Grab your spot at the free arXiv Accessibility Forum
Help | Advanced Search

Computer Science > Machine Learning
Title: optimizing disease prediction with artificial intelligence driven feature selection and attention networks.
Abstract: The rapid integration of machine learning methodologies in healthcare has ignited innovative strategies for disease prediction, particularly with the vast repositories of Electronic Health Records (EHR) data. This article delves into the realm of multi-disease prediction, presenting a comprehensive study that introduces a pioneering ensemble feature selection model. This model, designed to optimize learning systems, combines statistical, deep, and optimally selected features through the innovative Stabilized Energy Valley Optimization with Enhanced Bounds (SEV-EB) algorithm. The objective is to achieve unparalleled accuracy and stability in predicting various disorders. This work proposes an advanced ensemble model that synergistically integrates statistical, deep, and optimally selected features. This combination aims to enhance the predictive power of the model by capturing diverse aspects of the health data. At the heart of the proposed model lies the SEV-EB algorithm, a novel approach to optimal feature selection. The algorithm introduces enhanced bounds and stabilization techniques, contributing to the robustness and accuracy of the overall prediction model. To further elevate the predictive capabilities, an HSC-AttentionNet is introduced. This network architecture combines deep temporal convolution capabilities with LSTM, allowing the model to capture both short-term patterns and long-term dependencies in health data. Rigorous evaluations showcase the remarkable performance of the proposed model. Achieving a 95% accuracy and 94% F1-score in predicting various disorders, the model surpasses traditional methods, signifying a significant advancement in disease prediction accuracy. The implications of this research extend beyond the confines of academia.
Comments: | 16 Pages, 4 Figures |
Subjects: | Machine Learning (cs.LG); Artificial Intelligence (cs.AI) |
Cite as: | [cs.LG] |
(or [cs.LG] for this version) | |
Focus to learn more arXiv-issued DOI via DataCite | |
Journal reference: | Vol. 20 No. 3s (2024) |
: | Focus to learn more DOI(s) linking to related resources |
Submission history
Access paper:.
- Other Formats

References & Citations
- Google Scholar
- Semantic Scholar
BibTeX formatted citation

Bibliographic and Citation Tools
Code, data and media associated with this article, recommenders and search tools.
- Institution
arXivLabs: experimental projects with community collaborators
arXivLabs is a framework that allows collaborators to develop and share new arXiv features directly on our website.
Both individuals and organizations that work with arXivLabs have embraced and accepted our values of openness, community, excellence, and user data privacy. arXiv is committed to these values and only works with partners that adhere to them.
Have an idea for a project that will add value for arXiv's community? Learn more about arXivLabs .

An official website of the United States government
The .gov means it’s official. Federal government websites often end in .gov or .mil. Before sharing sensitive information, make sure you’re on a federal government site.
The site is secure. The https:// ensures that you are connecting to the official website and that any information you provide is encrypted and transmitted securely.
- Publications
- Account settings
Preview improvements coming to the PMC website in October 2024. Learn More or Try it out now .
- Advanced Search
- Journal List
- J Healthc Eng
- v.2022; 2022

Identification and Prediction of Chronic Diseases Using Machine Learning Approach
Rayan alanazi.
Department of Computer Science, College of Science and Arts in Qurayyat, Jouf University, Sakakah, Saudi Arabia
Associated Data
The data used to support the findings of this study are included within the article.
Nowadays, humans face various diseases due to the current environmental condition and their living habits. The identification and prediction of such diseases at their earlier stages are much important, so as to prevent the extremity of it. It is difficult for doctors to manually identify the diseases accurately most of the time. The goal of this paper is to identify and predict the patients with more common chronic illnesses. This could be achieved by using a cutting-edge machine learning technique to ensure that this categorization reliably identifies persons with chronic diseases. The prediction of diseases is also a challenging task. Hence, data mining plays a critical role in disease prediction. The proposed system offers a broad disease prognosis based on patient's symptoms by using the machine learning algorithms such as convolutional neural network (CNN) for automatic feature extraction and disease prediction and K-nearest neighbor (KNN) for distance calculation to find the exact match in the data set and the final disease prediction outcome. A collection of disease symptoms has been performed for the preparation of the data set along with the person's living habits, and details related to doctor consultations are taken into account in this general disease prediction. Finally, a comparative study of the proposed system with various algorithms such as Naïve Bayes, decision tree, and logistic regression has been demonstrated in this paper.
1. Introduction
All over the world, chronic diseases are a critical issue in the healthcare domain. According to the medical statement, due to chronic diseases, the death rate of humans increases. The treatments given for this disease consume over 70% of the patient's income. Hence, it is highly essential to minimize the patient's risk factor that leads to death. The advancement in medical research makes health-related data collection easier [ 1 , 2 ]. The healthcare data includes the demographics, medical analysis reports, and the history of disease of the patient. The diseases caused could be varied based on the regions and the living habitats in that region. Hence, along with the disease data, the environmental condition and the living habitat of the patient should also be recorded in the data set.
In recent years, the healthcare domain is evolving more due to the integration of information technology (IT) in it. The intention to integrate IT in healthcare is to make the life of an individual more affordable with comfort as smartphones made one's life easier [ 3 ]. This could be possible by making healthcare to be intelligent, for instance, the invention of the smart ambulance, smart hospital facilities, and so on, which helps the patients and doctors in several ways [ 4 ]. The research on a specified region for patients affected by chronic diseases every year had been held and found that the difference between the patients in genderwise is very small, and it is found that the large number of patients were admitted in the year 2014 for treating chronic diseases. The use of structured and unstructured data provides highly accurate results instead of using only structured data. Since the unstructured data includes the doctor's records on the patients related to diseases and the patient's symptoms and grievances faced by them, explained by themselves, which is an added advantage when used along with the structured data that consists of the patient demographics, disease details, living habitats, and laboratory test results [ 5 , 6 ]. It is difficult to diagnose rare diseases. Hence, the use of self-reported behavioral data helps differentiate the individuals with rare diseases from the ones with common chronic diseases. By using machine learning approaches along with questionnaires, it is believed that the identification of rare diseases is highly possible [ 7 ].
In the last decade, some innovative technologies had been introduced to rapidly collect the data such as MRI (magnetic resonant imaging) readouts, ultrasonography, social media gained data, and electronically gained activity, behavioral, and clinical data. These big data sets of healthcare are high-dimensional, which means the number of features recorder per observation might be greater than the total observations. They are noisy, sparse, cross-sectional, and lacks statistical power. By applying machine learning techniques, the issues in the high-dimensional data sets can be overcome [ 8 ]. Machine learning contributes more in several domains. Many of the complex models make use of exiting larger training data, simultaneously at the edge of a major shift in healthcare epidemiology [ 9 ]. These data can enhance the knowledge gain in the risk factors of diseases to reduce healthcare-associated infections, improve patient risk stratification, and find the way of transmitting the infectious diseases [ 10 ]. Machine learning can facilitate the analysis of laboratory results and other details of patients for the early detection of diseases. The low-level data could be converted to high-level knowledge via knowledge discovery in the database so as to gain knowledge about the disease patterns to support early detection [ 11 ]. The data collected for creating a data set should be preprocessed for its missing values, and then only the important features needed for accurate disease prediction are selected so as to enhance the prediction accuracy and minimizing the time taken for model training [ 12 ].
In the era of the Internet and technologies, people are not concerned about their health and lives. As everyone is interested in surfing and social media activities, they ignore visiting hospitals for their health checkup. By taking this activity as an advantage, a machine learning model that takes the symptoms given as input and predicts the possibility and risk of the disease affected or the development of such diseases in an individual should be developed [ 13 , 14 ]. The more common chronic diseases are diabetes, cardiovascular diseases, cancer, strokes, hepatitis C, and arthritis. As these diseases persist for a long time and have a high mortality rate, the diagnosis of such diseases is highly important in the healthcare domain. Foreseeing the disease can help take preventive actions and avoid getting affected by it, and early detection of it can help provide better treatment [ 15 ]. There are various techniques in machine learning such as supervised, semisupervised, unsupervised, reinforcement, evolutionary, and deep learning. The problem is associated with the processing of extracted features from real data and structured as vectors [ 16 ]. The processing quality is based on the proper combination of those vectors. But, most of the times, the high dimensionality of the vectors or the discrepancies in the data makes a big issue. Hence, it is important to reduce the dimensionality of the data set even if it leads to a little loss of details to make the data set a highly compatible dimension. This reduction in the dimensionality of the data set improves the model performance [ 17 ].
The system of chronic diseases management is essential for those affected by such diseases and in need of proper medical assessment and treatment information [ 18 ]. Also, this system can be useful for individuals who are in need of self-care to improve their health condition, since it is proved that self-management is the primary care of those with chronic diseases, and it is considered as the unavoidable part of treatment. With the use of mobile applications, the health information of patients can be recorded, and they serve as a better tool to enable self-management [ 19 ]. To effectively predict a disease, information such as narration about the symptoms felt by the patients, details of consultation with medical practitioners, lab examination results, and computed tomography and X-ray images [ 20 ]. Little research is performed in identifying the accuracy and predictive power for developing a machine learning model with only information from lab examination results for the diagnosis of diseases. And, for performance enhancement, ensemble machine learning and deep learning model can be used [ 21 , 22 ]. In the healthcare domain, artificial intelligence (AI) plays a major role in automating the roles involved in disease diagnosis and treatment suggestions and also schedules perfect timing by the medical practitioners to perform various obligations that cannot be automated [ 23 ].
The major objective of the proposed system is to identify and predict chronic disease in an individual using a machine learning approach [ 24 , 25 ]. The data set comprises both the structured data that includes the patient's age, gender, height, weight, and so on, excluding the patient's personal information such as name and ID, and the unstructured data that includes the patient's symptoms, information related to consultation about the disease with the doctors, and the living habits of that individual [ 26 ]. These data are preprocessed for finding the missing values. They are then reconstructed to increase the quality of the model, thereby increasing the prediction accuracy. For prediction, the machine learning algorithms such as CNN and KNN are used [ 27 , 28 ]. This paper is organized as the details of the related works carried out while doing the research are given in Section 2 , the preliminaries of the algorithms used in given in Section 3 , the description of the proposed methodology in Section 4 , the result and discussion part are given in Section 5 followed by the conclusion in Section 6 , and finally, a list of references used in this study has been given.
2. Related Work
This section describes the related works that are performed in developing the proposed model for predicting chronic diseases. The following are the discussions made by reviewing the existing literature that helps develop the proposed system efficiently and effectively.
The objective variable of the study in [ 29 ] is the resource consumption such as medical and long-term care expenses and a predictive model for medical care using a random forest machine learning algorithm [ 30 ]. This method uses data of more than 100 pieces that includes preventive activities, clinical tests, and medical practices. This model uses mean decrease Gini for classification and for regression mean square error (MSE) is used [ 31 , 32 ]. The training model uses a grid search for hyperparameter tuning and is validated using K -fold cross-validation. Along with the objective variable, exploratory variables such as age, gender, and analysis period are also included, since the aim of this paper is proper management of the budget for medical care [ 33 ]. A review that highlights the applications of machine learning techniques in various medical practices such as predicting, diagnosing, and prognosis of diseases such as multiple sclerosis, autoimmune chronic kidney disease, autoimmune rheumatic disease, and inflammatory bowel disease and for the selection of treatments and stratification of patients; drug development; drug repurposing; target interpretation; and validation has been given in [ 34 , 35 ]. This paper also provides a detailed description of the challenges faced by the machine learning approaches such as the need for quality data in preparation of robust models, external model validation using the independent data set, difficulties faced during implementation of a model, and ethical concerns. A predictive model for chronic kidney disease is explained in [ 36 , 37 ]. This model is developed using four machine learning approaches such as support vector machine (SVM), logistic regression (LR), decision tree (DT), and KNN for classification purposes. The data set used in this paper is the Indian chronic kidney disease (CKD) that consists of 400 occurrences, 24 features, and 2 classes obtained from the UCI machine learning repository. The developed model is evaluated using a 5-fold cross-validation process, and the experiment is conducted on the Weka data mining tool and MATLAB and finally concluded that the SVM classifier attains higher accuracy when compared to the others.
A system that can predict multiple diseases with the help of various machine learning algorithms such as Naïve Bayes, KNN, DT, random forest, and SVM algorithms has been described in [ 38 ] to bridge the gap among the patients and the doctors to achieve their own goals. The existing approaches in the field of automatic disease prediction lack the patient's trust in the model's prediction and also reduce the need for doctors, which makes the doctors get panic about their livelihood. But this method integrates a module for doctor recommendation that solves both the issues by making sure the patient to trust due to the intervention of doctors and also improves the business of doctors. A model called PARAMO, which is a platform of a parallel predictive model that uses electronic health records (EHR) for healthcare analysis, has been implemented in [ 39 ]. This method comprises three phases, namely, the generation of the dependency graph, which removes redundancy and identifies dependency; then execution engine for dependency graph, which includes prioritizing, scheduling, and parallel execution; and finally the parallelization infrastructure. The PARAMO model is tested with three sets of real data, that is, small, medium, and large data sets that includes the medications, diagnosis data, and lab records, obtained from EHR that ranges from 5,000 to around 300,000 patients. In addition to this, the small and large sets include the procedure data, and the medium set includes the symptoms of heart failure that are taken from medical records [ 40 ]. An efficient recommendation system for chronic disease diagnosis has been demonstrated in [ 41 ]. This method uses a data mining approach. The data set used in this system includes medical data and two-dimensional data. The medical data include the data obtained from sensors or medical data entries, and the two-dimensional data include the external user and the item features. For enhancing the accuracy of prediction, the decision tree approach, which is a highly prevalent data mining approach, is used for classification. Various decision tree classifiers such as random forest, REP tree, decision stump, and J48 are involved in the creation of this predictive model. This model is tested with randomly selected 20 samples and found that the RF outperforms the other three algorithms.
Prediction of 3 types of immune diseases such as allergy, infectious, and autoimmune diseases using decision tree, maximum margin learning, and instance-based learning, respectively, has been given in [ 42 ]. The correlation between the classification of immunogens and its physicochemical properties is one of the purposes of this study. The immunogen data such as the stats of diseases, responses from B-cell, discontinuous epitope location, host, source organisms, and so on are collected from Immune Epitope Database (IEDB) and analyzed its 6 physicochemical properties such as PSSM (position-specific scoring matrix) information per position, hydrophilic scale, flexibility, antigenic propensity, hydropathy index, and side chain polarity. This system is tested using a method called leave-one-out cross-validation for the performance of prediction outcomes with parameters such as accuracy and F-score. A risk prediction model for predicting disease risks using a random forest machine learning approach from highly imbalance data has been described in [ 43 ]. The data set used in this approach is the Nationwide Inpatient Sample (NIS), which includes 8 million records of hospital stays with 126 clinical as well as nonclinical data. The nonclinical data comprises patient's demographics, hospital location, date and year of admission, pin code, treatment/diagnosis cost, and duration of stay in a hospital ward. The clinical data comprises the treatment procedures, its categories, diagnosis categories, and its codes. Each record has a vector containing 15 diagnosis codes characterized by International Classification of Diseases, 9 th Revision, Clinical Modification (ICD-9-CM). As the unbalance data produces undesirable results, a repeated random sampling method is employed to solve this issue. The developed model is evaluated using SVM, RF ensemble learning, bagging, and boosting algorithms. The study [ 44 ] demonstrates a novel adaptive probabilistic divergence-based feature selection algorithm to predict chronic kidney disease in its earlier stage. This algorithm is based on statistical and divergence information theory. For classification, the hyperparameterized logistic regression model is used in this study. The data set used in this approach is obtained from various hospitals and laboratories with information of 630 patients with 52 attributes, and this data set is given to the physician for verification of its correctness. The model developed is evaluated using the data sets of diabetes, heart, and kidney diseases, and the performance evaluation metrics followed in this study is the precision, recall, F1-score, and ROC (receiver operating characteristics) curve.
A system that enhances the risk prediction of a patient's health condition using a deep learning approach on big data and a revised fusion node model has been demonstrated in [ 45 ]. This deep learning model for extracting the data and logical inference is made of the combination of complex machine learning algorithm such as Bayesian fusion and neural networks. The architecture of this system consists of five layers, namely, the data layer that is responsible for data collection, data aggregation layer for data acquisition from several data sources and desired format changing, analytics layer to do proper analytics on the data aggregated, information exploration layer to create the output that makes the results of analytics understandable for users, and big data governance layer that is responsible for managing the above layers. Also, in this paper, the application of MapReduce is discussed for optimizing the analytics efficiency and also inspires the design of SOA (service-oriented architecture) for making the external systems easily access the results from analytics. A machine learning model of disease prediction cost has been implemented in [ 46 ] that uses big data, which includes structured and unstructured data for preparing the data set and the developed model is made available at affordable. The prediction algorithm used in this method is the decision tree algorithm and the MapReduce algorithm is applied for enhancing the efficiency of the operation. The advantages of this model are reduction in retrieval time of queries, improved accuracy. A method of predicting the risk of chronic kidney disease using zub machine learning approaches has been described in [ 47 , 48 ]. Two types of data sets are used in this method. One is from UCI with 400 instances and 35 features, and the other is a real-time data set obtained from Khulna City Medical College with 55 instances and 25 features. Data processing is done using Pandas and Numpy libraries, and the missing data are handled using median filtering. Feature extraction is performed using the Chi-square test. Model evaluation is performed using 10-fold cross-validation. Artificial neural network (ANN) and random forest algorithms are used for disease classification. This method is believed that it can predict the risk of chronic kidney disease in its earlier stage [ 49 – 52 ].
3. Preliminaries
3.1. chronic disease.
According to US National Center for Health Statistics, chronic diseases are diseases that last for a long period of time, that is, more than three months. These diseases are neither treated by medicines nor prevented by vaccines. The major cause of chronic diseases is the use of tobacco, unhealthy food habits, and lack of physical activity. Also, this disease can commonly be caused due to ageing. Chronic diseases include cardiovascular disease, cancer, arthritis, diabetes, obesity, epilepsy and seizures, and problems in oral health [ 35 ].
Cardiovascular disease includes heart disease and stroke, which highly lead to death. This disease is caused due to the use of tobacco, intake of nutritionless food, and lack of physical activity. When these activities are changed by the patient, they might have the chance to reduce the impact on controlling and preventing cardiovascular disease.
Next to cardiovascular disease, cancer such as colon cancer and breast cancer is considered the deadliest disease. It can be controlled only by prevention, early detection, and proper medical support. Minimizing the prevalence of environmental and behavioral factors that causes cancer reduces the chance risk of causing it.
The chronic disease such as arthritis causes inflammation in the joints, causes pain, and stiffness that increases due to ageing. There is an availability of cost-effective methods for reducing the effects caused by arthritis but are not used much. The effects of arthritis can be reduced by following moderate exercises regularly.
Diabetes is a serious and high-money-consuming disease. The impact of diabetes can be reduced by self-care and early detection of the disease [ 53 ]. Around 7 million people over the age of 65 or above are affected by this disease particularly type 2 diabetes.
Since 1980, obesity is more common in adults for all age groups. The one who is overweight or obese can develop the risk of getting high blood pressure (BP), heart diseases, diabetes, and arthritis. Obesity can also cause some types of cancers.
Epilepsy and seizures are highly costly in treatment [ 54 ]. This disease is common among all age groups, especially in young and elders.
Oral health problems are a crucial issue that attains special attention in the health of older people. This is a serious issue, since it affects the normal day-to-day actions of a person such as speak, chew, swallow, and maintain a nutritional food plan.
3.2. Convolutional Neural Network (CNN)
The ConvNet or CNN is an algorithm of deep learning that fetches the input and assigns the bias and weights to its several aspects and then distinguishes one from the other [ 55 ] as shown in Algorithm 1 . The major reason for using CNN is that it requires only few efforts in preprocessing the data when compared with other algorithms, since the CNN can learn to optimize the filters through automate learning [ 56 ]. The output layer of CNN can be calculated using the following expression:

