- Columbia University in the City of New York
- Office of Teaching, Learning, and Innovation
- University Policies
- Columbia Online
- Academic Calendar
- Resources and Technology
- Instructional Technologies
- Teaching in All Modalities

Designing Assignments for Learning
The rapid shift to remote teaching and learning meant that many instructors reimagined their assessment practices. Whether adapting existing assignments or creatively designing new opportunities for their students to learn, instructors focused on helping students make meaning and demonstrate their learning outside of the traditional, face-to-face classroom setting. This resource distills the elements of assignment design that are important to carry forward as we continue to seek better ways of assessing learning and build on our innovative assignment designs.
On this page:
Rethinking traditional tests, quizzes, and exams.
- Examples from the Columbia University Classroom
- Tips for Designing Assignments for Learning
Reflect On Your Assignment Design
Connect with the ctl.
- Resources and References

Cite this resource: Columbia Center for Teaching and Learning (2021). Designing Assignments for Learning. Columbia University. Retrieved [today’s date] from https://ctl.columbia.edu/resources-and-technology/teaching-with-technology/teaching-online/designing-assignments/
Traditional assessments tend to reveal whether students can recognize, recall, or replicate what was learned out of context, and tend to focus on students providing correct responses (Wiggins, 1990). In contrast, authentic assignments, which are course assessments, engage students in higher order thinking, as they grapple with real or simulated challenges that help them prepare for their professional lives, and draw on the course knowledge learned and the skills acquired to create justifiable answers, performances or products (Wiggins, 1990). An authentic assessment provides opportunities for students to practice, consult resources, learn from feedback, and refine their performances and products accordingly (Wiggins 1990, 1998, 2014).
Authentic assignments ask students to “do” the subject with an audience in mind and apply their learning in a new situation. Examples of authentic assignments include asking students to:
- Write for a real audience (e.g., a memo, a policy brief, letter to the editor, a grant proposal, reports, building a website) and/or publication;
- Solve problem sets that have real world application;
- Design projects that address a real world problem;
- Engage in a community-partnered research project;
- Create an exhibit, performance, or conference presentation ;
- Compile and reflect on their work through a portfolio/e-portfolio.
Noteworthy elements of authentic designs are that instructors scaffold the assignment, and play an active role in preparing students for the tasks assigned, while students are intentionally asked to reflect on the process and product of their work thus building their metacognitive skills (Herrington and Oliver, 2000; Ashford-Rowe, Herrington and Brown, 2013; Frey, Schmitt, and Allen, 2012).
It’s worth noting here that authentic assessments can initially be time consuming to design, implement, and grade. They are critiqued for being challenging to use across course contexts and for grading reliability issues (Maclellan, 2004). Despite these challenges, authentic assessments are recognized as beneficial to student learning (Svinicki, 2004) as they are learner-centered (Weimer, 2013), promote academic integrity (McLaughlin, L. and Ricevuto, 2021; Sotiriadou et al., 2019; Schroeder, 2021) and motivate students to learn (Ambrose et al., 2010). The Columbia Center for Teaching and Learning is always available to consult with faculty who are considering authentic assessment designs and to discuss challenges and affordances.
Examples from the Columbia University Classroom
Columbia instructors have experimented with alternative ways of assessing student learning from oral exams to technology-enhanced assignments. Below are a few examples of authentic assignments in various teaching contexts across Columbia University.
- E-portfolios: Statia Cook shares her experiences with an ePorfolio assignment in her co-taught Frontiers of Science course (a submission to the Voices of Hybrid and Online Teaching and Learning initiative); CUIMC use of ePortfolios ;
- Case studies: Columbia instructors have engaged their students in authentic ways through case studies drawing on the Case Consortium at Columbia University. Read and watch a faculty spotlight to learn how Professor Mary Ann Price uses the case method to place pre-med students in real-life scenarios;
- Simulations: students at CUIMC engage in simulations to develop their professional skills in The Mary & Michael Jaharis Simulation Center in the Vagelos College of Physicians and Surgeons and the Helene Fuld Health Trust Simulation Center in the Columbia School of Nursing;
- Experiential learning: instructors have drawn on New York City as a learning laboratory such as Barnard’s NYC as Lab webpage which highlights courses that engage students in NYC;
- Design projects that address real world problems: Yevgeniy Yesilevskiy on the Engineering design projects completed using lab kits during remote learning. Watch Dr. Yesilevskiy talk about his teaching and read the Columbia News article .
- Writing assignments: Lia Marshall and her teaching associate Aparna Balasundaram reflect on their “non-disposable or renewable assignments” to prepare social work students for their professional lives as they write for a real audience; and Hannah Weaver spoke about a sandbox assignment used in her Core Literature Humanities course at the 2021 Celebration of Teaching and Learning Symposium . Watch Dr. Weaver share her experiences.
Tips for Designing Assignments for Learning
While designing an effective authentic assignment may seem like a daunting task, the following tips can be used as a starting point. See the Resources section for frameworks and tools that may be useful in this effort.
Align the assignment with your course learning objectives
Identify the kind of thinking that is important in your course, the knowledge students will apply, and the skills they will practice using through the assignment. What kind of thinking will students be asked to do for the assignment? What will students learn by completing this assignment? How will the assignment help students achieve the desired course learning outcomes? For more information on course learning objectives, see the CTL’s Course Design Essentials self-paced course and watch the video on Articulating Learning Objectives .
