an optimal solution.
In this paper, we investigate an assignment problem in which cost coefficients are triangular intuitionistic fuzzy numbers. In conventional assignment problem, cost is always certain. This paper develops an approach to solve an intuitionistic fuzzy
The parallel moving method provides an optimal solution to the fuzzy assignment problem in less number of iterations than the labeling algorithm. Abstract. A new method namely, parallel moving method is proposed to find an optimal solution to the fuzzy assignment problem considered in Lin and Wen [13]. We derive two theorems; one is related to an optimal solution to the fuzzy assignment ...
Fuzzy Assignment problems. Mohamed muamer. 2020, Journal of science - Misurata. In this paper, we deal with solving a fuzzy Assignment Problem (FAP), in this problem C denotes the cost for assigning the n jobs to the n workers and C has been considered to be triangular fuzzy numbers. The Hungarian method is used for solving FAP by using ranking ...
An approach to solve an intuitionistic fuzzy assignment problem where cost is not deterministic numbers but imprecise ones is developed andumerical examples show that an intuitionists fuzzy ranking method offers an effective tool for handling an intuitionism fuzzy. In this paper, we investigate an assignment problem in which cost coefficients are triangular intuitionistic fuzzy numbers. In ...
ABSTRACT: Assignment method consists of assigning a specific (worker) to a specific (job), assuming that the number of persons equal to the number of tasks available. Suppose the problem is taken into account in the fuzzy environment then the uncertainty can be minimized. This paper formulates Triangular Fuzzy Number, then through Matrix Ones Assignment method and direct method the problem is ...
PDF | In this paper, we investigate an assignment problem in which cost coefficients are triangular intuitionistic fuzzy numbers.
In this paper Branch and bound technique is applied to assignment problem with fuzzy cost with objective to minimise cost, fuzzy cost is assumed as triangular fuzzy number, Yager's ranking method has been used for ranking the fuzzy numbers, after transforming assignment problem into a crisp one using linguistic variables, assignment problem ...
When the cost values related to the assignment problem cannot be expressed precisely, fuzzy theory is used for a range of values. It has created fuzzy assignment problems.
Aggregators are a group of tools used in solving the aforementioned decision problems, including within fuzzy systems. Aggregating functions are a useful tool mainly in those artificial intelligence systems with problems arising from incomplete data.
In this paper, we deal with solving a fuzzy Assignment Problem (FAP), in this problem C denotes the cost for assigning the n jobs to the n workers and C has been considered to be triangular fuzzy numbers. The Hungarian method is used for solving FAP by using ranking function for fuzzy costs.