Convolutional neural network algorithm.
3.3. K-Nearest Neighbor (KNN)
KNN is a supervised machine learning algorithm, which analyzes the similarities between the new data and the existing data and adds the new data into the category that is highly similar to the available categories [ 57 ] as shown in Algorithm 2 . The KNN can be used in classification as well as regression tasks, but it is most commonly used in classification. This algorithm is also called the lazy learner algorithm; since it will not learn instantly from the training data, it stores the data set and does its action during the classification process. The calculation of Euclidean distance is expressed mathematically as follows:

K-nearest neighbor algorithm.
4. Proposed Methodology
In this section, a detailed description of the data set creation, model preparation, and disease prediction has been given. The first action is data collection. Our proposed system collects structured and unstructured data obtained from various sources. After data collection, they are subjected to preprocessing and are split into cleaning and test data sets. Then the training data set is trained with the machine learning algorithms such as CNN and KNN to a number of epochs for improving the accuracy of the prediction results. After multiple epochs, once the desired target is achieved, the developed model is ready for testing.
At this step, the model is tested with the test data set to verify the model performance with brand-new data that were not used for training. If the model attains the desired accuracy in test data, then the proposed model is ready for deployment as shown in Figure 1 .

Architecture of proposed disease and risk prediction system.
4.1. Data Collection
The real-life data that includes structured data such as patient basic information including demographics, living habitat, and lab test results and the unstructured data such as the symptoms of the disease faced by the patient and their consultation with the doctor. The data set excludes the patient's personal details such as name, ID, and location so as to preserve their privacy.
4.2. Preprocecssing
The collected data are preprocessed for the availability of missing values in most of the structured data. Hence, it is essential to fill out the missed data or remove or modify them to enhance the quality of the data set. The preprocessing step also eliminates the commas, punctuations, and white spaces. Once the preprocessing of data has been completed, it is then subjected to feature extraction followed by disease prediction.
4.3. Model Description
As discussed above, the data set consists of both structured and unstructured data. The structured data comprises patient demographics and the data related to the cause for the disease such as age, gender height, weight, and so on, patient's living habitat, laboratory test results, and the disease that they are affected in tabular format. The unstructured data comprises patient's disease symptoms and the information about the interrogation with doctors in text format. The unstructured data is an added advantage of the prediction task to get a more accurate results. The data set is split into 80% for training and 20% for testing.
4.4. Disease Prediction Using CNN
The proposed system uses the CNN algorithm in the prediction of chronic disease. At first, the data set is converted into vector form, followed by word embedding to adopt zero values for filling the data. It is then given to the convolution layer.
The pooling layer takes the input from the convolution layer and follows the max pooling operation. The output of max pooling is given to the fully connected layer, and then finally, the output layer provides the classification results. Figure 2 shows the block diagram of the convolutional neural network.

Block diagram of convolutional neural network.
4.5. Distance Calculation Using KNN
In K -Nearest Neighbor (KNN), the value of K is known, and the features that are similar to the K value are called the nearest neighbor. The nearest neighbor to the known K value is chosen, and the nearest distance between them is calculated. The feature with less distance value is considered to be the exact match, which is the final disease prediction output. In the proposed system, Euclidean distance is used, since the result obtained by it is better when compared to other distance calculation methods. It is a nonparametric algorithm since it will not take decisions on original data. In KNN, the training input data are located in X and Y axes, and the test data are located in the plots of X and Y axes. Then, the plots of test data with less distance are chosen and are considered as the desired target. It is important to choose the value of the nearest K point should be always odd.
The calculation of Euclidean distance can be performed by using the following formula and is represented in Figure 3 :

Calculation of Euclidean distance.
5. Performance Evaluation
For evaluating the proposed disease prediction model, four performance evaluation metrics are used. The confusion matrix consists of the true positives (TP), which is the correct prediction of the target as a patient with chronic disease; the true negatives (TN), which is the correct prediction of the persons without diseases; false positives (FP), which is the incorrect prediction of the healthy person as a diseased person, and false negatives (FN), which is the incorrect prediction of the target as healthy persons. The following is the description of the four performance evaluation parameters.
5.1. Accuracy
The classification accuracy is described as the ratio of correct predicted values to the total predicted values and is depicted mathematically as follows:
5.2. Precision
The precision or positive predictive value (PPV) is described as the ratio of correct prediction to the total correct values including the true and false predictions and is depicted mathematically as follows:
5.3. Recall
The recall or sensitivity or true positive rate (TPR) is described as the ratio of correct predicted values to the sum of correct positive predictions and the incorrect negative predicted values and is depicted mathematically as follows:
5.4. F1-Score
The F-measure ( F β ) is described as the weighted average of the values obtained from the calculation of precision and recall parameters. Whenever the distribution of class is not even, then the value of F 1 − Score is highly important than the accuracy value. And whenever the values of false positives and negatives are dissimilar, the value of F 1 − Score is highly suitable. The F 1 − Score is depicted mathematically as follows:
By simplifying using β =1,
The obtained values of precision, recall, and F1-score of the proposed CNN and KNN model is compared with the values of the performance metrics of Naïve Bayes, decision tree, and logistic regression algorithms, and the results are tabulated in Table 1.
The accuracy is the important parameter since the prediction result is the important factor for the patient, and if it is wrong, then it will be a detriment to them. The other parameters such as precision, recall, and F1-score are for the evaluation of the model performance as shown in Table 1 .
Performance evaluation comparison.
Accuracy (%) | Precision (%) | Recall (%) | F1-score (%) | |
---|---|---|---|---|
Naïve Bayes | 52 | 52 | 80 | 65 |
Decision tree | 62 | 64 | 60 | 62 |
Logistic regression | 86 | 84 | 88 | 82 |
CNN and KNN | 96 | 93 | 99 | 97 |
Figure 4 shows the graphical representation of the comparison results of accuracies of the proposed and other algorithms. This graph illustrates the variations in the prediction accuracies of the four algorithms such as the Naïve Bayes, decision tree, logistic regression, and the proposed CNN and KNN algorithms as 52%, 62%, 86%, and 96%, respectively. This shows that the proposed system achieves the highest accuracy of 96% when compared to the other machine learning algorithms.

Comparison of accuracies of proposed and other algorithms.
Figure 5 shows the graphical representation of the comparison precision, recall, and F1-score values of the proposed and other algorithms. This graph illustrates the variations in the three performance evaluation parameters of the four algorithms such as the Naïve Bayes, decision tree, logistic regression, and the proposed CNN and KNN algorithms as 52%, 64%, 84%, and 93%, respectively, for precision; 80%, 605, 88%, and 99%, respectively, for recall; and 65%, 62%, 82%, and 97%, respectively, for F1-score. These results shows that the prosed model developed using CNN and KNN algorithm is considered to be the best of the remaining three algorithms with 93%, 99%, and 97% for precision, recall, and F1-score, respectively, which is higher when compared to the others.