Identify an authentic meaning-making task
For meaning-making to occur, students need to understand the relevance of the assignment to the course and beyond (Ambrose et al., 2010). To Bean (2011) a “meaning-making” or “meaning-constructing” task has two dimensions: 1) it presents students with an authentic disciplinary problem or asks students to formulate their own problems, both of which engage them in active critical thinking, and 2) the problem is placed in “a context that gives students a role or purpose, a targeted audience, and a genre.” (Bean, 2011: 97-98).
An authentic task gives students a realistic challenge to grapple with, a role to take on that allows them to “rehearse for the complex ambiguities” of life, provides resources and supports to draw on, and requires students to justify their work and the process they used to inform their solution (Wiggins, 1990). Note that if students find an assignment interesting or relevant, they will see value in completing it.
Consider the kind of activities in the real world that use the knowledge and skills that are the focus of your course. How is this knowledge and these skills applied to answer real-world questions to solve real-world problems? (Herrington et al., 2010: 22). What do professionals or academics in your discipline do on a regular basis? What does it mean to think like a biologist, statistician, historian, social scientist? How might your assignment ask students to draw on current events, issues, or problems that relate to the course and are of interest to them? How might your assignment tap into student motivation and engage them in the kinds of thinking they can apply to better understand the world around them? (Ambrose et al., 2010).
Determine the evaluation criteria and create a rubric
To ensure equitable and consistent grading of assignments across students, make transparent the criteria you will use to evaluate student work. The criteria should focus on the knowledge and skills that are central to the assignment. Build on the criteria identified, create a rubric that makes explicit the expectations of deliverables and share this rubric with your students so they can use it as they work on the assignment. For more information on rubrics, see the CTL’s resource Incorporating Rubrics into Your Grading and Feedback Practices , and explore the Association of American Colleges & Universities VALUE Rubrics (Valid Assessment of Learning in Undergraduate Education).
Build in metacognition
Ask students to reflect on what and how they learned from the assignment. Help students uncover personal relevance of the assignment, find intrinsic value in their work, and deepen their motivation by asking them to reflect on their process and their assignment deliverable. Sample prompts might include: what did you learn from this assignment? How might you draw on the knowledge and skills you used on this assignment in the future? See Ambrose et al., 2010 for more strategies that support motivation and the CTL’s resource on Metacognition ).
Provide students with opportunities to practice
Design your assignment to be a learning experience and prepare students for success on the assignment. If students can reasonably expect to be successful on an assignment when they put in the required effort ,with the support and guidance of the instructor, they are more likely to engage in the behaviors necessary for learning (Ambrose et al., 2010). Ensure student success by actively teaching the knowledge and skills of the course (e.g., how to problem solve, how to write for a particular audience), modeling the desired thinking, and creating learning activities that build up to a graded assignment. Provide opportunities for students to practice using the knowledge and skills they will need for the assignment, whether through low-stakes in-class activities or homework activities that include opportunities to receive and incorporate formative feedback. For more information on providing feedback, see the CTL resource Feedback for Learning .
Communicate about the assignment
Share the purpose, task, audience, expectations, and criteria for the assignment. Students may have expectations about assessments and how they will be graded that is informed by their prior experiences completing high-stakes assessments, so be transparent. Tell your students why you are asking them to do this assignment, what skills they will be using, how it aligns with the course learning outcomes, and why it is relevant to their learning and their professional lives (i.e., how practitioners / professionals use the knowledge and skills in your course in real world contexts and for what purposes). Finally, verify that students understand what they need to do to complete the assignment. This can be done by asking students to respond to poll questions about different parts of the assignment, a “scavenger hunt” of the assignment instructions–giving students questions to answer about the assignment and having them work in small groups to answer the questions, or by having students share back what they think is expected of them.
Plan to iterate and to keep the focus on learning
Draw on multiple sources of data to help make decisions about what changes are needed to the assignment, the assignment instructions, and/or rubric to ensure that it contributes to student learning. Explore assignment performance data. As Deandra Little reminds us: “a really good assignment, which is a really good assessment, also teaches you something or tells the instructor something. As much as it tells you what students are learning, it’s also telling you what they aren’t learning.” ( Teaching in Higher Ed podcast episode 337 ). Assignment bottlenecks–where students get stuck or struggle–can be good indicators that students need further support or opportunities to practice prior to completing an assignment. This awareness can inform teaching decisions.
Triangulate the performance data by collecting student feedback, and noting your own reflections about what worked well and what did not. Revise the assignment instructions, rubric, and teaching practices accordingly. Consider how you might better align your assignment with your course objectives and/or provide more opportunities for students to practice using the knowledge and skills that they will rely on for the assignment. Additionally, keep in mind societal, disciplinary, and technological changes as you tweak your assignments for future use.
Now is a great time to reflect on your practices and experiences with assignment design and think critically about your approach. Take a closer look at an existing assignment. Questions to consider include: What is this assignment meant to do? What purpose does it serve? Why do you ask students to do this assignment? How are they prepared to complete the assignment? Does the assignment assess the kind of learning that you really want? What would help students learn from this assignment?
Using the tips in the previous section: How can the assignment be tweaked to be more authentic and meaningful to students?
As you plan forward for post-pandemic teaching and reflect on your practices and reimagine your course design, you may find the following CTL resources helpful: Reflecting On Your Experiences with Remote Teaching , Transition to In-Person Teaching , and Course Design Support .