Comparison of other performance evaluation metrics of proposed and other algorithms.
6. Conclusion
This paper proposed a method of identification and prediction of the presence of chronic disease in an individual using the machine learning algorithms such as CNN and KNN. The advantage of the proposed system is the use of both structured and unstructured data from real life for data set preparation, which lacks in many of the existing approaches. In this paper, the performance of the proposed model is compared with other algorithms such as Naïve Bayes, decision tree, and logistic regression algorithms. The results show that the proposed system provides an accuracy of 95% that is higher than that of the other two algorithms. It is highly believed that the proposed system can reduce the risk of chronic diseases by diagnosing them earlier and also reduces the cost for diagnosis, treatment, and doctor consultation.
Acknowledgments
This work was funded by the Deanship of Scientific Research at Jouf University under grant no. DSR-2021-02-0371.
Data Availability
Conflicts of interest.
The author declares that there are no conflicts of interest.
Disease outbreak prediction using natural language processing: a review
- Published: 06 August 2024
Cite this article
- Avneet Singh Gautam ORCID: orcid.org/0000-0002-8603-6455 1 &
- Zahid Raza ORCID: orcid.org/0000-0003-1906-6774 1
75 Accesses
Explore all metrics
Research on disease outbreak prediction has suddenly received an enormous interest owing to the COVID-19 pandemic. Natural language processing using user-generated text data has proven to be quite effective for the same. Disease outbreaks that occur frequently can be easily predicted, but novel disease outbreaks are difficult to predict. This review work attempts to summarize the research concerning disease outbreaks and the use of datasets such as news headlines, tweets, and search engine queries using natural language processing techniques. Existing state-of-the-art systems have been analytically discussed with their contributions and limitations. This work is an insight into the existing research in the domain of disease outbreak prediction. A total of 146 articles were reviewed in this study, and results show that news and Twitter datasets are being used most to predict disease outbreaks. This research underlines the fact that numerous works are available in the literature based on specific outbreak-related Internet-sourced text data, viz. news, tweets, and search engine queries. However, this becomes a limitation for any disease outbreak prediction system as it can predict only specific disease outbreaks and motivates the development of systems capable of disease outbreak prediction without any bias.
This is a preview of subscription content, log in via an institution to check access.
Access this article
Subscribe and save.
- Get 10 units per month
- Download Article/Chapter or eBook
- 1 Unit = 1 Article or 1 Chapter
- Cancel anytime
Price includes VAT (Russian Federation)
Instant access to the full article PDF.
Rent this article via DeepDyve
Institutional subscriptions
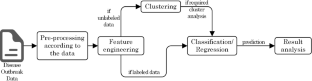
Similar content being viewed by others
BIOPAK FLASHER: Epidemic Disease Monitoring and Detection in Pakistan Using Text Mining
Automated Disease Outbreak Detection and Analysis
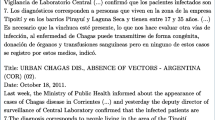
Digital surveillance in Latin American diseases outbreaks: information extraction from a novel Spanish corpus
Data availability.
Not applicable.
Code Availability
Not Applicable.
Authors considered media articles and news media as news data in this study.
Twitter re-branded as X in July 2023.
Disease outbreak news. https://www.who.int/emergencies/disease-outbreak-news . Accessed 07 Aug 2022
Who emro. http://www.emro.who.int/health-topics/disease-outbreaks/index.html . Accessed 07 Aug 2022
Liu J, Xia S (2020) Computational epidemiology: from disease transmission modeling to vaccination decision making. Springer, Nature
Book Google Scholar
Pley C, Evans M, Lowe R, Montgomery H, Yacoub S (2021) Digital and technological innovation in vector-borne disease surveillance to predict, detect, and control climate-driven outbreaks. Lancet Planet Health 5(10):739–745. https://doi.org/10.1016/S2542-5196(21)00141-8
Article Google Scholar
BlueDot-Who We Are. https://bluedot.global/research/ . Accessed 15 Aug 2022
Bogoch II, Watts A, Thomas-Bachli A, Huber C, Kraemer MUG, Khan K (2020) Potential for global spread of a novel coronavirus from China. J Travel Med. https://doi.org/10.1093/jtm/taaa011
The true death toll of COVID-19 (2021) estimating global excess mortality. Technical report, World Bank. World Bank. Accessed 4 Sep 2023
World Bank Group (2022) Chapter 1. the economic impacts of the COVID-19 crisis. Technical report, WHO. WHO. Accessed 4 Sep 2023
Fernstrom A, Goldblatt M (2013) Aerobiology and its role in the transmission of infectious diseases. J Pathog 2013:1–13. https://doi.org/10.1155/2013/493960
Bogoch II, Watts A, Thomas-Bachli A, Huber C, Kraemer MU, Khan K (2020) Pneumonia of unknown aetiology in wuhan, china: potential for international spread via commercial air travel. J Travel Med 27(2):008
Fong SJ, Dey N, Chaki J (2021) Ai-empowered data analytics for coronavirus epidemic monitoring and control. In: SpringerBriefs in applied sciences and technology, pp 47–71. https://doi.org/10.1007/978-981-15-5936-5_3
Bogoch II (2016) Anticipating the international spread of zika virus from brazil. The Lancet 387(10016):335–336. https://doi.org/10.1016/S0140-6736(16)00080-5
Nikolaou P, Dimitriou L (2020) Identification of critical airports for controlling global infectious disease outbreaks: stress-tests focusing in europe. J Air Transp Manag 85:101819. https://doi.org/10.1016/j.jairtraman.2020.101819
Allam Z, Dey G, Jones D (2020) Artificial intelligence (ai) provided early detection of the coronavirus (covid-19) in china and will influence future urban health policy internationally. Ai 1(2):156–165. https://doi.org/10.3390/ai1020009
Epidemic Tracker Metabiota. https://www.metabiota.com/epidemic-tracker . Accessed 13 Aug 2022
Raynaud M (2021) Impact of the covid-19 pandemic on publication dynamics and non-covid-19 research production. BMC Med Res Methodol 21(1):1–10. https://doi.org/10.1186/s12874-021-01404-9
Else H (2020) Covid in papers: a torrent of science. Nature 588:553. https://doi.org/10.1038/d41586-020-03564-y
Alsiri NF, Alhadhoud MA, Palmer S (2021) The impact of the covid-19 on research. J Clin Epidemiol 129:124–125. https://doi.org/10.1016/j.jclinepi.2020.09.040
Yu F, Mani N (2020) How american academic medical/health sciences libraries responded to the covid-19 health crisis: an observational study. Data Inf Manag 4(3):200–208. https://doi.org/10.2478/dim-2020-0013
Jurafsky D, Martin JH (2000) Speech and language processing: an introduction to natural language processing. Computational Linguistics and Speech Recognition. Pearson, Upper Saddle River, NJ
Google Scholar
Bishop C, Nasrabadi M, Nasser M (2006) Pattern recognition and machine learning, vol 4. Springer, New York, NY
Mitchell T (1997) Machine learning. McGraw-Hill Professional, New York, NY
Laosiritaworn Y, Laosiritaworn WS, Laosiritaworn Y (2018) Monte carlo, design of experiment, and neural network modeling of basic reproduction number in disease spreading system. In: 2018 7th international conference on industrial technology and management, ICITM 2018, vol 2018-Janua, pp 345–349. https://doi.org/10.1109/ICITM.2018.8333973
Kiran CRS, Naveen C, Kumar DA, Saiteja T, Karthikeyan C (2021) Prediction of epidimic outbreak using deep learning methods. In: Proceedings of the 6th international conference on inventive computation technologies, ICICT, pp 995–1000. https://doi.org/10.1109/ICICT50816.2021.9358710
Ardabili SF, Mosavi A, Ghamisi P, Ferdinand F, Varkonyi-Koczy AR, Reuter U, Rabczuk T, Atkinson PM (2020) COVID-19 outbreak prediction with machine learning. Algorithms 13(10):249. https://doi.org/10.3390/a13100249
Article MathSciNet Google Scholar
Singh S, Parmar KS, Kumar J, Makkhan SJS (2020) Development of new hybrid model of discrete wavelet decomposition and autoregressive integrated moving average (arima) models in application to one month forecast the casualties cases of covid-19. Chaos, Solitons Fractals 135:1–8. https://doi.org/10.1016/j.chaos.2020.109866
Kane MJ, Price N, Scotch M, Rabinowitz P (2014) Comparison of arima and random forest time series models for prediction of avian influenza h5n1 outbreaks. BMC Bioinform. https://doi.org/10.1186/1471-2105-15-276
Duan X, Zhang X (2020) Arima modelling and forecasting of irregularly patterned covid-19 outbreaks using japanese and south korean data. Data Brief 31:105779. https://doi.org/10.1016/j.dib.2020.105779
Chae S, Kwon S, Lee D (2018) Predicting infectious disease using deep learning and big data. Int J Environ Res Public Health. https://doi.org/10.3390/ijerph15081596
Wang Y (2021) Estimating the covid-19 prevalence and mortality using a novel data-driven hybrid model based on ensemble empirical mode decomposition. Sci Rep 11(1):1–17. https://doi.org/10.1038/s41598-021-00948-6
He Y, Liu H, Xie X, Gu W, Mao Y, Luo W (2021) Infectious disease prediction and analysis based on parametric-nonparametric hybrid model. ACM Int Conf Proc Ser. https://doi.org/10.1145/3469213.3471317
Khotimah PH, Rozie AF, Nugraheni E, Arisal A, Suwarningsih W, Purwarianti A (2020-11) Deep learning for dengue fever event detection using online news. In: Proceeding - 2020 international conference on radar, antenna, microwave, electronics and telecommunications, ICRAMET 2020, pp 261–266. https://doi.org/10.1109/ICRAMET51080.2020.9298630
Wakamiya S, Kawai Y, Aramaki E (2018) Twitter-based influenza detection after flu peak via tweets with indirect information: Text mining study. JMIR Public Health Surveill 4(3):65
Nsoesie EO, Oladeji O, Abah ASA, Ndeffo-Mbah ML (2021) Forecasting influenza-like illness trends in cameroon using google search data. Sci Rep 11(1):1–11. https://doi.org/10.1038/s41598-021-85987-9
Karaduzović-Hadžiabdić K, Spahić R, Tahirović E (2022) Evaluation of ibm watson natural language processing service to predict influenza-like illness outbreaks from twitter data. Period Eng Natl Sci 10(1):122–137. https://doi.org/10.21533/pen.v10i1.2454
Amin S (2020) Detecting dengue/flu infections based on tweets using lstm and word embedding. IEEE Access 8:189054–189068. https://doi.org/10.1109/ACCESS.2020.3031174
Aziz A, Aziz A (2021) Dengue cases prediction using machine learning approach. iRASD J Comp Info Tech 2(1):13–25
Amin S, Uddin MI, Zeb MA, Alarood AA, Mahmoud M, Alkinani MH (2021) Detecting information on the spread of dengue on twitter using artificial neural networks. Comput Mater Continua 67(1):1317–1332. https://doi.org/10.32604/cmc.2021.014733
Huang Y, Zhang P, Wang Z, Lu Z, Wang Z (2022) Hfmd cases prediction using transfer one-step-ahead learning. Neural Process Lett. https://doi.org/10.1007/s11063-022-10795-9
Wang Y, Cao Z, Zeng D, Wang X, Wang Q (2020) Using deep learning to predict the hand-foot-and-mouth disease of enterovirus a71 subtype in beijing from 2011 to 2018. Sci Rep 10(1):1–10. https://doi.org/10.1038/s41598-020-68840-3
Meng D, Xu J, Zhao J (2021) Analysis and prediction of hand, foot and mouth disease incidence in china using random forest and xgboost. PLoS ONE 16(12):1–16. https://doi.org/10.1371/journal.pone.0261629
Fung ICH (2013) Chinese social media reaction to the mers-cov and avian influenza a(h7n9) outbreaks. Infect Dis Poverty 2(1):1–12. https://doi.org/10.1186/2049-9957-2-31
Odlum M, Yoon S (2015) What can we learn about the ebola outbreak from tweets? Am J Infect Control 43(6):563–571. https://doi.org/10.1016/j.ajic.2015.02.023
Joshi A (2020) Automated monitoring of tweets for early detection of the 2014 ebola epidemic. PLoS ONE 15(3):1–10. https://doi.org/10.1371/journal.pone.0230322
Park J, Chaffee AW, Harrigan RJ, Schoenberg FP (2022) A non-parametric hawkes model of the spread of ebola in west africa. J Appl Stat 49(3):621–637. https://doi.org/10.1080/02664763.2020.1825646
Fung ICH (2018) Twitter and middle east respiratory syndrome, south korea, 2015: A multi-lingual study. Infect Dis Health 23(1):10–16. https://doi.org/10.1016/j.idh.2017.08.005
Lee H (2019) Stochastic and spatio-temporal analysis of the middle east respiratory syndrome outbreak in south korea, 2015. Infect Dis Model 4:227–238. https://doi.org/10.1016/j.idm.2019.06.002
Balashankar A, Dugar A, Subramanian L, Fraiberger S (2019) Reconstructing the mers disease outbreak from news. In: COMPASS 2019 - proceedings of the 2019 conference on computing and sustainable societies, pp 272–280. https://doi.org/10.1145/3314344.3332498
Lampos V (2021) Tracking covid-19 using online search. NPJ Digit Med 4(1):17. https://doi.org/10.1038/s41746-021-00384-w
Menaouer B, Zoulikha D, El-Houda K, Sabri M, Nada M (2022) Coronavirus-pneumonia-classification-using-x-ray-and-ct-scan-images-with-deep-convolutional-neural-network-models. J Inf Technol Res 15:1–23. https://doi.org/10.4018/JITR.299391
Menaouer B, Abdeldjouad FZ, Sabri M (2022) Multi-class-sentiment-classification-for-healthcare-tweets-using-supervised-learning-techniques. Int J Serv Sci Manag Eng Technol 13:1–23. https://doi.org/10.4018/IJSSMET.298669
Menaouer B, Sabri M, Nada M (2020) Towards a model to improve boolean knowledge mapping by using text mining and its applications: Case study in healthcare. Int J Inf Retriev Res 10:35–56. https://doi.org/10.4018/IJIRR.2020070103
Fast SM, Kim L, Cohn EL, Mekaru SR, Brownstein JS, Markuzon N. Predicting social response to infectious disease outbreaks from internet-based news streams. Ann Oper Res 263(1–2), 551–564. https://doi.org/10.1007/s10479-017-2480-9
Kim M, Chae K, Lee S, Jang HJ, Kim S (2020) Automated classification of online sources for infectious disease occurrences using machine-learning-based natural language processing approaches. Int J Environ Res Public Health 17(24):1–13. https://doi.org/10.3390/ijerph17249467
Azam N, Tahir B, Mehmood MA (2020) News-EDS: news based epidemic disease surveillance using machine learning. https://doi.org/10.1109/ICOSST51357.2020.9333083
Freifeld CC, Mandl KD, Reis BY, Brownstein JS (2008) Healthmap: Global infectious disease monitoring through automated classification and visualization of internet media reports. J Am Med Inform Assoc 15(2):150–157. https://doi.org/10.1197/jamia.M2544
Chakraborty S, Subramanian L (2017) Extracting signals from news streams for disease outbreak prediction
International Health Regulations (IHR) Secretariat. https://www.who.int/teams/ihr . Accessed 07 Aug 2022
Weekly bulletins on outbreaks and other emergencies | WHO | Regional Office for Africa. https://www.afro.who.int/health-topics/disease-outbreaks/outbreaks-and-other-emergencies-updates . Accessed 07 Aug 2022
Nigeria Centre for Disease Control. https://ncdc.gov.ng/ . Accessed 07 Aug 2022
Command and Control Center. https://www.moh.gov.sa/en/CCC . Accessed 07 Aug 2022
Azzedin F, Ghaleb M, Mohammed SA, Yazdani J (2019) Framework for disease outbreak notification systems with an optimized federation layer. Int J Adv Comput Sci Appl 10(2):546–553. https://doi.org/10.14569/ijacsa.2019.0100268
Ajagbe SA, Adigun MO (2023) Deep learning techniques for detection and prediction of pandemic diseases: a systematic literature review. Multimedia Tools Appl:1–35
Alessa A, Faezipour M (2018) A review of influenza detection and prediction through social networking sites. Theor Biol Med Model 15(1):2
Alruily M (2018) A review on event-based epidemic surveillance systems that support the arabic language. Int J Adv Comput Sci Appl. https://doi.org/10.14569/IJACSA.2018.0911102
Eckhardt M, Hultquist JF, Kaake RM, Hüttenhain R, Krogan NJ (2020) A systems approach to infectious disease. Nat Rev Genet 21(6):339–354
Singh R, Singh R (2023) Applications of sentiment analysis and machine learning techniques in disease outbreak prediction – a review. Materials Today: Proceedings 81, 1006–1011. https://doi.org/10.1016/j.matpr.2021.04.356 . International Virtual Conference on Sustainable Materials (IVCSM-2k20)
Kaur I, Sandhu AK, Kumar Y (2022) Artificial intelligence techniques for predictive modeling of vector-borne diseases and its pathogens: a systematic review. Arch Comput Methods Eng 29(6):3741–3771
Hu S-n, Cheng X, Chen D (2021) Comparative study on early warning methods of infectious diseases. In: E3S Web of Conferences, vol. 251, p. 03084. EDP Sciences
Sylvestre E, Joachim C, Cecilia-Joseph E, Bouzille G, Campillo-Gimenez B, Cuggia M, Cabié A (2022) Data-driven methods for dengue prediction and surveillance using real-world and big data: a systematic review. PLoS Negl Trop Dis 16(1):0010056
Siang TK, Ramachandran CR, Meskaran F (2021) Dengue disease prediction using machine learning algorithms: a review. J Appl Technol Innov 5(4):24–29
Batista EDdA, Bublitz FM, Araujo WCd, Lira RV (2020) Dengue prediction through Machine Learning and Deep Learning: A Scoping review protocol
Steele L, Orefuwa E, Dickmann P (2016) Drivers of earlier infectious disease outbreak detection: a systematic literature review. Int J Infect Dis 53:15–20. https://doi.org/10.1016/j.ijid.2016.10.005
Hussain-Alkhateeb L, Rivera Ramirez T, Kroeger A, Gozzer E, Runge-Ranzinger S (2021) Early warning systems (ewss) for chikungunya, dengue, malaria, yellow fever, and zika outbreaks: What is the evidence? a scoping review. PLoS Negl Trop Dis 15(9):0009686
Fung IC-H, Duke CH, Finch KC, Snook KR, Tseng P-L, Hernandez AC, Gambhir M, Fu K-W, Tse ZTH (2016) Ebola virus disease and social media: a systematic review. Am J Infect Control 44(12):1660–1671
Sylvestre E, Cuggia M, Cabié A, Joachim C (2020) Harnessing big data and machine learning methods for dengue surveillance and prediction: a systematic review. International prospective register of systematic reviews
Syrowatka A, Kuznetsova M, Alsubai A, Beckman AL, Bain PA, Craig KJT, Hu J, Jackson GP, Rhee K, Bates DW (2021) Leveraging artificial intelligence for pandemic preparedness and response: a scoping review to identify key use cases. NPJ Digital Med 4(1):96
Baldominos A, Puello A, Oğul H, Aşuroğlu T, Colomo-Palacios R (2020) Predicting infections using computational intelligence-a systematic review. IEEE Access 8:31083–31102
Phoobane P, Masinde M, Mabhaudhi T (2022) Predicting infectious diseases: a bibliometric review on africa. Int J Environ Res Public Health 19(3):1893
Jonkmans N, D’Acremont V, Flahault A (2021) Scoping future outbreaks: a scoping review on the outbreak prediction of the who blueprint list of priority diseases. BMJ Glob Health 6(9):006623
Wilson AE, Lehmann CU, Saleh SN, Hanna J, Medford RJ (2021) Social media: a new tool for outbreak surveillance. Antimicrob Stewardship Healthcare Epidemiol 1(1):50
Aiello AE, Renson A, Zivich P (2020) Social media-and internet-based disease surveillance for public health. Annu Rev Public Health 41:101
Tang L, Bie B, Park S-E, Zhi D (2018) Social media and outbreaks of emerging infectious diseases: a systematic review of literature. Am J Infect Control 46(9):962–972
Gupta A, Katarya R (2019) Social media based surveillance systems for healthcare using machine learning: a systematic review. J Biomed Inform 108:103500. https://doi.org/10.1016/j.jbi.2020.103500
Alfred R, Obit JH (2021) The roles of machine learning methods in limiting the spread of deadly diseases: a systematic review. Heliyon 7(6)
Swaan C, Broek A, Kretzschmar M, Richardus JH (2018) Timeliness of notification systems for infectious diseases: a systematic literature review. PLoS ONE 13(6):0198845
Nolasco D, Oliveira J (2019) Subevents detection through topic modeling in social media posts. Futur Gener Comput Syst 93:290–303. https://doi.org/10.1016/j.future.2018.09.008
Blei DM, Ng AY, Jordan MI (2003) Latent dirichlet allocation. J Mach Learn Res 3(null):993–1022
Dumais ST (2004) Latent semantic analysis. Ann Rev Inf Sci Technol 38(1):188–230. https://doi.org/10.1002/ARIS.1440380105
Dumais ST, Furnas GW, Landauer TK, Deerwester S, Harshman R (1988) Using latent semantic analysis to improve access to textual information. In: Conference on Human Factors in Computing Systems - Proceedings, vol. Part F130202, pp 281–285. https://doi.org/10.1145/57167.57214
Deerwester S, Dumais ST, Furnas GW, Landauer TK, Harshman R. Indexing by Latent Semantic Analysis. https://doi.org/10.1002/(SICI)1097-4571(199009)41:6
Hofmann T (1999) Probabilistic latent semantic indexing. In: Proceedings of the 22nd annual international ACM SIGIR conference on research and development in information retrieval, SIGIR, pp 50–57. https://doi.org/10.1145/312624.312649
Choi S, Lee J, Kang MG, Min H, Chang YS, Yoon S (2017) Large-scale machine learning of media outlets for understanding public reactions to nation-wide viral infection outbreaks. Methods 129:50–59. https://doi.org/10.1016/j.ymeth.2017.07.027
PAHO/WHO Regional Zika Epidemiological Update August 25 2017. https://www.paho.org/hq/index.php . Accessed 02 Aug 2022
Chen L, Hossain KSMT, Butler P, Ramakrishnan N, Prakash BA (2014) Flu gone viral: Syndromic surveillance of flu on twitter using temporal topic models. In: Proceedings - IEEE international conference on data mining (january), 755–760. https://doi.org/10.1109/ICDM.2014.137
Dai X, Bikdash M, Meyer B (2017) From social media to public health surveillance: word embedding based clustering method for twitter classification. Conf Proc IEEE SOUTHEASTCON (Table I). https://doi.org/10.1109/SECON.2017.7925400
Kim M, Kim I, Lee M, Jang B (2018) Poster abstract: Worldwide emerging disease-related information extraction system from news data. In: SenSys 2018 - proceedings of the 16th conference on embedded networked sensor systems, pp 331–332. https://doi.org/10.1145/3274783.3275168
Erraguntla M, Zapletal J, Lawley M (2019) Framework for infectious disease analysis: a comprehensive and integrative multi-modeling approach to disease prediction and management. Health Inform J 25(4):1170–1187. https://doi.org/10.1177/1460458217747112
Noble P-JM, Appleton C, Radford AD, Nenadic G (2021) Using topic modelling for unsupervised annotation of electronic health records to identify an outbreak of disease in uk dogs. PLoS ONE 16(12):0260402. https://doi.org/10.1371/journal.pone.0260402
Jang B, Kim M, Kim I, Kim JW (2021) Eagleeye: a worldwide disease-related topic extraction system using a deep learning based ranking algorithm and internet-sourced data. Sensors. https://doi.org/10.3390/s21144665
Beckhaus J, Becher H, Belau MH (2022) The use and applicability of internet search queries for infectious disease surveillance in low- to middle-income countries. One Health Implementation Res 2(1):15–28. https://doi.org/10.20517/ohir.2022.01
Thapen N, Simmie D, Hankin C (2016) The early bird catches the term: Combining twitter and news data for event detection and situational awareness. J Biomed Seman 7(1):1–14. https://doi.org/10.1186/s13326-016-0103-z
Ester M, Kriegel HP, Sander J, Xu X (1996) A density-based algorithm for discovering clusters in large spatial databases with noise. Kdd 96(34):226
Valentin S, Lancelot R, Roche M (2021): Identifying associations between epidemiological entities in news data for animal disease surveillance. In: Artificial intelligence in agriculture, vol 5, pp 163–174. https://doi.org/10.1016/j.aiia.2021.07.003
Villanueva-Miranda I, Akbar M (2021) Integrating heterogeneous data for a multi-disease outbreak detection framework. In: 2021 IEEE international conference on big data (big data), pp 2828–2837. Big Data. https://doi.org/10.1109/BigData52589.2021.9671841
Zhou X, Menche J, Barabási AL, Sharma A (2014) Human symptoms-disease network. Nat Commun. https://doi.org/10.1038/ncomms5212
Nguyen DQ, Vu T, Nguyen AT, Research V (2020) BERTweet: A pre-trained language model for English Tweets. https://doi.org/10.18653/V1/2020.EMNLP-DEMOS.2
Xie R, Chu SKW, Chiu DKW, Wang Y (2021) Exploring public response to covid-19 on weibo with lda topic modeling and sentiment analysis. Data Inf Manag 5(1):86–99. https://doi.org/10.2478/dim-2020-0023
Gupta A, Katarya R (2021) A novel lda-based framework to forecast covid-19 trends. SSRN Electron J. https://doi.org/10.2139/ssrn.3833706
Yoon J, Kim JW, Jang B (2018) Ditex: disease-related topic extraction system through internet-based sources. PLoS ONE 13(8):1–16. https://doi.org/10.1371/journal.pone.0201933
Jang B, Kim I, Kim JW (2021) Effective training data extraction method to improve influenza outbreak prediction from online news articles: Deep learning model study. JMIR Med Inform. https://doi.org/10.2196/23305
Abbood A, Ullrich A, Busche R, Ghozzi S (2020) Eventepi-a natural language processing framework for event-based surveillance. PLoS Comput Biol 16(11):1–16. https://doi.org/10.1371/journal.pcbi.1008277
EpiTator: EpiTator annotates epidemiological information in text documents. https://github.com/ecohealthalliance/EpiTator . Accessed 07 Aug 2022
Hassan S, Khan A, Nasser N, Alharbi A, Alyami H (2020) Recurrent neural networks with TF-IDF embedding technique for detection and classification in tweets of dengue disease. https://doi.org/10.1109/ACCESS.2020.3009058
Id BJ (2019) Word2vec convolutional neural networks for classification of news articles and tweets. PLoS ONE 14(8):1–20. https://doi.org/10.1371/journal.pone.0220976
Karwande G, Chintalapati RV, Vattikonda SK (2021) Deep learning based disease outbreak prediction by anomaly detection. https://doi.org/10.13140/RG.2.2.15239.11680
Kohonen T (1990) The self-organizing map. Proc IEEE 78(9):1464–1480. https://doi.org/10.1109/5.58325
Liu FT, Ting KM, Zhou ZH (2008) Isolation forest. In: Proceedings - IEEE international conference on data mining, vol ICDM, pp 413–422. https://doi.org/10.1109/ICDM.2008.17
Munir M, Siddiqui SA, Dengel A, Ahmed S (2019) Deepant: a deep learning approach for unsupervised anomaly detection in time series. IEEE Access 7:1991–2005. https://doi.org/10.1109/access.2018.2886457
Valentin S (2021) Padi-web 3.0: a new framework for extracting and disseminating fine-grained information from the news for animal disease surveillance. One Health. https://doi.org/10.1016/j.onehlt.2021.100357
Valentin S (2019) Padi-web: a multilingual event-based surveillance system for monitoring animal infectious diseases. Comput Electron Agric 169:105163. https://doi.org/10.1016/j.compag.2019.105163
What is custom Translator? https://docs.microsoft.com/en-us/azure/cognitive-services/translator/custom-translator/overview . Online]. Available:
Valentin S, Arsevska E, Vilain A, Waele V, Lancelot R, Roche M (2021) Annotation of epidemiological information in animal disease-related news articles: guidelines. http://arxiv.org/abs/2101.06150. arXiv [cs.IR]. [Online]. Available:
Rabatel J, Arsevska E, Roche M (2019) Padi-web corpus: labeled textual data in animal health domain. Data Brief 22:643–646. https://doi.org/10.1016/j.dib.2018.12.063
Lossio-Ventura JA, Jonquet C, Roche M, Teisseire M (2016) Biomedical term extraction: overview and a new methodology. Inf Retr Boston 19(1–2):59–99. https://doi.org/10.1007/s10791-015-9262-2
Fisichella M (2021) Unified approach to retrospective event detection for event- based epidemic intelligence. Int J Digit Libr 22(4):339–364. https://doi.org/10.1007/s00799-021-00308-9
Linge JP (2010) Medisys: medical information system. In: Advanced ICTs for disaster management and threat detection: collaborative and distributed frameworks, pp 131–142. https://doi.org/10.4018/978-1-61520-987-3.ch009
Li Z, Wang B, Li M, Ma WY (2005) A probabilistic model for retrospective news event detection. In: SIGIR 2005 - Proceedings of the 28th annual international ACM SIGIR conference on research and development in information retrieval, pp 106–113. https://doi.org/10.1145/1076034.1076055
Gu D (2021) The global infectious diseases epidemic information monitoring system: Development and usability study of an effective tool for travel health management in china. JMIR Public Health Surveill 7(2):1–15. https://doi.org/10.2196/24204
Arsevska E (2018) Web monitoring of emerging animal infectious diseases integrated in the french animal health epidemic intelligence system. PLoS ONE 13(8):1–25. https://doi.org/10.1371/journal.pone.0199960
Arsevska E, Roche M, Hendrikx P, Chavernac D, Falala S, Lancelot R, Dufour B (2016) Identification of terms for detecting early signals of emerging infectious disease outbreaks on the web. Comput Electron Agric 123:104–115
Jang B, Lee M, Kim JW (2019) Peacock: a map-based multitype infectious disease outbreak information system. IEEE Access 7:82956–82969. https://doi.org/10.1109/ACCESS.2019.2924189
Şerban O, Thapen N, Maginnis B, Hankin C, Foot V (2019) Real-time processing of social media with sentinel: a syndromic surveillance system incorporating deep learning for health classification. Inf Process Manag 56(3):1166–1184. https://doi.org/10.1016/j.ipm.2018.04.011
Lampos V, Cristianini N (2012) Nowcasting events from the social web with statistical learning. ACM Trans Intell Syst Technol 3(4):1–22. https://doi.org/10.1145/2337542.2337557
Morbidity and Mortality Weekly Report (MMWR. https://www.cdc.gov/mmwr/index.html. Online]
Thapen N, Simmie D, Hankin C, Gillard J (2016) Defender: detecting and forecasting epidemics using novel data-analytics for enhanced response. PLoS ONE 11(5):0155417. https://doi.org/10.1371/journal.pone.0155417
Europe media monitor. Trends Analyt Chem 9(1), (1990)
Maimon OZ, Rokach L (2005) Data mining and knowledge discovery handbook. Springer, New York, NY
PULS Project: Surveillance of Global News Media. http://puls.cs.helsinki.fi/static/index.html . Accessed 17 Feb 2023
Madoff LC (2004) Promed-mail: an early warning system for emerging diseases. Clin Infect Dis 39(2):227–232. https://doi.org/10.1086/422003
Mawudeku A, Blench M (2005) Global public health intelligence network. In: Proceedings of Machine Translation Summit X: Invited Papers
Collier N (2008) Biocaster: detecting public health rumors with a web-based text mining system. Bioinformatics 24(24):2940–2941. https://doi.org/10.1093/bioinformatics/btn534
Collier N (2006) A multilingual ontology for infectious disease surveillance: rationale, design and challenges. Lang Resour Eval 40(3):405. https://doi.org/10.1007/s10579-007-9019-7
Baker QB, Shatnawi F, Rawashdeh S (2022) Forecasting epidemic diseases with arabic twitter data and who reports using machine learning techniques. Bull Electr Eng Inform 11(2):738–749. https://doi.org/10.11591/eei.v11i2.3447
Amin S (2021) Early detection of seasonal outbreaks from twitter data using machine learning approaches. Complexity. https://doi.org/10.1155/2021/5520366
Guidry JPD, Jin Y, Orr CA, Messner M, Meganck S (2017) Ebola on instagram and twitter: How health organizations address the health crisis in their social media engagement. Public Relat Rev 43(3):477–486. https://doi.org/10.1016/j.pubrev.2017.04.009
Yousefinaghani S, Dara R, Poljak Z, Bernardo TM, Sharif S (2019) The assessment of twitter’s potential for outbreak detection: Avian influenza case study. Sci Rep 9(1):1–17. https://doi.org/10.1038/s41598-019-54388-4
Fu KW, Liang H, Saroha N, Tse ZTH, Ip P, Fung ICH (2016) How people react to zika virus outbreaks on twitter? a computational content analysis. Am J Infect Control 44(12):1700–1702. https://doi.org/10.1016/j.ajic.2016.04.253
Alkouz B, Aghbari Z, Al-Garadi MA, Sarker A (2022) Deepluenza: deep learning for influenza detection from twitter. Expert Syst Appl. https://doi.org/10.1016/j.eswa.2022.116845
Ashok A, Guruprasad M, Prakash CO, Shylaja SS (2019) A machine learning approach for disease surveillance and visualization using twitter data. In: ICCIDS 2019 - 2nd international conference on computational intelligence in data science, Proceedings, pp 1–6. https://doi.org/10.1109/ICCIDS.2019.8862087
Jahanbin K, Rahmanian V (2020) Using twitter and web news mining to predict covid-19 outbreak. Asian Pacific J Trop Med 13(8):378–380. https://doi.org/10.4103/1995-7645.279651
Liu D (2020) A machine learning methodology for real-time forecasting of the 2019-2020 COVID-19 outbreak using Internet searches , news alerts , and estimates from mechanistic models. http://arxiv.org/abs/2004.04019. no. d, Apr. 2020, [Online]
Belt TH (2018) Social media posts and online search behaviour as early-warning system for mrsa outbreaks. Antimicrob Resist Infect Control 7(1):1–10. https://doi.org/10.1186/s13756-018-0359-4
Liu D (2019) A dengue fever predicting model based on baidu search index data and climate data in south china. PLoS One. https://doi.org/10.1371/journal.pone.0226841
Yom-Tov E, Lampos V, Inns T, Cox IJ, Edelstein M (2022) Providing early indication of regional anomalies in covid-19 case counts in england using search engine queries. Sci Rep 12(1):1–10. https://doi.org/10.1038/s41598-022-06340-2
Liang F, Guan P, Wu W, Huang D (2018) Forecasting influenza epidemics by integrating internet search queries and traditional surveillance data with the support vector machine regression model in liaoning, from 2011 to 2015. PeerJ. https://doi.org/10.7717/peerj.5134
Li J, Sia CL, Chen Z, Huang W (2021) Enhancing influenza epidemics forecasting accuracy in china with both official and unofficial online news articles, 2019–2020. Int J Environ Res Public Health. https://doi.org/10.3390/ijerph18126591
Zhang Y, Ibaraki M, Schwartz FW (2019) Disease surveillance using online news: Dengue and zika in tropical countries. J Biomed Inform 102:103374. https://doi.org/10.1016/j.jbi.2020.103374
Hartigan A, Wong MA (1979) A k-means clustering algorithm. J R Stat Soc 28(1)
Collier N (2010) What’s unusual in online disease outbreak news? J Biomed Seman. https://doi.org/10.1186/2041-1480-1-2
Khan SA, Patel CO, Kukafka R (2006) Godsn: Global news driven disease outbreak and surveillance. In: AMIA ... Annual Symposium Proceedings / AMIA Symposium. AMIA Symposium, p 983
Mele I, Bahrainian SA, Crestani F (2019) Event mining and timeliness analysis from heterogeneous news streams. Inf Process Manag 56(3):969–993. https://doi.org/10.1016/j.ipm.2019.02.003
Goel R (2019) Epidnews: extracting, exploring and annotating news for monitoring animal diseases. J Comput Lang. https://doi.org/10.1016/j.cola.2019.100936
Ghosh S (2017) Temporal topic modeling to assess associations between news trends and infectious disease outbreaks. Sci Rep. https://doi.org/10.1038/srep40841
Lukandu IA, Tree D, Burden D, Surveillance D, Symptom D, Gain I (2021) An algorithm for notifiable disease modeling and prediction using artificial intelligence techniques
Nkiruka O, Prasad R, Clement O (2021) Prediction of malaria incidence using climate variability and machine learning. Inform Med Unlocked 22:100508. https://doi.org/10.1016/j.imu.2020.100508
Dansana D, Kumar R, Bhattacharjee A, Mahanty C (2022) Covid-19 outbreak prediction and analysis of e-healthcare data using random forest algorithms. Int J Reliab Qual E-Healthcare 11(1):1–13. https://doi.org/10.4018/IJRQEH.297075
Abdullahi T, Nitschke G, Sweijd N (2022) Predicting diarrhoea outbreaks with climate change. PLoS ONE 17(4):0262008. https://doi.org/10.1371/journal.pone.0262008
Zhao L, Chen F, Lu C-T, Ramakrishnan N (2016) Multi-resolution spatial event forecasting in social media. In: 2016 IEEE 16th International Conference on Data Mining (ICDM, pp 689–698. https://doi.org/10.1109/icdm.2016.0080.
Organization WH (2014) Early detection, assessment and response to acute public health events: Implementation of early warning and response with a focus on event-based surveillance. Who, 1–64
Keller M (2009) Use of Unstructured Event-Based Reports for Global Infectious Disease Surveillance. https://doi.org/10.3201/eid1505.081114
Mutuvi S, Doucet A, Lejeune G, Odeo M (2020) A dataset for multilingual epidemiological event extraction. LREC 2020 - 12th international conference on language resources and evaluation, conference proceedings (May), pp 4139–4144
Xiang WEI, Wang B (2019) A survey of event extraction from text. IEEE Access. https://doi.org/10.1109/ACCESS.2019.2956831
Lampos V, Zou B, Cox IJ (2017) Enhancing feature selection using word embeddings: The case of flu surveillance. 26th International World Wide Web Conference, WWW 2017 (Ili), pp 695–704. https://doi.org/10.1145/3038912.3052622
Yang CT (2020) Influenza-like illness prediction using a long short-term memory deep learning model with multiple open data sources. J Supercomput 76(12):9303–9329. https://doi.org/10.1007/s11227-020-03182-5
Darwish A, Rahhal Y, Jafar A (2020) A comparative study on predicting influenza outbreaks using different feature spaces: Application of influenza-like illness data from early warning alert and response system in syria. BMC Res Notes 13(1):1–8. https://doi.org/10.1186/s13104-020-4889-5
Wen Z, Powell G, Chafi I, Buckeridge DL, Li Y (2022) Inferring global-scale temporal latent topics from news reports to predict public health interventions for covid-19. Patterns. https://doi.org/10.1016/j.patter.2022.100435
Yan SJ, Chughtai AA, Macintyre CR (2017) Utility and potential of rapid epidemic intelligence from internet-based sources. Int J Infect Dis 63:77–87. https://doi.org/10.1016/j.ijid.2017.07.020
Lampos V (2022) Online searching trend on covid-19 using google trend: infodemiological study in malaysia. NPJ Digit Med 5(1):17. https://doi.org/10.1016/j.inpa.2022.03.004
Mandal S, Rath M, Wang Y, Patra BG (2018) Predicting zika prevention techniques discussed on twitter: An exploratory study. CHIIR 2018 - Proceedings of the 2018 Conference on Human Information Interaction and Retrieval, pp 269–272. https://doi.org/10.1145/3176349.3176874
Talvis K, Chorianopoulos K, Kermanidis KL (2014) Real-time monitoring of flu epidemics through linguistic and statistical analysis of twitter messages. In: Proceedings - 9th International Workshop on Semantic and Social Media Adaptation and Personalization, SMAP, pp 83–87. https://doi.org/10.1109/SMAP.2014.38
Carlos MA, Nogueira M, Machado RJ (2017): Analysis of dengue outbreaks using big data analytics and social networks. In: 2017 4th international conference on systems and informatics (ICSAI), pp 1592–1597
Zhang Y, Chen K, Weng Y, Chen Z, Zhang J, Hubbard R (2022) An intelligent early warning system of analyzing twitter data using machine learning on covid-19 surveillance in the us. Expert Syst Appl. https://doi.org/10.1016/j.eswa.2022.116882
Cheng IK, Heyl J, Lad N, Facini G, Grout Z (2021) Evaluation of twitter data for an emerging crisis: an application to the first wave of covid-19 in the uk. Sci Rep 11(1):1–13. https://doi.org/10.1038/s41598-021-98396-9
Wojcik S, Hughes A (2019) Sizing up twitter users. Jun 24
Bello-Orgaz G, Hernandez-Castro J, Camacho D (2015) A survey of social web mining applications for disease outbreak detection. Stud Comput Intell 570:345–356. https://doi.org/10.1007/978-3-319-10422-5_36
Download references
Acknowledgements
The first author would like to thank Naima Firdaus and Supriya Singh for their contribution toward understanding formatting.
The work did not receive any specific funding.
Author information
Authors and affiliations.
School of Computer and Systems Sciences, Jawaharlal Nehru University, JNU Ring Road, New Delhi, 110067, India
Avneet Singh Gautam & Zahid Raza
You can also search for this author in PubMed Google Scholar
Contributions
Avneet Singh Gautam contributed to literature search, conceptualization, methodology, study design, table design, validation, writing—original draft, and writing—review, and editing. Zahid Raza contributed to study design, supervision, validation, and writing—review and editing.
Corresponding author
Correspondence to Avneet Singh Gautam .
Ethics declarations
Conflict of interest.
The authors have no conflict of interest to declare.
Ethics approval
Additional information, publisher's note.
Springer Nature remains neutral with regard to jurisdictional claims in published maps and institutional affiliations.
Rights and permissions
Springer Nature or its licensor (e.g. a society or other partner) holds exclusive rights to this article under a publishing agreement with the author(s) or other rightsholder(s); author self-archiving of the accepted manuscript version of this article is solely governed by the terms of such publishing agreement and applicable law.
Reprints and permissions
About this article
Gautam, A.S., Raza, Z. Disease outbreak prediction using natural language processing: a review. Knowl Inf Syst (2024). https://doi.org/10.1007/s10115-024-02192-6
Download citation
Received : 04 November 2023
Revised : 30 June 2024
Accepted : 23 July 2024
Published : 06 August 2024
DOI : https://doi.org/10.1007/s10115-024-02192-6
Share this article
Anyone you share the following link with will be able to read this content:
Sorry, a shareable link is not currently available for this article.
Provided by the Springer Nature SharedIt content-sharing initiative
- Disease outbreak prediction
- Natural language processing
- Text analysis
- Machine learning
- Search data
- Twitter data
- Find a journal
- Publish with us
- Track your research
Thank you for visiting nature.com. You are using a browser version with limited support for CSS. To obtain the best experience, we recommend you use a more up to date browser (or turn off compatibility mode in Internet Explorer). In the meantime, to ensure continued support, we are displaying the site without styles and JavaScript.
- View all journals
- Explore content
- About the journal
- Publish with us
- Sign up for alerts
- Open access
- Published: 12 November 2020
Early and accurate detection and diagnosis of heart disease using intelligent computational model
- Yar Muhammad 1 ,
- Muhammad Tahir 1 ,
- Maqsood Hayat 1 &
- Kil To Chong 2
Scientific Reports volume 10 , Article number: 19747 ( 2020 ) Cite this article
27k Accesses
80 Citations
Metrics details
- Cardiovascular diseases
- Computational biology and bioinformatics
- Health care
- Heart failure
Heart disease is a fatal human disease, rapidly increases globally in both developed and undeveloped countries and consequently, causes death. Normally, in this disease, the heart fails to supply a sufficient amount of blood to other parts of the body in order to accomplish their normal functionalities. Early and on-time diagnosing of this problem is very essential for preventing patients from more damage and saving their lives. Among the conventional invasive-based techniques, angiography is considered to be the most well-known technique for diagnosing heart problems but it has some limitations. On the other hand, the non-invasive based methods, like intelligent learning-based computational techniques are found more upright and effectual for the heart disease diagnosis. Here, an intelligent computational predictive system is introduced for the identification and diagnosis of cardiac disease. In this study, various machine learning classification algorithms are investigated. In order to remove irrelevant and noisy data from extracted feature space, four distinct feature selection algorithms are applied and the results of each feature selection algorithm along with classifiers are analyzed. Several performance metrics namely: accuracy, sensitivity, specificity, AUC, F1-score, MCC, and ROC curve are used to observe the effectiveness and strength of the developed model. The classification rates of the developed system are examined on both full and optimal feature spaces, consequently, the performance of the developed model is boosted in case of high variated optimal feature space. In addition, P-value and Chi-square are also computed for the ET classifier along with each feature selection technique. It is anticipated that the proposed system will be useful and helpful for the physician to diagnose heart disease accurately and effectively.
Similar content being viewed by others
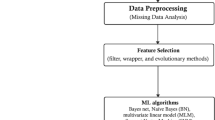