The Columbia Center for Teaching and Learning (CTL) is here to help!
For assistance with assignment design, rubric design, or any other teaching and learning need, please request a consultation by emailing [email protected] .
Transparency in Learning and Teaching (TILT) framework for assignments. The TILT Examples and Resources page ( https://tilthighered.com/tiltexamplesandresources ) includes example assignments from across disciplines, as well as a transparent assignment template and a checklist for designing transparent assignments . Each emphasizes the importance of articulating to students the purpose of the assignment or activity, the what and how of the task, and specifying the criteria that will be used to assess students.
Association of American Colleges & Universities (AAC&U) offers VALUE ADD (Assignment Design and Diagnostic) tools ( https://www.aacu.org/value-add-tools ) to help with the creation of clear and effective assignments that align with the desired learning outcomes and associated VALUE rubrics (Valid Assessment of Learning in Undergraduate Education). VALUE ADD encourages instructors to explicitly state assignment information such as the purpose of the assignment, what skills students will be using, how it aligns with course learning outcomes, the assignment type, the audience and context for the assignment, clear evaluation criteria, desired formatting, and expectations for completion whether individual or in a group.
Villarroel et al. (2017) propose a blueprint for building authentic assessments which includes four steps: 1) consider the workplace context, 2) design the authentic assessment; 3) learn and apply standards for judgement; and 4) give feedback.
References
Ambrose, S. A., Bridges, M. W., & DiPietro, M. (2010). Chapter 3: What Factors Motivate Students to Learn? In How Learning Works: Seven Research-Based Principles for Smart Teaching . Jossey-Bass.
Ashford-Rowe, K., Herrington, J., and Brown, C. (2013). Establishing the critical elements that determine authentic assessment. Assessment & Evaluation in Higher Education. 39(2), 205-222, http://dx.doi.org/10.1080/02602938.2013.819566 .
Bean, J.C. (2011). Engaging Ideas: The Professor’s Guide to Integrating Writing, Critical Thinking, and Active Learning in the Classroom . Second Edition. Jossey-Bass.
Frey, B. B, Schmitt, V. L., and Allen, J. P. (2012). Defining Authentic Classroom Assessment. Practical Assessment, Research, and Evaluation. 17(2). DOI: https://doi.org/10.7275/sxbs-0829
Herrington, J., Reeves, T. C., and Oliver, R. (2010). A Guide to Authentic e-Learning . Routledge.
Herrington, J. and Oliver, R. (2000). An instructional design framework for authentic learning environments. Educational Technology Research and Development, 48(3), 23-48.
Litchfield, B. C. and Dempsey, J. V. (2015). Authentic Assessment of Knowledge, Skills, and Attitudes. New Directions for Teaching and Learning. 142 (Summer 2015), 65-80.
Maclellan, E. (2004). How convincing is alternative assessment for use in higher education. Assessment & Evaluation in Higher Education. 29(3), June 2004. DOI: 10.1080/0260293042000188267
McLaughlin, L. and Ricevuto, J. (2021). Assessments in a Virtual Environment: You Won’t Need that Lockdown Browser! Faculty Focus. June 2, 2021.
Mueller, J. (2005). The Authentic Assessment Toolbox: Enhancing Student Learning through Online Faculty Development . MERLOT Journal of Online Learning and Teaching. 1(1). July 2005. Mueller’s Authentic Assessment Toolbox is available online.
Schroeder, R. (2021). Vaccinate Against Cheating With Authentic Assessment . Inside Higher Ed. (February 26, 2021).
Sotiriadou, P., Logan, D., Daly, A., and Guest, R. (2019). The role of authentic assessment to preserve academic integrity and promote skills development and employability. Studies in Higher Education. 45(111), 2132-2148. https://doi.org/10.1080/03075079.2019.1582015
Stachowiak, B. (Host). (November 25, 2020). Authentic Assignments with Deandra Little. (Episode 337). In Teaching in Higher Ed . https://teachinginhighered.com/podcast/authentic-assignments/
Svinicki, M. D. (2004). Authentic Assessment: Testing in Reality. New Directions for Teaching and Learning. 100 (Winter 2004): 23-29.
Villarroel, V., Bloxham, S, Bruna, D., Bruna, C., and Herrera-Seda, C. (2017). Authentic assessment: creating a blueprint for course design. Assessment & Evaluation in Higher Education. 43(5), 840-854. https://doi.org/10.1080/02602938.2017.1412396
Weimer, M. (2013). Learner-Centered Teaching: Five Key Changes to Practice . Second Edition. San Francisco: Jossey-Bass.
Wiggins, G. (2014). Authenticity in assessment, (re-)defined and explained. Retrieved from https://grantwiggins.wordpress.com/2014/01/26/authenticity-in-assessment-re-defined-and-explained/
Wiggins, G. (1998). Teaching to the (Authentic) Test. Educational Leadership . April 1989. 41-47.
Wiggins, Grant (1990). The Case for Authentic Assessment . Practical Assessment, Research & Evaluation , 2(2).
Wondering how AI tools might play a role in your course assignments?
See the CTL’s resource “Considerations for AI Tools in the Classroom.”
This website uses cookies to identify users, improve the user experience and requires cookies to work. By continuing to use this website, you consent to Columbia University's use of cookies and similar technologies, in accordance with the Columbia University Website Cookie Notice .

How do I create and track Assignments?
The Assignments feature allows Premium members to assign specific worksheets, games or exercises to their students. The student can complete the assignment digitally and submit it for you to review. The Assignments manager can be found under your Premium user ID in the top right-hand corner and selecting My Assignments.
How to Assign Worksheets, Games or Exercises:
– Select the content you’d like to assign, for example a worksheet, game, exercise, or Guided Lesson.
– Click on the “Assign Digitally” button.
– From here, you have two options: Add to an existing Assignment or Add to a new Assignment.
– If you select “ Create new Assignment, ” give it a name. You can select how many attempts the student gets to complete the Assignment, and also if you would like this Assignment to be “Mandatory.” These are both optional, but if “Mandatory” is enabled, students must complete this Assignment before they will be able to play other games within the Brainzy islands.
– Click “Continue.”
– Select the learner(s) you want to send this Assignment to, and then you can choose to select “Publish Assignment.” If you don’t publish now, this Assignment will be saved as a draft. If you have not yet added a student to your account, you can do so in the process of creating an Assignment.
– If you prefer to add this new content to an existing assignment, Select “Assign Digitally” then choose the assignment you would like to add it to and select “Add.”
– If you would like to create an Assignment but you do not want your children to immediately see it, then you can toggle off “Publish assignment?” to save it as a draft.
– When you are finished adding children to the Assignment, then you can click “Save and Finish.” You will see a confirmation message once it has been successfully assigned.
Please note: There is a maximum limit of 50 items per assignment.
How Children Can Access Their Assignments:
– As a parent, if you choose, your children can log in to your account at Education.com using your Premium membership email and password.
– Teachers can have students log in to our kid-safe environment at education.com/games/play . Please have them select “Sign In” and on the next screen they can select “Use Access Code” and then enter their Classroom or Student Code. Classroom Code is recommended for use in the classroom only, while Student Code is recommended for use at home.
– Once the student selects their profile, they will land on our main menu where they will see available Assignments and due dates (if applicable).
– To complete the Assignments, students click on the games or exercises listed on the Assignment page, click “Play,” learn and have fun!
The main menu also allows students to see their progress in each individual game and exercise in the Assignment.
Editing an Assignment or Changing the due date:
– To access your Assignments, sign in to your account and click on your Premium user ID in the top right-hand corner of any page. – You can then select “My Assignments.” You’ll be able to make edits to Assignments here, such as removing items, changing the due dates, editing the children assigned to them or deleting. – When you open the “My Assignments” page, you will see “Draft” which contains any Assignments you have created but not yet assigned, “In Progress” which contains all active Assignments, and also “Past Due” which contains all past due or finished Assignments.
– To edit an Assignment (such as changing its name, due date, number of attempts, mandatory, or a draft), click on it in the table, and then click on “Edit Assignment.” You can edit its details and also duplicate or delete it.
– If you click “Start Assignment” for a Draft Assignment, then it will be sent to your “In Progress” assignments, and also made active for the children to play.
– To change the learners who are on an Assignment, scroll down to “Assigned Children” and click “Edit,” which can be found to the right of the title.
– To quickly change an assignment to/from mandatory , click on the assignment in the table and you will see the column to the right “Mandatory”. Each assignment will have a Yes or No for this. If “Yes” is selected students must complete this Assignment before they will be able to play other games. Click on “Yes” or “No” to switch the setting.
– If you select “Edit” next to the Assigned Items of an assignment, you can reorder the content of the assignment or delete an item from the assignment.
Track Assignment Progress:
– On the “My Assignments” page, you will see a progress bar for “Student Progress.” This shows how many children have started the Assignment. For example, if there are two children assigned to an Assignment, and one has finished it, then you will see “1 finished · 1 not started.”
– To see more detailed progress for each child, click on an Assignment. If you scroll down to “Assigned Children,” then you can click on their name in the table to see their progress on specific items.
– To grade digitally assigned worksheets, once you have selected the student, click on “Set Score” next to the worksheet title. You will be able to view the completed work, and you can then enter the score for the worksheet.
– You can then repeat this process if there are multiple students in the Assignment.
– Please Note: The Assignment only becomes “Finished” when the due date passes. If your children complete the Assignment before the due date, then it will remain “In Progress.”
Educational Tools
- Learning Library
- Interactive Worksheets
- Lesson Plans
- Common Core Resources
- Guided Lessons
- Progress Tracker
- Help center
- Education.com for Schools
- Get a Quote
- Redeem Gift
- Tell Us What You Think
- Privacy Policy
- COPPA Privacy Policy
- Terms of Service
Copyright @2024 Education.com, Inc, a division of IXL Learning • All Rights Reserved.