Analyzing the impact of feature selection methods on machine learning algorithms for heart disease prediction
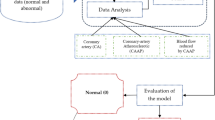
An active learning machine technique based prediction of cardiovascular heart disease from UCI-repository database
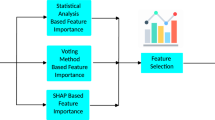
Finding the influential clinical traits that impact on the diagnosis of heart disease using statistical and machine-learning techniques
Introduction.
Heart disease is considered one of the most perilous and life snatching chronic diseases all over the world. In heart disease, normally the heart fails to supply sufficient blood to other parts of the body to accomplish their normal functionality 1 . Heart failure occurs due to blockage and narrowing of coronary arteries. Coronary arteries are responsible for the supply of blood to the heart itself 2 . A recent survey reveals that the United States is the most affected country by heart disease where the ratio of heart disease patients is very high 3 . The most common symptoms of heart disease include physical body weakness, shortness of breath, feet swollen, and weariness with associated signs, etc. 4 . The risk of heart disease may be increased by the lifestyle of a person like smoking, unhealthy diet, high cholesterol level, high blood pressure, deficiency of exercise and fitness, etc. 5 . Heart disease has several types in which coronary artery disease (CAD) is the common one that can lead to chest pain, stroke, and heart attack. The other types of heart disease include heart rhythm problems, congestive heart failure, congenital heart disease (birth time heart disease), and cardiovascular disease (CVD). Initially, traditional investigation techniques were used for the identification of heart disease, however, they were found complex 6 . Owing to the non-availability of medical diagnosing tools and medical experts specifically in undeveloped countries, diagnosis and cure of heart disease are very complex 7 . However, the precise and appropriate diagnosis of heart disease is very imperative to prevent the patient from more damage 8 . Heart disease is a fatal disease that rapidly increases in both economically developed and undeveloped countries. According to a report generated by the World Health Organization (WHO), an average of 17.90 million humans died from CVD in 2016. This amount represents approximately 30% of all global deaths. According to a report, 0.2 million people die from heart disease annually in Pakistan. Every year, the number of victimizing people is rapidly increasing. European Society of Cardiology (ESC) has published a report in which 26.5 million adults were identified having heart disease and 3.8 million were identified each year. About 50–55% of heart disease patients die within the initial 1–3 years, and the cost of heart disease treatment is about 4% of the overall healthcare annual budget 9 .
Conventional invasive-based methods used for the diagnosis of heart disease which were based on the medical history of a patient, physical test results, and investigation of related symptoms by the doctors 10 . Among the conventional methods, angiography is considered one of the most precise technique for the identification of heart problems. Conversely, angiography has some drawbacks like high cost, various side effects, and strong technological knowledge 11 . Conventional methods often lead to imprecise diagnosis and take more time due to human mistakes. In addition, it is a very expensive and computational intensive approach for the diagnosis of disease and takes time in assessment 12 .
To overcome the issues in conventional invasive-based methods for the identification of heart disease, researchers attempted to develop different non-invasive smart healthcare systems based on predictive machine learning techniques namely: Support Vector Machine (SVM), K-Nearest Neighbor (KNN), Naïve Bayes (NB), and Decision Tree (DT), etc. 13 . As a result, the death ratio of heart disease patients has been decreased 14 . In literature, the Cleveland heart disease dataset is extensively utilized by the researchers 15 , 16 .
In this regard, Robert et al . 17 have used a logistic regression classification algorithm for heart disease detection and obtained an accuracy of 77.1%. Similarly, Wankhade et al . 18 have used a multi-layer perceptron (MLP) classifier for heart disease diagnosis and attained accuracy of 80%. Likewise, Allahverdi et al . 19 have developed a heart disease classification system in which they integrated neural networks with an artificial neural network and attained an accuracy of 82.4%. In a sequel, Awang et al . 20 have used NB and DT for the diagnosis and prediction of heart disease and achieved reasonable results in terms of accuracy. They achieved an accuracy of 82.7% with NB and 80.4% with DT. Oyedodum and Olaniye 21 have proposed a three-phase system for the prediction of heart disease using ANN. Das and Turkoglu 22 have proposed an ANN ensemble-based predictive model for the prediction of heart disease. Similarly, Paul and Robin 23 have used the adaptive fuzzy ensemble method for the prediction of heart disease. Likewise, Tomov et al. 24 have introduced a deep neural network for heart disease prediction and his proposed model performed well and produced good outcomes. Further, Manogaran and Varatharajan 25 have introduced the concept of a hybrid recommendation system for diagnosing heart disease and their model has given considerable results. Alizadehsani et al . 26 have developed a non-invasive based model for the prediction of coronary artery disease and showed some good results regarding the accuracy and other performance assessment metrics. Amin et al . 27 have proposed a framework of a hybrid system for the identification of cardiac disease, using machine learning, and attained an accuracy of 86.0%. Similarly, Mohan et al . 28 have proposed another intelligent system that integrates RF with a linear model for the prediction of heart disease and achieved the classification accuracy of 88.7%. Likewise, Liaqat et al . 29 have developed an expert system that uses stacked SVM for the prediction of heart disease and obtained 91.11% classification accuracy on selected features.
The contribution of the current work is to introduce an intelligent medical decision system for the diagnosis of heart disease based on contemporary machine learning algorithms. In this study, 10 different nature of machine learning classification algorithms such as Logistic Regression (LR), Decision Tree (DT), Naïve Bayes (NB), Random Forest (RF), Artificial Neural Network (ANN), etc. are implemented in order to select the best model for timely and accurate detection of heart disease at an early stage. Four feature selection algorithms, Fast Correlation-Based Filter Solution (FCBF), minimal redundancy maximal relevance (mRMR), Least Absolute Shrinkage and Selection Operator (LASSO), and Relief have been used for selecting the vital and more correlated features that have truly reflect the motif of the desired target. Our developed system has been trained and tested on the Cleveland (S 1 ) and Hungarian (S 2 ) heart disease datasets which are available online on the UCI machine learning repository. All the processing and computations were performed using Anaconda IDE. Python has been used as a tool for implementing all the classifiers. The main packages and libraries used include pandas, NumPy, matplotlib, sci-kit learn (sklearn), and seaborn. The main contribution of our proposed work is given below:
The performance of all classifiers has been tested on full feature spaces in terms of all performance evaluation matrices specifically accuracy.
The performances of the classifiers are tested on selected feature spaces, selected through various feature selection algorithms mentioned above.
The research study recommends that which feature selection algorithm is feasible with which classification algorithm for developing a high-level intelligence system for the diagnosing of heart disease patients.
The rest of the paper is organized as: “ Results and discussion ” section represents the results and discussion, “ Material and methods ” section describes the material and methods used in this paper. Finally, we conclude our proposed research work in “ Conclusion ” section.
Results and discussion
This section of the paper discusses the experimental results of various contemporary classification algorithms. At first, the performance of all used classification models i.e. K-Nearest Neighbors (KNN), Decision Tree (DT), Extra-Tree Classifier (ETC), Random Forest (RF), Logistic Regression (LR), Naïve Bayes (NB), Artificial Neural Network (ANN), Support Vector Machine (SVM), Adaboost (AB), and Gradient Boosting (GB) along with full feature space is evaluated. After that, four feature selection algorithms (FSA): Fast Correlation-Based Filter (FCBF), Minimal Redundancy Maximal Relevance (mRMR), Least Absolute Shrinkage and Selection Operator (LASSO), and Relief are applied to select the prominent and high variant features from feature space. Furthermore, the selected feature spaces are provided to classification algorithms as input to analyze the significance of feature selection techniques. The cross-validation techniques i.e. k-fold (10-fold) are applied on both the full and selected feature spaces to analyze the generalization power of the proposed model. Various performance evaluation metrics are implemented for measuring the performances of the classification models.
Classifiers’ predictive outcomes on full feature space
The experimental outcomes of the applied classification algorithms on the full feature space of the two benchmark datasets by using 10-fold cross-validation (CV) techniques are shown in Tables 1 and 2 , respectively.
The experimental results demonstrated that the ET classifier performed quite well in terms of all performance evaluation metrics compared to the other classifiers using 10-fold CV. ET achieved 92.09% accuracy, 91.82% sensitivity, 92.38% specificity, 97.92% AUC, 92.84% Precision, 0.92 F1-Score and 0.84 MCC. The specificity indicates that the diagnosed test was negative and the individual doesn't have the disease. While the sensitivity indicates the diagnostic test was positive and the patient has heart disease. In the case of the KNN classification model, multiple experiments were accomplished by considering various values for k i.e. k = 3, 5, 7, 9, 13, and 15, respectively. Consequently, KNN has shown the best performance at value k = 7 and achieved a classification accuracy of 85.55%, 85.93% sensitivity, 85.17% specificity, 95.64% AUC, 86.09% Precision, 0.86 F1-Score, and 0.71 MCC. Similarly, DT classifier has achieved accuracy of 86.82%, 89.73% sensitivity, 83.76% specificity, 91.89% AUC, 85.40% Precision, 0.87 F1-Score, and 0.73 MCC. Likewise, GB classifier has yielded accuracy of 91.34%, 90.32% sensitivity, 91.52% specificity, 96.87% AUC, 92.14% Precision, 0.92 F1-Score, and 0.83 MCC. After empirically evaluating the success rates of all classifiers, it is observed that ET Classifier out-performed among all the used classification algorithms in terms of accuracy, sensitivity, and specificity. Whereas, NB shows the lowest performance in terms of accuracy, sensitivity, and specificity. The ROC curve of all classification algorithms on full feature space is represented in Fig. 1 .
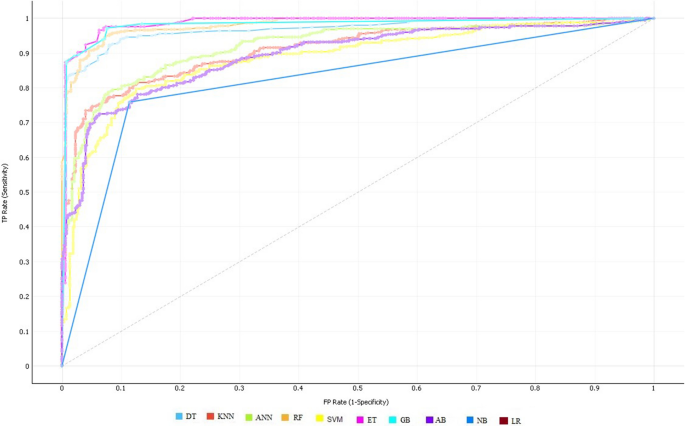
ROC curves of all classifiers on full feature space using 10-fold cross-validation on S 1 .
In the case of dataset S 2 , composed of 1025 total instances in which 525 belong to the positive class and 500 instances of having negative class, again ET has obtained quite well results compared to other classifiers using a 10-fold cross-validation test, which are 96.74% accuracy, 96.36 sensitivity, 97.40% specificity, and 0.93 MCC as shown in Table 2 .
Classifiers’ predictive outcomes on selected feature space
Fcbf feature selection technique.
FCBF feature selection technique is applied to select the best subset of feature space. In this attempt, various length of subspaces is generated and tested. Finally, the best results are achieved by classification algorithms on the subset of feature space (n = 6) using a 10-fold CV. Table 3 shows various performance measures of classifiers executed on the selected features space of FCBF.
Table 3 demonstrates that the ET classifier obtained quite good results including accuracy of 94.14%, 94.29% sensitivity, and specificity of 93.98%. In contrast, NB reported the lowest performance compared to the other classification algorithms. The performance of classification algorithms is also illustrated in Fig. 2 by using ROC curves.
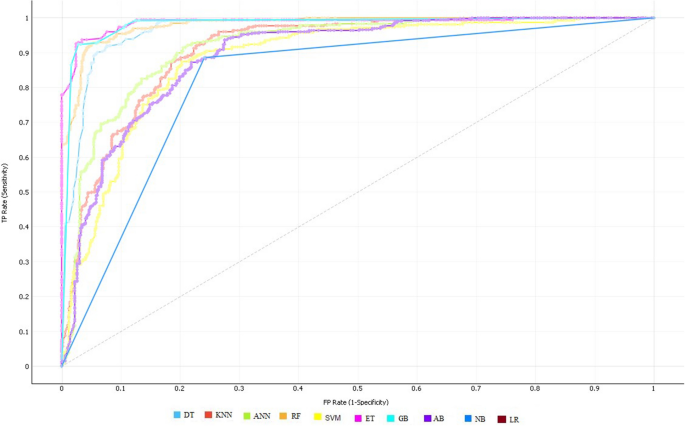
ROC curve of all classifiers on selected features by FCBF feature selection algorithm.
mRMR feature selection technique
mRMR feature selection technique is used in order to select a subset of features that enhance the performance of classifiers. The best results reported on a subset of n = 6 of feature space which is shown in Table 4 .
In the case of mRMR, still, the success rates of the ET classifier are well in terms of all performance evaluation metrics compared to the other classifiers. ET has attained 93.42% accuracy, 93.92% sensitivity, and specificity of 93.88%. In contrast, NB has achieved the lowest outcomes which are 81.84% accuracy. Figure 3 shows the ROC curve of all ten classifiers using the mRMR feature selection algorithm.
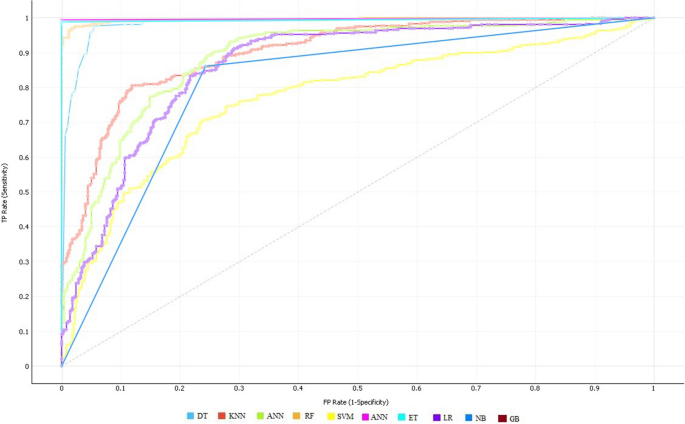
ROC curve of all classifiers on selected features using the mRMR feature selection algorithm.
LASSO feature selection technique
In order to choose the optimal feature space which not only reduces computational cost but also progresses the performance of the classifiers, LASSO feature selection technique is applied. After performing various experiments on different subsets of feature space, the best results are still noted on the subspace of (n = 6). The predicted outcomes of the best-selected feature space are reported in Table 5 using the 10-fold CV.
Table 5 demonstrated that the predicted outcomes of the ET classifier are considerable and better compared to the other classifiers. ET has achieved 89.36% accuracy, 88.21% sensitivity, and specificity of 90.58%. Likewise, GB has yielded the second-best result which is the accuracy of 88.47%, 89.54% sensitivity, and specificity of 87.37%. Whereas, LR has performed worse results and achieved 80.77% accuracy, 83.46% sensitivity, and specificity of 77.95%. ROC curves of the classifiers are shown in Fig. 4 .
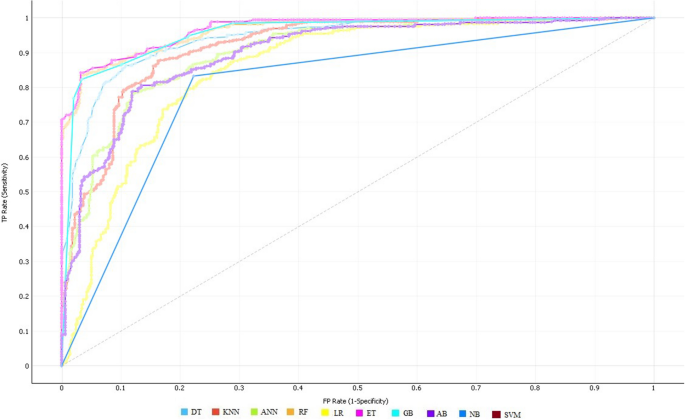
ROC curve of all classifiers on selected feature space using the LASSO feature selection algorithm.
Relief feature selection technique
In a sequel, another feature selection technique Relief is applied to investigate the performance of classifiers on different sub-feature spaces by using the wrapper method. After empirically analyzing the results of the classifiers on a different subset of feature spaces, it is observed that the performance of classifiers is outstanding on the sub-space of length (n = 6). The results of the optimal feature space on the 10-fold CV technique are listed in Table 6 .
Again, the ET classifier performed outstandingly in terms of all performance evaluation metrics as compared to other classifiers. ET has obtained an accuracy of 94.41%, 94.93% sensitivity, and specificity of 94.89%. In contrast, NB has shown the lowest performance and achieved 80.29% accuracy, 81.93% sensitivity, and specificity of 78.55%. The ROC curves of the classifiers are demonstrated in Fig. 5 .
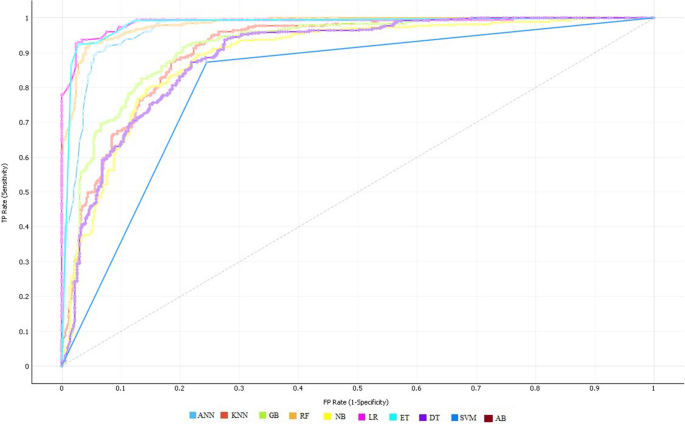
ROC curve of all classifiers on selected features selected by the Relief feature selection algorithm.
After executing classification algorithms along with full and selected feature spaces in order to select the optimal algorithm for the operational engine, the empirical results have revealed that ET performed well not only on all feature space but also on optimal selected feature space among all the used classification algorithms. Furthermore, the ET classifier obtained quite promising accuracy in the case of the Relief feature selection technique which is 94.41%. Overall, the performance of ET is reported better in terms of most of the measures while other classifiers have shown good results in one measure while worse in other measures. In addition, the performance of the ET classifier is also evaluated on a 10-fold CV in combination with different sub-feature spaces of varying length starting from 1 to 12 with a step size of 1 to check the stability and discrimination power of the classifier as described in 30 . Doing so will assist the readers to have a better understanding of the impact, of the number of selected features on the performance of the classifiers. The same process is repeated for another dataset i.e. S 2 (Hungarian heart disease dataset) as well, to know the impact of selected features on the classification performance.
Tables 7 and 8 shows the performance of the ET classifier using 10-fold CV in combination with different feature sub-spaces starting from 1 to 12 with a step size of 1. The experimental results show that the performance of the ET classifier is affected significantly by using the varying length of sub-feature spaces. Finally, it is concluded that all these achievements are ascribed with the best selection of Relief feature selection technique which not only reduces the feature space but also enhances the predictive power of classifiers. In addition, the ET classifier has also played a quite promising role in these achievements because it has clearly and precisely learned the motif of the target class and reflected it truly. In addition, the performance of the ET classifier is also evaluated on 5-fold and 7-fold CV in combination with different sub-spaces of length 5 and 7 to check the stability and discrimination power of the classifier. It is also tested on another dataset S 2 (Hungarian heart disease dataset). The results are shown in supplementary materials .
In Table 9 , P-value and Chi-Square values are also computed for the ET classifier in combination with the optimal feature spaces of different feature selection techniques.
Performance comparison with existing models
Further, a comparative study of the developed system is conducted with other states of the art machine learning approaches discussed in the literature. Table 10 represents, a brief description and classification accuracies of those approaches. The results demonstrate that our proposed model success rate is high compared to existing models in the literature.
Material and methods
The subsections represent the materials and the methods that are used in this paper.
The first and rudimentary step of developing an intelligent computational model is to construct or develop a problem-related dataset that truly and effectively reflects the pattern of the target class. Well organized and problem-related dataset has a high influence on the performance of the computational model. Looking at the significance of the dataset, two datasets i.e. the Cleveland heart disease dataset S 1 and Hungarian heart disease dataset (S 2 ) are used, which are available online at the University of California Irvine (UCI) machine learning repository and UCI Kaggle repository, and various researchers have used it for conducting their research studies 28 , 31 , 32 . The S1 consists of 304 instances, where each instance has distinct 13 attributes along with the target labels and are selected for training. The dataset is composed of two classes, presence or absence of heart disease. The S 2 is composed of 1025 instances in which 525 instances belong to positive class while the rest of 500 instances have negative class. The description of attributes of both the datasets is the same, and both have similar attributes. The complete description and information of the datasets with 13 attributes are given in Table 11 .
Proposed system methodology
The main theme of the developed system is to identify heart problems in human beings. In this study, four distant feature selection techniques namely: FCBF, mRMR, Relief, and LASSO are applied on the provided dataset in order to remove noisy, redundant features and select variant features, consequently may cause of enhancing the performance of the proposed model. Various machine learning classification algorithms are used in this study which include, KNN, DT, ETC, RF, LR, NB, ANN, SVM, AB, and GB. Different evaluation metrics are computed to assess the performance of classification algorithms. The methodology of the proposed system is carried out in five stages which include dataset preprocessing, selection of features, cross-validation technique, classification algorithms, and performance evaluation of classifiers. The framework of the proposed system is illustrated in Fig. 6 .
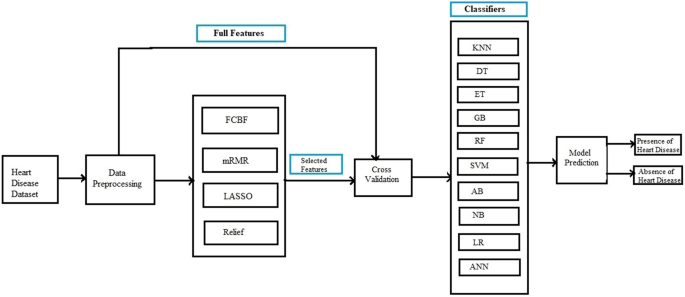
An Intelligent Hybrid Framework for the prediction of heart disease.
Preprocessing of data
Data preprocessing is the process of transforming raw data into meaningful patterns. It is very crucial for a good representation of data. Various preprocessing approaches such as missing values removal, standard scalar, and Min–Max scalar are used on the dataset in order to make it more effective for classification.
Feature selection algorithms
Feature selection technique selects the optimal features sub-space among all the features in a dataset. It is very crucial because sometimes, the classification performance degrades due to irrelevant features in the dataset. The feature selection technique improves the performance of classification algorithms and also reduces their execution time. In this research study, four feature selection techniques are used and are listed below:
Fast correlation-based filter (FCBF): FCBF feature selection algorithm follows a sequential search strategy. It first selects full features and then uses symmetric uncertainty for measuring the dependencies of the features on each other and how they affect the target output label. After this, it selects the most important features using the backward sequential search strategy. FCBF outperforms on high dimensional datasets. Table 12 shows the results of the selected features (n = 6) by using the FCBF feature selection algorithm. Each attribute is given a weight based on its importance. According to the FCBF feature selection technique, the most important features are THA and CPT as shown in Table 12 . The ranking that the FCBF gives to all the features of the dataset is shown in Fig. 7 .
Minimal redundancy maximal relevance (mRMR): mRMR uses the heuristic approach for selecting the most vital features that have minimum redundancy and maximum relevance. It selects those features which are useful and relevant to the target. As it follows a heuristic approach so, it checks one feature at a time and then computes its pairwise redundancy with the other features. The mRMR feature selection algorithm is not suitable for high domain feature problems 33 . The results of selected features by the mRMR feature selection algorithm (n = 6) are listed in Table 13 . In addition, among these attributes, PES and CPT have the highest score. Figure 7 describes the attributes ranking given by the mRMR feature selection algorithm to all attributes in the feature space.
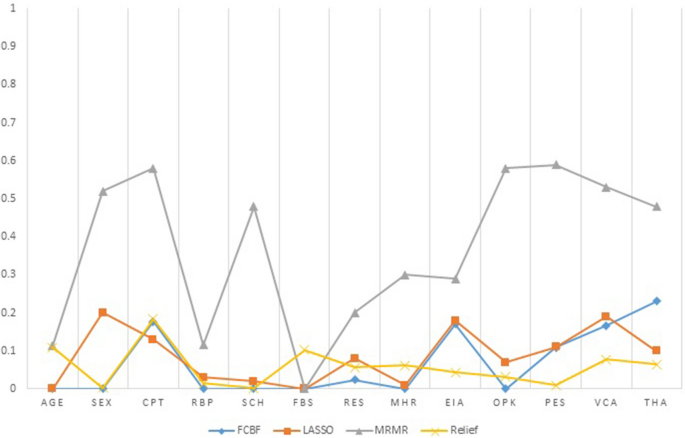
Features ranking by four feature selection algorithms (FCBF, LASSO, mRMR, Relief).
Least absolute shrinkage and selection operator (LASSO) LASSO selects features based on updating the absolute value of the features coefficient. In updating the features coefficient values, zero becoming values are removed from the features subset. LASSO outperforms with low feature coefficient values. The features having high coefficient values will be selected in the subset of features and the rest will be eliminated. Moreover, some irrelevant features with higher coefficient values may be selected and are included in the subset of features 30 . Table 14 represents the six most profound attributes which have a great correlation with the target and their scores selected by the LASSO feature selection algorithm. Figure 7 represents the important features and their scoring values given by the LASSO feature selection algorithm.
Relief feature selection algorithm Relief utilizes the concept of instance-based learning which allocates weight to each attribute based on its significance. The weight of each attribute demonstrates its capability to differentiate among class values. Attributes are rated by weights, and those attributes whose weight is exceeding a user-specified cutoff, are chosen as the final subset 34 . The relief feature selection algorithm selects the most significant attributes which have more effect on the target 35 . The algorithm operates by selecting instances randomly from the training samples. The nearest instance of the same class (nearest hit) and opposite class (nearest miss) is identified for each sampled instance. The weight of an attribute is updated according to how well its values differentiate between the sampled instance and its nearest miss and hit. If an attribute discriminates amongst instances from different classes and has the same value for instances of the same class, it will get a high weight.
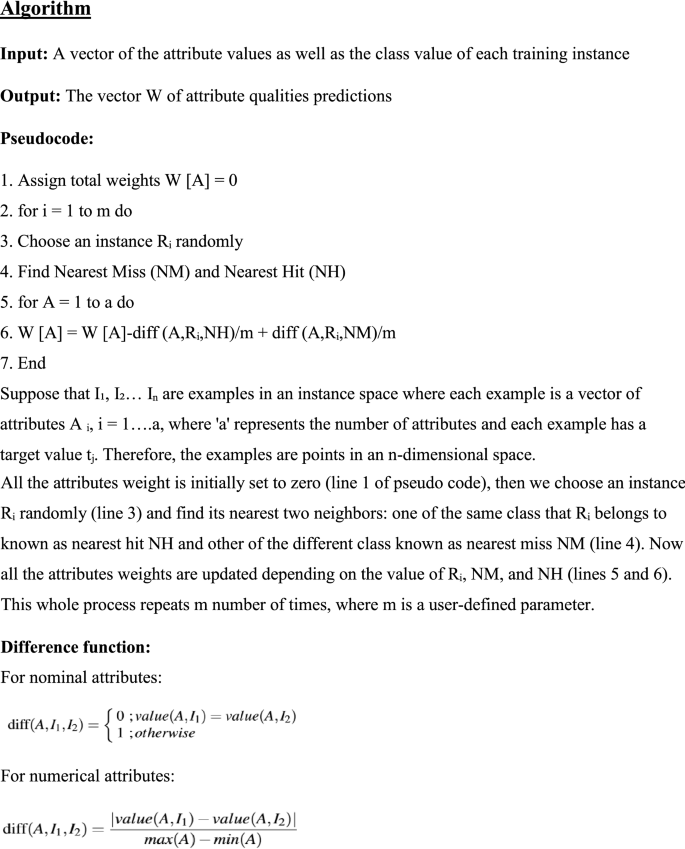
The weight updating of attributes works on a simple idea (line 6). That if instance R i and NH have dissimilar value (i.e. the diff value is large), that means the attribute splits two instances with the same class which is not worthwhile, and thus we reduce the attributes weight. On the other hand, if the instance R i and NM have a distinct value that means the attribute separates the two instances with a different class, which is desirable. The six most important features selected by the Relief algorithm are listed in descending order in Table 15 . Based on weight values the most vital features are CPT and Age. Figure 7 demonstrates the important features and their ranking given by the Relief feature selection algorithm.
Machine learning classification algorithms
Various machine learning classification algorithms are investigated for early detection of heart disease, in this study. Each classification algorithm has its significance and the importance is reported varied from application to application. In this paper, 10 distant nature of classification algorithms namely: KNN, DT, ET, GB, RF, SVM, AB, NB, LR, and ANN are applied to select the best and generalize prediction model.
Classifier validation method
Validation of the prediction model is an essential step in machine learning processes. In this paper, the K-Fold cross-validation method is applied to validating the results of the above-mentioned classification models.
K-fold cross validation (CV)
In K-Fold CV, the whole dataset is split into k equal parts. The (k-1) parts are utilized for training and the rest is used for the testing at each iteration. This process continues for k-iteration. Various researchers have used different values of k for CV. Here k = 10 is used for experimental work because it produces good results. In tenfold CV, 90% of data is utilized for training the model and the remaining 10% of data is used for the testing of the model at each iteration. At last, the mean of the results of each step is taken which is the final result.
Performance evaluation metrics
For measuring the performance of the classification algorithms used in this paper, various evaluation matrices have been implemented including accuracy, sensitivity, specificity, f1-score, recall, Mathew Correlation-coefficient (MCC), AUC-score, and ROC curve. All these measures are calculated from the confusion matrix described in Table 16 .
In confusion matrix True Negative (TN) shows that the patient has not heart disease and the model also predicts the same i.e. a healthy person is correctly classified by the model.
True Positive (TP) represents that the patient has heart disease and the model also predicts the same result i.e. a person having heart disease is correctly classified by the model.
False Positive (FP) demonstrates that the patient has not heart disease but the model predicted that the patient has i.e. a healthy person is incorrectly classified by the model. This is also called a type-1 error.
False Negative (FN) notifies that the patient has heart disease but the model predicted that the patient has not i.e. a person having heart disease is incorrectly classified by the model. This is also called a type-2 error.
Accuracy Accuracy of the classification model shows the overall performance of the model and can be calculated by the formula given below:
Specificity specificity is a ratio of the recently classified healthy people to the total number of healthy people. It means the prediction is negative and the person is healthy. The formula for calculating specificity is given as follows:
Sensitivity Sensitivity is the ratio of recently classified heart patients to the total patients having heart disease. It means the model prediction is positive and the person has heart disease. The formula for calculating sensitivity is given below:
Precision: Precision is the ratio of the actual positive score and the positive score predicted by the classification model/algorithm. Precision can be calculated by the following formula:
F1-score F1 is the weighted measure of both recall precision and sensitivity. Its value ranges between 0 and 1. If its value is one then it means the good performance of the classification algorithm and if its value is 0 then it means the bad performance of the classification algorithm.
MCC It is a correlation coefficient between the actual and predicted results. MCC gives resulting values between − 1 and + 1. Where − 1 represents the completely wrong prediction of the classifier.0 means that the classifier generates random prediction and + 1 represents the ideal prediction of the classification models. The formula for calculating MCC values is given below:
Finally, we will examine the predictability of the machine learning classification algorithms with the help of the receiver optimistic curve (ROC) which represents a graphical demonstration of the performance of ML classifiers. The area under the curve (AUC) describes the ROC of a classifier and the performance of the classification algorithms is directly linked with AUC i.e. larger the value of AUC greater will be the performance of the classification algorithm.
In this study, 10 different machine learning classification algorithms namely: LR, DT, NB, RF, ANN, KNN, GB, SVM, AB, and ET are implemented in order to select the best model for early and accurate detection of heart disease. Four feature selection algorithms such as FCBF, mRMR, LASSO, and Relief have been used to select the most vital and correlated features that truly reflect the motif of the desired target. Our developed intelligent computational model has been trained and tested on two datasets i.e. Cleveland (S1) and Hungarian (S2) heart disease datasets. Python has been used as a tool for implementation and simulating the results of all the utilized classification algorithms.
The performance of all classification models has been tested in terms of various performance metrics on full feature space as well as selected feature spaces, selected through various feature selection algorithms. This research study recommends that which feature selection algorithm is feasible with which classification model for developing a high-level intelligent system for the diagnosis of a patient having heart disease. From simulation results, it is observed that ET is the best classifier while relief is the optimal feature selection algorithm. In addition, P-value and Chi-square are also computed for the ET classifier along with each feature selection algorithm. It is anticipated that the proposed system will be useful and helpful for the doctors and other care-givers to diagnose a patient having heart disease accurately and effectively at the early stages.
Heart disease is one of the most devastating and fatal chronic diseases that rapidly increase in both economically developed and undeveloped countries and causes death. This damage can be reduced considerably if the patient is diagnosed in the early stages and proper treatment is provided to her. In this paper, we developed an intelligent predictive system based on contemporary machine learning algorithms for the prediction and diagnosis of heart disease. The developed system was checked on two datasets i.e. Cleveland (S1) and Hungarian (S2) heart disease datasets. The developed system was trained and tested on full features and optimal features as well. Ten classification algorithms including, KNN, DT, RF, NB, SVM, AB, ET, GB, LR, and ANN, and four feature selection algorithms such as FCBF, mRMR, LASSO, and Relief are used. The feature selection algorithm selects the most significant features from the feature space, which not only reduces the classification errors but also shrink the feature space. To assess the performance of classification algorithms various performance evaluation metrics were used such as accuracy, sensitivity, specificity, AUC, F1-score, MCC, and ROC curve. The classification accuracies of the top two classification algorithms i.e. ET and GB on full features were 92.09% and 91.34% respectively. After applying feature selection algorithms, the classification accuracy of ET with the relief feature selection algorithm increases from 92.09 to 94.41%. The accuracy of GB increases from 91.34 to 93.36% with the FCBF feature selection algorithm. So, the ET classifier with the relief feature selection algorithm performs excellently. P-value and Chi-square are also computed for the ET classifier with each feature selection technique. The future work of this research study is to use more optimization techniques, feature selection algorithms, and classification algorithms to improve the performance of the predictive system for the diagnosis of heart disease.
Bui, A. L., Horwich, T. B. & Fonarow, G. C. Epidemiology and risk profile of heart failure. Nat. Rev. Cardiol. 8 , 30 (2011).
Article PubMed Google Scholar
Polat, K. & Güneş, S. Artificial immune recognition system with fuzzy resource allocation mechanism classifier, principal component analysis, and FFT method based new hybrid automated identification system for classification of EEG signals. Expert Syst. Appl. 34 , 2039–2048 (2010).
Article Google Scholar
Heidenreich, P. A. et al. Forecasting the future of cardiovascular disease in the United States: A policy statement from the American Heart Association. Circulation 123 , 933–944 (2011).
Durairaj, M. & Ramasamy, N. A comparison of the perceptive approaches for preprocessing the data set for predicting fertility success rate. Int. J. Control Theory Appl. 9 , 255–260 (2016).
Google Scholar
Das, R., Turkoglu, I. & Sengur, A. Effective diagnosis of heart disease through neural networks ensembles. Expert Syst. Appl. 36 , 7675–7680 (2012).
Allen, L. A. et al. Decision making in advanced heart failure: A scientific statement from the American Heart Association. Circulation 125 , 1928–1952 (2014).
Yang, H. & Garibaldi, J. M. A hybrid model for automatic identification of risk factors for heart disease. J. Biomed. Inform. 58 , S171–S182 (2015).
Article PubMed PubMed Central Google Scholar
Alizadehsani, R., Hosseini, M. J., Sani, Z. A., Ghandeharioun, A. & Boghrati, R. In 2012 IEEE 12th International Conference on Data Mining Workshops. 9–16 (IEEE, New York).
Arabasadi, Z., Alizadehsani, R., Roshanzamir, M., Moosaei, H. & Yarifard, A. A. Computer aided decision making for heart disease detection using hybrid neural network-Genetic algorithm. Comput. Methods Programs Biomed. 141 , 19–26 (2017).
Samuel, O. W., Asogbon, G. M., Sangaiah, A. K., Fang, P. & Li, G. An integrated decision support system based on ANN and Fuzzy_AHP for heart failure risk prediction. Expert Syst. Appl. 68 , 163–172 (2017).
Patil, S. B. & Kumaraswamy, Y. Intelligent and effective heart attack prediction system using data mining and artificial neural network. Eur. J. Sci. Res. 31 , 642–656 (2009).
Vanisree, K. & Singaraju, J. Decision support system for congenital heart disease diagnosis based on signs and symptoms using neural networks. Int. J. Comput. Appl. 19 , 6–12 (2015).
B. Edmonds. In Proceedings of AISB Symposium on Socially Inspired Computing 1–12 (Hatfield, 2005).
Methaila, A., Kansal, P., Arya, H. & Kumar, P. Early heart disease prediction using data mining techniques. Comput. Sci. Inf. Technol. J. https://doi.org/10.5121/csit.2014.4807 (2014).
Samuel, O. W., Asogbon, G. M., Sangaiah, A. K., Fang, P. & Li, G. An integrated decision support system based on ANN and Fuzzy_AHP for heart failure risk prediction. Expert Syst. Appl. 68 , 163–172 (2018).
Nazir, S., Shahzad, S., Mahfooz, S. & Nazir, M. Fuzzy logic based decision support system for component security evaluation. Int. Arab J. Inf. Technol. 15 , 224–231 (2018).
Detrano, R. et al. International application of a new probability algorithm for the diagnosis of coronary artery disease. Am. J. Cardiol. 64 , 304–310 (2009).
Gudadhe, M., Wankhade, K. & Dongre, S. In 2010 International Conference on Computer and Communication Technology (ICCCT) , 741–745 (IEEE, New York).
Kahramanli, H. & Allahverdi, N. Design of a hybrid system for the diabetes and heart diseases. Expert Syst. Appl. 35 , 82–89 (2013).
Palaniappan, S. & Awang, R. In 2012 IEEE/ACS International Conference on Computer Systems and Applications 108–115 (IEEE, New York).
Olaniyi, E. O., Oyedotun, O. K. & Adnan, K. Heart diseases diagnosis using neural networks arbitration. Int. J. Intel. Syst. Appl. 7 , 72 (2015).
Das, R., Turkoglu, I. & Sengur, A. Effective diagnosis of heart disease through neural networks ensembles. Expert Syst. Appl. 36 , 7675–7680 (2011).
Paul, A. K., Shill, P. C., Rabin, M. R. I. & Murase, K. Adaptive weighted fuzzy rule-based system for the risk level assessment of heart disease. Applied Intelligence 48 , 1739–1756 (2018).
Tomov, N.-S. & Tomov, S. On deep neural networks for detecting heart disease. arXiv:1808.07168 (2018).
Manogaran, G., Varatharajan, R. & Priyan, M. Hybrid recommendation system for heart disease diagnosis based on multiple kernel learning with adaptive neuro-fuzzy inference system. Multimedia Tools Appl. 77 , 4379–4399 (2018).
Alizadehsani, R. et al. Non-invasive detection of coronary artery disease in high-risk patients based on the stenosis prediction of separate coronary arteries. Comput. Methods Programs Biomed. 162 , 119–127 (2018).
Haq, A. U., Li, J. P., Memon, M. H., Nazir, S. & Sun, R. A hybrid intelligent system framework for the prediction of heart disease using machine learning algorithms. Mobile Inf. Syst. 2018 , 3860146. https://doi.org/10.1155/2018/3860146 (2018).
Mohan, S., Thirumalai, C. & Srivastava, G. Effective heart disease prediction using hybrid machine learning techniques. IEEE Access 7 , 81542–81554 (2019).
Ali, L. et al. An optimized stacked support vector machines based expert system for the effective prediction of heart failure. IEEE Access 7 , 54007–54014 (2019).
Peng, H., Long, F. & Ding, C. Feature selection based on mutual information criteria of max-dependency, max-relevance, and min-redundancy. IEEE Trans. Pattern Anal. Mach. Intell. 27 (8), 1226–1238 (2005).
Palaniappan, S. & Awang, R. In 2008 IEEE/ACS International Conference on Computer Systems and Applications 108–115 (IEEE, New York).
Ali, L., Niamat, A., Golilarz, N. A., Ali, A. & Xingzhong, X. An expert system based on optimized stacked support vector machines for effective diagnosis of heart disease. IEEE Access (2019).
Pérez, N. P., López, M. A. G., Silva, A. & Ramos, I. Improving the Mann-Whitney statistical test for feature selection: An approach in breast cancer diagnosis on mammography. Artif. Intell. Med. 63 , 19–31 (2015).
Tibshirani, R. Regression shrinkage and selection via the lasso: A retrospective. J. R. Stat. Soc. Ser. B Stat. Methodol. 73 , 273–282 (2011).
Article MathSciNet Google Scholar
Peng, H., Long, F. & Ding, C. Feature selection based on mutual information criteria of max-dependency, max-relevance, and min-redundancy. IEEE Trans. Pattern Anal. Mach. Intell. 27 , 1226–1238 (2012).
de Silva, A. M. & Leong, P. H. Grammar-Based Feature Generation for Time-Series Prediction (Springer, Berlin, 2015).
Book Google Scholar
Download references
Acknowledgements
This research was supported by the Brain Research Program of the National Research Foundation (NRF) funded by the Korean government (MSIT) (No. NRF-2017M3C7A1044815).
Author information
Authors and affiliations.
Department of Computer Science, Abdul Wali Khan University Mardan, Mardan, 23200, KP, Pakistan
Yar Muhammad, Muhammad Tahir & Maqsood Hayat
Department of Electronic and Information Engineering, Jeonbuk National University, Jeonju, 54896, South Korea
Kil To Chong
You can also search for this author in PubMed Google Scholar
Contributions
All authors have equal contributions.
Corresponding authors
Correspondence to Maqsood Hayat or Kil To Chong .
Ethics declarations
Competing interests.
The authors declare no competing interests.
Additional information
Publisher's note.
Springer Nature remains neutral with regard to jurisdictional claims in published maps and institutional affiliations.
Supplementary information
Supplementary information., rights and permissions.
Open Access This article is licensed under a Creative Commons Attribution 4.0 International License, which permits use, sharing, adaptation, distribution and reproduction in any medium or format, as long as you give appropriate credit to the original author(s) and the source, provide a link to the Creative Commons licence, and indicate if changes were made. The images or other third party material in this article are included in the article's Creative Commons licence, unless indicated otherwise in a credit line to the material. If material is not included in the article's Creative Commons licence and your intended use is not permitted by statutory regulation or exceeds the permitted use, you will need to obtain permission directly from the copyright holder. To view a copy of this licence, visit http://creativecommons.org/licenses/by/4.0/ .
Reprints and permissions
About this article
Cite this article.
Muhammad, Y., Tahir, M., Hayat, M. et al. Early and accurate detection and diagnosis of heart disease using intelligent computational model. Sci Rep 10 , 19747 (2020). https://doi.org/10.1038/s41598-020-76635-9
Download citation
Received : 03 April 2020
Accepted : 28 October 2020
Published : 12 November 2020
DOI : https://doi.org/10.1038/s41598-020-76635-9
Share this article
Anyone you share the following link with will be able to read this content:
Sorry, a shareable link is not currently available for this article.
Provided by the Springer Nature SharedIt content-sharing initiative
This article is cited by
Comprehensive evaluation and performance analysis of machine learning in heart disease prediction.
- Halah A. Al-Alshaikh
- Abeer A. AlSanad
Scientific Reports (2024)
Future prediction for precautionary measures associated with heart-related issues based on IoT prototype
- Ganesh Keshaorao Yenurkar
- Aniket Pathade
Multimedia Tools and Applications (2024)
An improved machine learning-based prediction framework for early detection of events in heart failure patients using mHealth
- Deepak Kumar
- Keerthiveena Balraj
- Anurag S. Rathore
Health and Technology (2024)
Identification and classification of pneumonia disease using a deep learning-based intelligent computational framework
- Lanying Tang
Neural Computing and Applications (2023)
Back propagation artificial neural network for diagnose of the heart disease
- Jagmohan Kaur
- Baljit S. Khehra
- Amarinder Singh
Journal of Reliable Intelligent Environments (2023)
By submitting a comment you agree to abide by our Terms and Community Guidelines . If you find something abusive or that does not comply with our terms or guidelines please flag it as inappropriate.
Quick links
- Explore articles by subject
- Guide to authors
- Editorial policies
Sign up for the Nature Briefing newsletter — what matters in science, free to your inbox daily.