- About Education.com
- Your Account
- Student Access / Brainzy
- Premium Features
- Purchase Information
- Technical Help
Google Assignments, your new grading companion
Aug 14, 2019
[[read-time]] min read
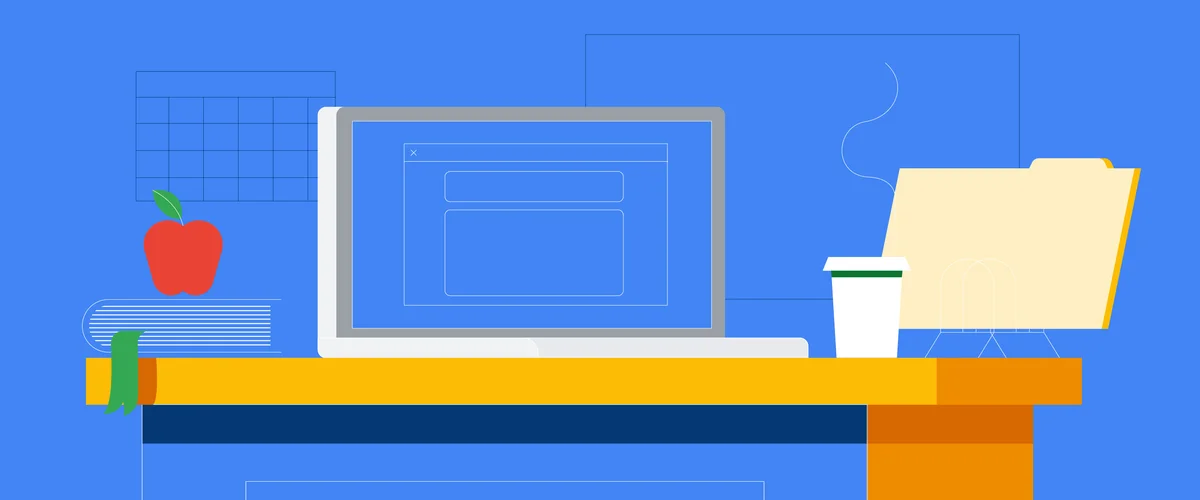
Instructors lose valuable time doing cumbersome tasks: writing the same comment on multiple essays, returning piles of paper assignments, and battling copy machine jams. These frustrations are most often felt by instructors with the highest teaching workloads and the least time. For the last five years, we’ve been building tools—like Classroom and Quizzes in Google Forms—to address these challenges. Now you can take advantage of these tools if you use a traditional Learning Management System (LMS).
Assignments brings together the capabilities of Google Docs, Drive and Search into a new tool for collecting and grading student work. It helps you save time with streamlined assignment workflows, ensure student work is authentic with originality reports, and give constructive feedback with comment banks. You can use Assignments as a standalone tool and a companion to your LMS (no setup required!) or your school admin can integrate it with your LMS. Sign up today to try Assignments.
If you're one of the 40 million people using Classroom: you've got the best of Assignments already baked in, including our new originality reports . For everyone else, Assignments gives you access to these features as a compliment to your school’s LMS.
Assignments is your tireless grading companion
Using an LMS can create more work than it saves: students turn in all kinds of files, you have to download and re-upload student files one-by-one, and what if students can keep editing after they already turned in their work? Assignments handles all this for you.
Assignments streamlines the creation and management of coursework, and tackles some of your biggest frustrations:
Stop typing the same feedback over and over by using a comment bank, and never worry about pressing the “save” button again
Check student work for originality and automatically lock work once it’s turned in
Assign files with the option to send each student a copy (no more copy machines!)
Grade assignments for an entire class with a student switcher and rubrics, and review any file type without leaving your grading interface
Comment and leave suggestions on student work with Google Docs
Instructors and students can attach anything to assignments: Docs or Word files for papers, spreadsheets for data analysis, slides for presentations, sites for digital portfolios or final projects, Colab notebooks for programming exercises, and much more.
Help students turn in their best work with originality reports
With originality reports in Assignments, you can check student work for missed citations and possible plagiarism without interrupting your grading workflow. When students turn in a document, Assignments will check students’ text against hundreds of billions of web pages and tens of millions of books.
If you enable originality reports on an assignment, students can also check their work for authenticity (a limited number of times) to correct issues, turn in their best work, and save instructors time grading. Since both you and your students can see originality reports, they’re designed to help you teach your students about authenticity and academic integrity.
Getting started with Assignments
Starting today, you can sign up to get access to Assignments when it becomes available in a few weeks. Assignments will be available for free as part of G Suite for Education and can be used by instructors alongside or integrated with an LMS.
Instructors can use Assignments even if your school has an LMS. There’s no setup required, all you need is to sign up and have a school-issued Google account.
Admins can turn on access to Assignments within your LMS. Assignments is available as an LTI tool, which provides a more integrated experience and enables roster syncing and grade transmission to your LMS gradebook. Assignments is an improved and expanded version of Course Kit, so if you’re already in the Course Kit beta, you’ll automatically have access to Assignments.
If you use Canvas, we’ve worked with their team to complement the Assignments LTI tool with a set of additional features that make Docs and Drive work seamlessly across all Canvas assignments.