Disease Prediction System using Support Vector Machine and Multilinear Regression
International Journal of Innovative Research in Computer Science & Technology (IJIRCST) ISSN: 2347-5552, Volume, 8, Issue, 4, July, 2020
6 Pages Posted: 30 Sep 2020
Md. Ehtisham Farooqui
Integral University
Dr. Jameel Ahmad
Date Written: August 13, 2020
Evolution of modern technologies like data science and machine learning has opened the path for healthcare communities and medical institutions, to detect the diseases earliest as possible and it helps to provide better patient care. Accuracy of detecting the possible diseases is reduced when we do not have complete medical data. Furthermore, certain diseases are region-based, which might cause weak disease prediction. Our body shows the symptoms when something wrong is happening within our body, sometime it may be just minor problem but sometimes we can have severe illness and if we do not take care of these symptoms at the early stage then it might be too late to cure the disease. So we are proposing a disease prediction system that can predict the possible diseases based on symptoms so it can be cured at the early stage. It saves time that is required to do the complete diagnosis of the patient and based on the suggestions provided by the system we can only get the patient diagnosed for those diseases that are required. In this paper, we are using machine learning algorithms that try to accurately predict possible diseases. The results generated by the proposed system have an accuracy of up to 87%. The system has incredible potential in anticipating the possible diseases more precisely. The main motive of this study is to help the nontechnical person and freshman doctors to make a correct opinion about the diseases.
Keywords: Disease Prediction System, Machine Learning, Multilinear Regression (MLR), Support Vector Machine (SVM)
Suggested Citation: Suggested Citation
Md. Ehtisham Farooqui (Contact Author)
Integral university ( email ).
Basha Dasauli Kursi Road Lucknow, Uttar Pradesh 226026 India
Do you have a job opening that you would like to promote on SSRN?
Paper statistics, related ejournals, artificial intelligence ejournal.
Subscribe to this fee journal for more curated articles on this topic
Innovation & Geography eJournal
Computational biology ejournal.
IEEE Account
- Change Username/Password
- Update Address
Purchase Details
- Payment Options
- Order History
- View Purchased Documents
Profile Information
- Communications Preferences
- Profession and Education
- Technical Interests
- US & Canada: +1 800 678 4333
- Worldwide: +1 732 981 0060
- Contact & Support
- About IEEE Xplore
- Accessibility
- Terms of Use
- Nondiscrimination Policy
- Privacy & Opting Out of Cookies
A not-for-profit organization, IEEE is the world's largest technical professional organization dedicated to advancing technology for the benefit of humanity. © Copyright 2024 IEEE - All rights reserved. Use of this web site signifies your agreement to the terms and conditions.
Heart disease disorder prediction using electrocardiogram signals and machine learning
- Gupta, Ayush
- Kumar, C. Ashok
Heart disease is quickly becoming one of the most critical and widespread health concerns in the globe at the present time. At this time, a great deal of research is being conducted in an effort to treat a variety of cardiac conditions and a great number of lives. Because it enables medical practitioners to begin treating patients as soon as possible, early diagnosis of any heart illness is critical for this purpose. The purpose of this research is to develop a method that is capable of detecting coronary disease even before the onset of symptoms. After acquiring a data set consisting of highly processed ECG signal levels and other parameters that are required for the diagnosis of a variety of heart-related conditions, anomalies and outliers were eliminated from the data. One part of the dataset consisted of inputs classified as a category, whereas the other part consisted of inputs classified as binary. After that, we used the Keras framework to construct a deep learning model with three layers, and then we fed it the data set. The accuracy of the model with the binary data set was 93.89 percent, which is satisfactory given the modification made to this data set to include cancer cases in adults aged 18 to 77. In addition to the artificial neural network, a K-Fold Cross Validation model was developed and data was input into it. The accuracy of this model in diagnosing heart illness was 91.6 percent, which is quite good and helps in recognizing anomalies. This model also had a high success rate in identifying heart disease. As a result of advancements in technology, we are now in a position to diagnose illnesses and abnormalities of the heart in a greater population with greater precision
- ENGINEERING AND TECHNOLOGY
- Multiple chronic conditions
Multi Disease Detection and Predictions Based On Machine Learning
- February 2020
- SSRN Electronic Journal 7(2):950-953
- 7(2):950-953