Related stories
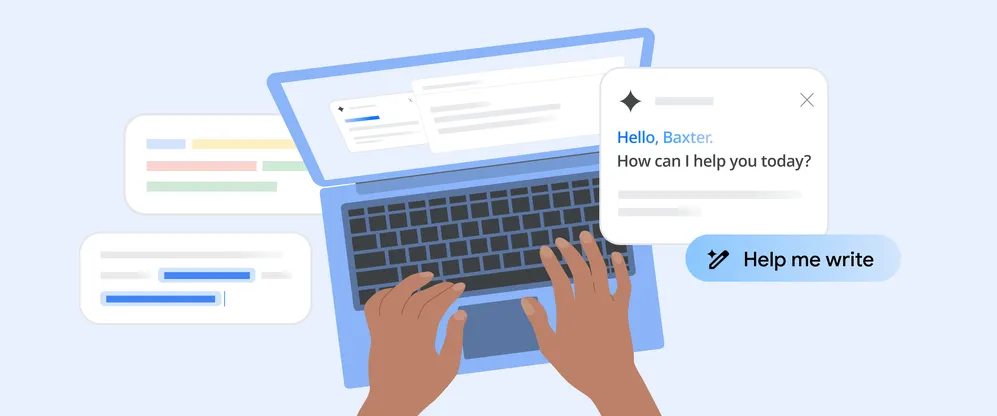
5 tips for writing great prompts for Gemini in the Workspace side panel
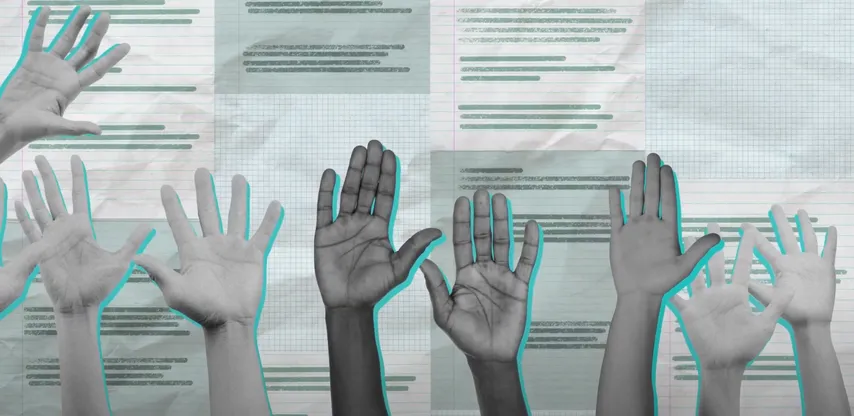
3 things parents and students told us about how generative AI can support learning
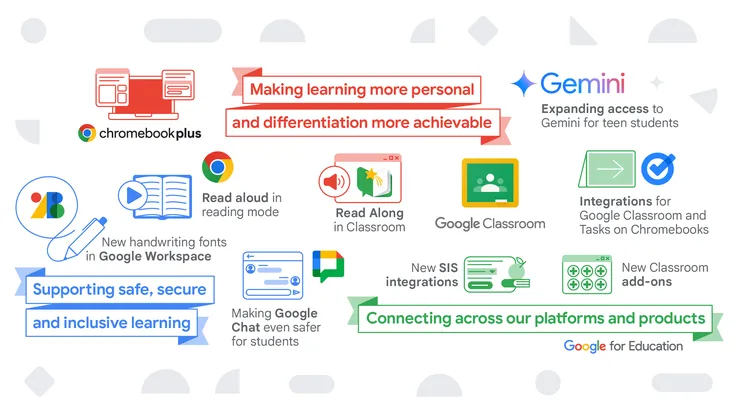
Updates on how we're using AI to support students and educators
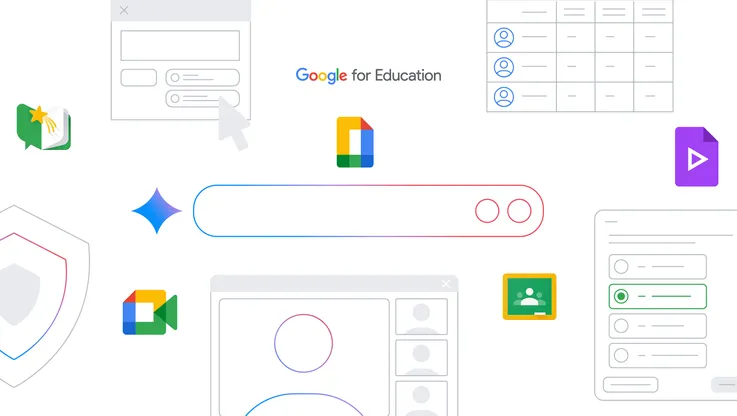
New AI tools for Google Workspace for Education
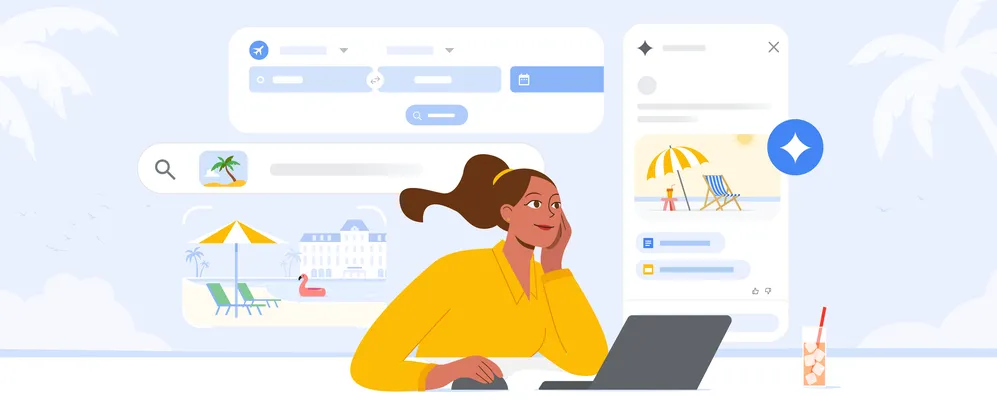
Use these 5 AI-powered tools to plan your summer travel
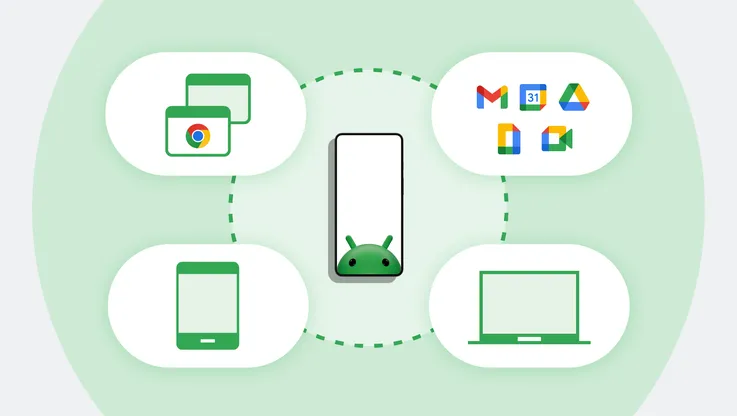
How we’re making Android Enterprise signup and access to Google services better
Let’s stay in touch. Get the latest news from Google in your inbox.