- College of Engineering, Pune
- This person is not on ResearchGate, or hasn't claimed this research yet.
Discover the world's research
- 25+ million members
- 160+ million publication pages
- 2.3+ billion citations
- IJSREM JOURNAL
- M Kalaivani
- Shreenagamanjula Rani
- D. Roja Ramani

- S.A. Pattekari
- Dhanesh D Deshpande
- Rahul P Lokhande
- Juilee M Mundhe
- Abbas Khosravi
- , Syed Moshfeq Salaken
- Amin Khatami
- Saeid Nahavandi
- N G Bhuvaneswari Amma
- G Annapoorani
- Recruit researchers
- Join for free
- Login Email Tip: Most researchers use their institutional email address as their ResearchGate login Password Forgot password? Keep me logged in Log in or Continue with Google Welcome back! Please log in. Email · Hint Tip: Most researchers use their institutional email address as their ResearchGate login Password Forgot password? Keep me logged in Log in or Continue with Google No account? Sign up
Academia.edu no longer supports Internet Explorer.
To browse Academia.edu and the wider internet faster and more securely, please take a few seconds to upgrade your browser .
Enter the email address you signed up with and we'll email you a reset link.
- We're Hiring!
- Help Center
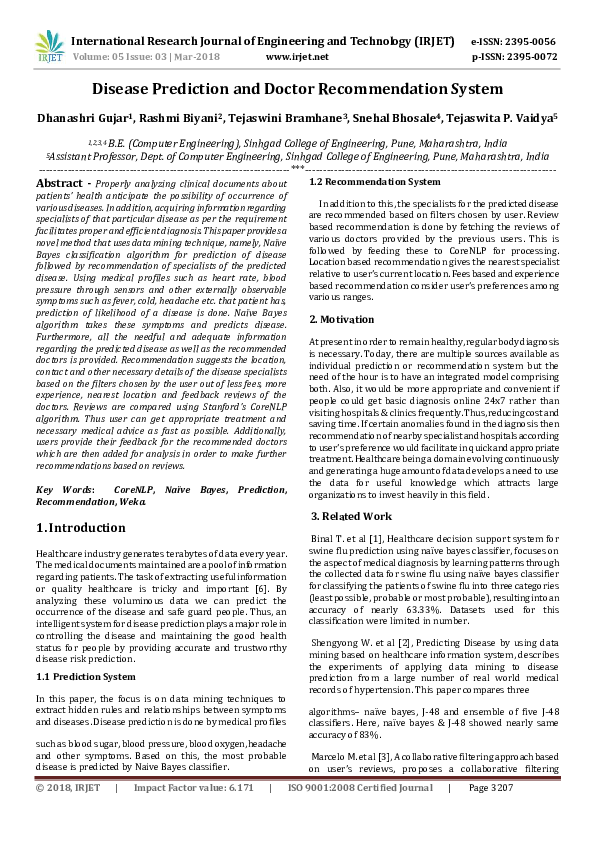
Disease Prediction and Doctor Recommendation System