- Help Center
- Assignments
- Privacy Policy
- Terms of Service
- Submit feedback
About Assignments LTI™
Assignments is an add-on application for learning management systems (LMSs) that helps you distribute, analyze, and grade student work with Google Workspace for Education.
Assignments makes Google Docs and Google Drive compatible with your LMS for file submissions. You can use Assignments to save time distributing and grading student work, and analyze student submissions with originality reports to ensure authenticity.
- It works with any LMS that supports LTI version 1.1, such as Canvas, Blackboard, Moodle, and more.
- You can also use Assignments with LTI version 1.3 for Canvas and Schoology.
- Adding Assignments to your LMS might require assistance from your LMS administrator. If you have never set up an add-on application or external tool for your LMS, talk to your IT admin or LMS admin about setting up Assignments in Canvas , or another LMS, such as Schoology .
- If you use an account associated with your educational institution, terms may apply based on your organization's agreement with Google. If you don’t use a managed account, the Google Terms of Service may apply.
- At this time, Google Workspace Admin support for installation help and troubleshooting is only available for customers using Canvas . If you use a different LMS, help is available in the community forum .
To get the latest updates on Assignments, follow Google for Education Twitter and subscribe to the Google for Education blog .
Features and benefits of Assignments
Distribute personalized google drive templates and worksheets to students.
- Assign and collect virtually any file type, including Google Docs and Microsoft® Word files.
- Automatically distribute a personalized copy of assignment files for each student.
- Each distributed copy will be labeled with a student’s name and organized in a Drive folder.
Spot missed citations and possible plagiarism with originality reports
- Assess student work for originality without leaving your grading tool.
- Searches hundreds of billions of web pages and 40 million books.
- Help students learn to support their ideas by letting them scan their work for missed citations up to three times before submitting.
- For Google Workspace for Education Plus customers, originality reports will scan past student submissions for student-to-student matches within your school’s domain.
Save time grading while providing rich feedback
- Prevents students from editing their work while you grade.
- Grade with rubrics to keep grading consistent and transparent.
- Give rich feedback and suggestions on student work using the power of Google Docs, including margin comments, strikethroughs, and highlighting.
- Easily reuse frequent margin comments with a personalized comment bank.
- Grades save to the LMS gradebook.
Get started with Assignments
- Set up Assignments in Canvas
- Set up Assignments in Schoology
- Set up Assignments in Blackboard
- Set up Assignments in D2L/Brightspace
- Set up Assignments in Moodle
- Set up Assignments in Sakai
For educators
Related articles.
- How Assignments works
- Set up Assignments in Schoology or another LMS
- Educator's guide to Assignments setup
Need more help?
Try these next steps:.

mindmyeducation.com
educational tips that are always on your mind
9 Common Types of Assignment in Education
Education assignments take different formats based on the unit you are studying and the goals a tutor wants to achieve. The concept being tested will also dfetermine the type of assignment.
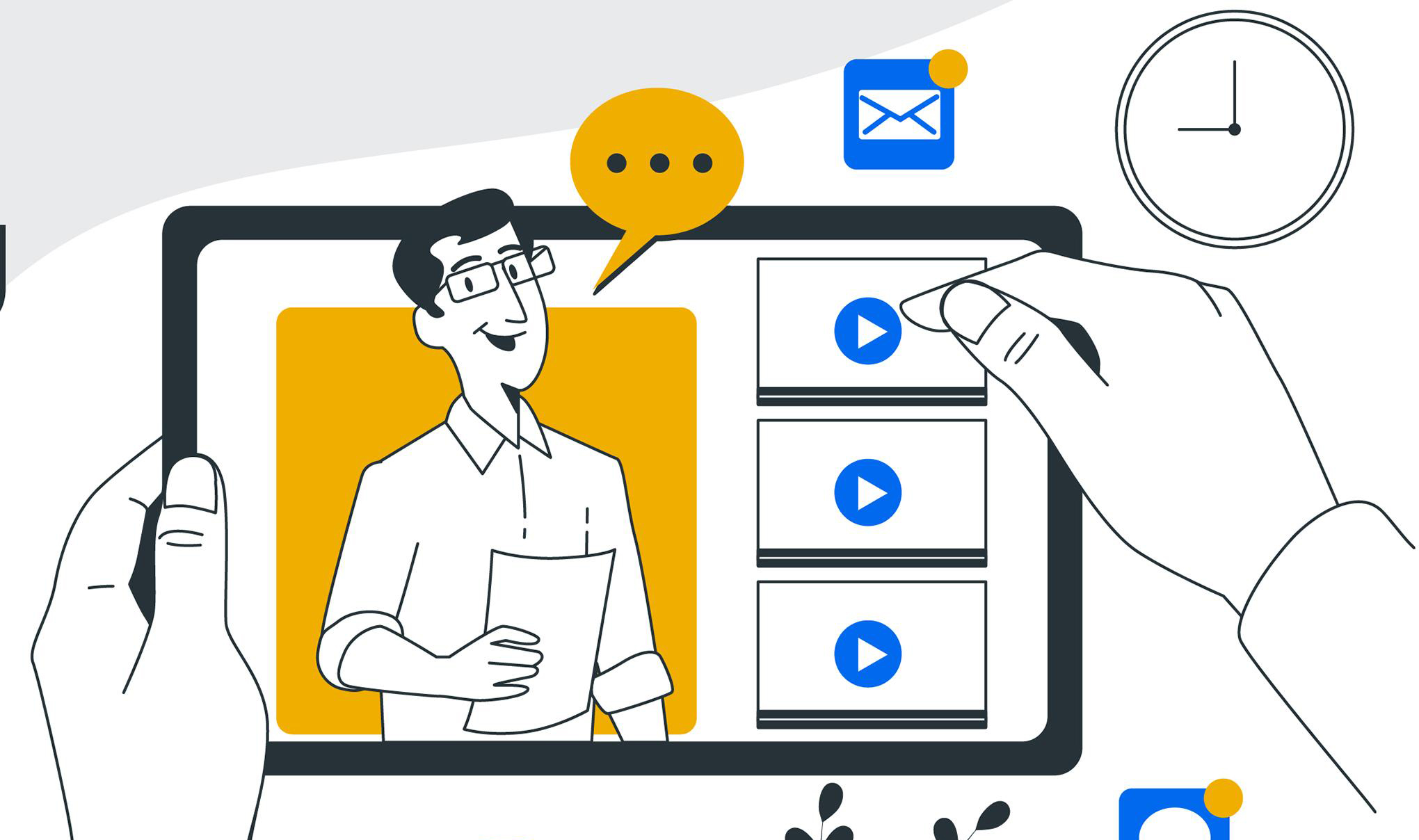
Here are the 9 most common types of assignments you will find in a class setting.
If you as what is assignment in education, the most obvious answer you will get is an essay. Essays come with topics and prompts that direct the student on the issues to study. A student may also be required to craft a topic around a subject.