https://irjet.net/archives/V5/i3/IRJET-V5I3756.pdf
Related Papers
Dr.SHASHI DHAR V , Pradyumna Kubear
Sufferer fulfilment has become an important measurement for keeping an eye on health maintenance and gig of convalescent homes. This shape has thrived into a new feature: the perspective of the sufferer's side of egis. Currently data stored in medical Database is growing rapidly. Analysing the data is important for medical decision making. It is extensively recognized that medical data analysis promotes well care by improving sufferer gig. This shape has thrived into a new feature: the perspective of the sufferer's side of egis. Currently, data is stored in the form of medical Database is growing rapidly. Analysing the datum is important for medical decision making. It is extensively recognized that medical data analysis promotes well care by improving sufferer direction gig. Sufferer length is the most commonly used outcome quantify for monitoring convalescent homes resource utilization and convalescent home show. It helps to manage the kitty and pronouncement fittingly.
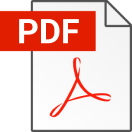
IRJET Journal
Nowadays people are progressively started caring about the health and medical diagnosis problems. However, according to the administration's report, more than 1 crore people every year die due to medication error done by novices(New doctor's). More than 42% medication errors are caused by doctors because they provide prescriptions according to their experience which are quite limited. And sometimes they have left many parts of the book they have read Technologies as data mining and recommender technologies provide possibilities to explore potential knowledge from diagnosis history and help the doctors to prescribe medication correctly to decrease the medication error. In this recommendation we will design and implement a universal medicine recommender system framework that applies data mining technologies to the recommendation system. The medicine recommender system consists of database system module, recommendation model module, model evaluation, and data visualization module. We investigated different medicine recommendation algorithms which are generally used in recommendation system SVM (Support Vector Machine), BP neural network algorithm and ID3 decision tree algorithm based on the diagnosis data. Each algorithm are checked to get better performance. Finally, in the given open data set, SVM recommendation model is selected for the medicine recommendation module to obtain a good trade-off among model accuracy and model efficiency, Experimental results shows that our system will be able to give proper medication recommendation
Mrudula Mahajan
Data mining is a subfield of Computer Science that uses already existing data in different databases to transform it into new researches and results. It makes use of Artificial Intelligence, machine learning and database management to extract new patterns from large data sets and the knowledge associated with these patterns. Data mining techniques provides uncovering the new trends for the acute health problems. The major objective is to use the data mining techniques and the Intelligent System efficiently for the prediction of patient's disease. This can be done by taking the inputs as symptoms of the patients and providing them with the suspected disease and later also with the recommendation of the prescription of the obtained disease. Data used for this will be extracted from the Medlineplus (online) in unstructured format and by using open source tools it will be converted to structured format. The Clustering of data according to requirements and then by applying associatio...
For ceaseless sickness, Medical history reproduction is fundamental for review database investigations. It significantly affects the measurement of further analysis result. It shows the prescription development structure for the medicinal history of patients with chronic, or acute diseases. The main goal is to identify the disease name and predicting the solutions. Suggesting the medicine to the patients for using data mining (prediction) user find the location of doctor specialist from the analyzed datasets.
MEGHA RATHI
Sonali Agarwal
Worldwide, several cases go undiagnosed due to poor healthcare support in remote areas. In this context, a centralized system is needed for effective monitoring and analysis of the medical records. A web-based patient diagnostic system is a central platform to store the medical history and predict the possible disease based on the current symptoms experienced by a patient to ensure faster and accurate diagnosis. Early disease prediction can help the users determine the severity of the disease and take quick action. The proposed web-based disease prediction system utilizes machine learning based classification techniques on a data set acquired from the National Centre of Disease Control (NCDC). K-nearest neighbor (K-NN), random forest and naive bayes classification approaches are utilized and an ensemble voting algorithm is also proposed where each classifier is assigned weights dynamically based on the prediction confidence. The proposed system is also equipped with a recommendation...
International Journal of Engineering Applied Sciences and Technology
Arohi Rastogi
We have been afraid to go to the doctor in recent years because of the COVID's situation. So for minor diseases, we planned to create a web application that will be helpful for people. Our motive is to create a system for the welfare of people. In this paper, we aim to predict user's diseases based on their symptoms. We implement the Decision Tree Algorithm to reach our goal, which helps to determine the patient's health condition after collecting their symptoms by predicting the disease. This web application can determine and extract previously unseen patterns, relations, and concepts related to multiple diseases from historical database records of specified multiple diseases. The paper presents an overview of the data mining techniques with their applications in the healthcare field. In health care areas, due to regulations and due to the availability of computers, a large amount of data is becoming available. Such a large amount of data cannot be processed by humans in a short time to make a diagnosis. One of the main objectives is to examine data mining techniques in healthcare applications in order to make the best decisions possible.
Harish Rajora
Regular Issue
Dhananjay Kalbande
Loading Preview
Sorry, preview is currently unavailable. You can download the paper by clicking the button above.
RELATED PAPERS
Ijaems Journal
International journal for research in applied science and engineering technology ijraset
IJRASET Publication
International Journal of Scientific Research in Computer Science, Engineering and Information Technology
International Journal of Scientific Research in Computer Science, Engineering and Information Technology IJSRCSEIT
J4R - Journal 4 Research
J4R - Journal for Research
International Journal for Research in Applied Science & Engineering Technology (IJRASET)
International journal of health sciences
Smruti Smaraki Sarangi
International Journal of Computer Applications
Abu Abu raihan
IJIRT Journal
Alina Campan
International Journal for Research in Applied Science & Engineering Technology (IJRASET)
Prabhat Chaudhary
Journal of Pharmaceutical Research International
shailesh kamble
Saumya Shandilya
Nirmala Shinde
International Journal of Scientific Research in Science, Engineering and Technology
Prajakta Khairnar, Vamsi Avula, Aditya Hargane, Pratik Baisware , International Journal of Scientific Research in Science, Engineering and Technology IJSRSET
Modafar Ati
Satish Kamble
Alhassan Salamudeen
- We're Hiring!
- Help Center
- Find new research papers in:
- Health Sciences
- Earth Sciences
- Cognitive Science
- Mathematics
- Computer Science
- Academia ©2024

COMMENTS
Based on the symptoms, age, and gender of an individual, the diagnosis system gives the output as the disease that the individual might be suffering from. The weighted KNN algorithm gave the best results as compared to the other algorithms. The accuracy of the weighted KNN algorithm for the prediction was 93.5 %.
Bournemouth, England. [email protected]. Abstract —The wide adaptation of computer-based technology. in the health care industry resulted in the accumulation of. electronic data. Due to ...
the disease is omitted mistakenly from the consideration. Machine learning (ML) is used practically everywhere, from cutting-edge technology (such as mobile phones, computers, and robotics) to health care (i.e., disease diagnosis, safety). ML is gaining popularity in various fields, including disease diagnosis in health care.
For the analysis, a sample of 4920 patient records with 41 disorders was chosen. A total of 41 diseases made up the dependent variable. We enhanced 95 of the 132 independent variables (symptoms) that are closely related to illnesses. This paper illustrates a disease prediction system constructed using the Random Forest Machine Learning algorithm.
The numbers of disease prediction papers using XGBoost with medical data have increased recently 33,34,35,36. XGBoost is an algorithm that overcomes the shortcomings of GBM (gradient boosting ...
The research work 4 conducted a comprehensive review of ML-based disease diagnosis, examining the most recent trends and approaches in ML for disease diagnosis.
In this paper we are proposes a complete Multiple Disease Prediction System that makes accurate predictions of diabetes, cancer, and heart disease using machine learning algorithms. The system's ...
Most importantly, pooled analyses indicate that, in general, ML algorithms are accurate (AUC 0.8-0.9 s) in overall cardiovascular disease prediction. In subgroup analyses of each ML algorithms ...
The rapid integration of machine learning methodologies in healthcare has ignited innovative strategies for disease prediction, particularly with the vast repositories of Electronic Health Records (EHR) data. This article delves into the realm of multi-disease prediction, presenting a comprehensive study that introduces a pioneering ensemble feature selection model. This model, designed to ...
Cardiovascular disease refers to any critical condition that impacts the heart. Because heart diseases can be life-threatening, researchers are focusing on designing smart systems to accurately diagnose them based on electronic health data, with the aid of machine learning algorithms. This work presents several machine learning approaches for predicting heart diseases, using data of major ...
Jeyaganesan et al. (Citation 2020) derived an effective prediction system to predict cardiovascular disease utilizing the Kaggle dataset focusing on 13 attributes including ECG, BP, cholesterol, blood sugar, type of chest pain, age, gender and more. As a phase of preprocessing, the distribution of data was checked by plotting techniques, and ...
This paper can enlighten relevant researchers, help them understand the current development, existing problems and future development trend of disease prediction algorithms, and let them focus on hot spot algorithms, combine current advanced technologies and concepts, and make more efficient, effective and reasonable research with the goal of ...
Heart disease (HD), including heart attacks, is a primary cause of death across the world. In the area of medical data analysis, one of the most difficult problems to solve is determining the probability of a patient having heart disease. Death rates can be lowered by the early detection of heart diseases and the constant monitoring of patients by physicians. Unfortunately, heart disease ...
This research work carried out demonstrates the disease prediction system developed using Machine learning algorithms such as Decision Tree classifier, Random forest classifier, and Naïve Bayes classifier. The paper presents the comparative study of the results of the above algorithms used.
The suggested method proved effective for predicting heart disease with an accuracy of 80% and can be very useful for healthcare practitioners. Authors in [14] presented an automated approach for answering difficult inquiries for heart disease prediction. The Naive Bayes methodology was used to create this intelligent system in order to provide ...
ChaoTan et al [1] explored the feasibility of using decision stumps as a poor classification method and track element analysis to predict timely lung cancer in a combination of Adaboost (machine learning ensemble). For the illustration, a cancer dataset was used which identified 9 trace elements in 122 urine samples.
This paper proposed a method of identification and prediction of the presence of chronic disease in an individual using the machine learning algorithms such as CNN and KNN. The advantage of the proposed system is the use of both structured and unstructured data from real life for data set preparation, which lacks in many of the existing approaches.
Abstract. In the face of increasing health challenges, the "Multiple Disease Prediction System Using Machine Learning" project strives to address a critical concern: the proactive identification and prediction of various diseases for effective healthcare management.
This prediction is an area that is widely researched. Our paper is part of the research on the detection and prediction of heart disease. It is based on the application of Machine Learning ...
Research on disease outbreak prediction has suddenly received an enormous interest owing to the COVID-19 pandemic. Natural language processing using user-generated text data has proven to be quite effective for the same. Disease outbreaks that occur frequently can be easily predicted, but novel disease outbreaks are difficult to predict. This review work attempts to summarize the research ...
Disease prediction is crucial in healthcare, enabling professionals to diagnose and treat diseases more effectively. In recent years, machine learning and web technology have emerged as powerful tools for predicting various diseases. Machine learning algorithms can analyze large and complex datasets to learn patterns and relationships in the data, enabling them to make accurate disease ...
In this paper, we developed an intelligent predictive system based on contemporary machine learning algorithms for the prediction and diagnosis of heart disease. The developed system was checked ...
In this paper, we are using machine learning algorithms that try to accurately predict possible diseases. The results generated by the proposed system have an accuracy of up to 87%. The system has incredible potential in anticipating the possible diseases more precisely.
The system then runs the corresponding machine learning model, predicts the output, and displays it on the screen. It's like having all your disease predictions in one convenient spot. 1.2 Problem Statement One challenge faced in developing a multiple disease prediction system for diabetes, heart and Parkinson's diseases is ensuring the
Prediction. abstract. Data mining for healthcare is an interdisciplinary field of study that originated in database statistics and. is useful in examining the effectiveness of medical therapies ...
Abstract. Disease Prediction using Machine Learning is the system that is used to predict the diseases from the symptoms which are given by the patients or any user. The system processes the ...
Machine learning (ML) refers to the science and engineering of artificially intelligent systems, providing them with the capability to learn without being explicitly programmed. In recent years, ML in the healthcare domain has made great advancements in the early predictions of many critical illnesses. While there have been significant contributions to single disease prediction systems (like ...
Heart disease is quickly becoming one of the most critical and widespread health concerns in the globe at the present time. At this time, a great deal of research is being conducted in an effort to treat a variety of cardiac conditions and a great number of lives. Because it enables medical practitioners to begin treating patients as soon as possible, early diagnosis of any heart illness is ...
The main objective of this research paper is to summarize the recent research with comparative results that has been done on heart disease prediction and also make analytical conclusions.
Disease prediction is done by medical profiles such as blood sugar, blood pressure, blood oxygen, headache and other symptoms. Based on this, the most probable disease is predicted by Naive Bayes classifier. 1.2 Recommendation System In addition to this, the specialists for the predicted disease are recommended based on filters chosen by user.