An essay-assignment requires you to adhere to the instructions provided. You must also follow a set academic structure and format your paper by adhering to particular rules. A sample, example, or template will help you to produce a better essay.
- Case studies
A case study involves examining a particular situation from an academic lens. You are mainly required to use the knowledge acquired while studying a specific topic. For instance, you may study the civil rights movement and are asked to study Rosa Park’s influence. The cases help you to understand a concept better.
- Creativity assignments
A creativity exercise is one of the types of assignment in education that examine the ability of the student to practice what he has learned in class. Once you learn to write a short story or film script, the assignment may require you to write one. The results will help your tutor to evaluate your level of understanding.
The education system is supposed to teach kids the power of collaboration. Some of the assignments will involve group work. The students are supposed to work on an essay or collect data together. It ensures that students can learn to work as a team, a skill they will need in workplaces and when completing personal projects.
Group work requires a team leader. In this social distance era, the team has to discover ways to collaborate. They can use online tools for meetings and document editing. This is the ultimate test for collaboration.
- Field assignments
Field assignments involve collecting data, interviews, and observations. It may also involve a visit to historical sites, watching games, and observing phenomena like the launch of a rocket. It is a type of education assignment that brings the student face to face with an actual situation. It takes the students away from the library, giving them a different learning experience.
The tests came with questions about past topics. The tutor in such a case wants to assess how well you understood past topics. It will help him to improve your performance in future topics. It will also help you to determine how well you understood the topic and whether you need further clarification.
- Future-tests
The questions or tests focus on topics that you are yet to cover. It is a model of assignment on education that will prepare the student for what is to come. Assignments on future tests require you to read ahead. You will be better prepared for the next lesson. It also tests your diligence in the search for new materials that can be used to make the topics easier to understand.
- Practical tests
The tests involve practical work. You build a robot or enact a play. It is also an application type of assignment. The tutor wants you to innovate and test the skills or concepts you have learned.
Research assignments want you to discover a mystery behind a subject or topic. You have to collect data, review literature, and develop a hypothesis. It is the purest form of academic writing.
The different kinds of writing assignments combine with practical work to assess your ability to demonstrate your knowledge. You can get help on all these assignments by choosing to pay someone to do my homework online. They also require a strategy that will help you to achieve the set objectives.
Leave a Reply Cancel reply
Your email address will not be published. Required fields are marked *
Filter Results
- clear all filters
Resource Type
- Worksheets
- Guided Lessons
- Lesson Plans
- Hands-on Activities
- Interactive Stories
- Online Exercises
- Printable Workbooks
- Science Projects
- Song Videos
middle-school
- Fine arts
- Foreign language
- Math
- Reading & Writing
- Science
- Social emotional
- Social studies
- Typing
- Arts & crafts
- Coloring
- Holidays
- Offline games
- Pop Culture & Events
- Seasonal
- Teacher Resources
- Common Core
Free Worksheets and Printables for Kids

Make Learning Fun with Printable Worksheets

Advantages and Disadvantages of Assignments For Students
Looking for advantages and disadvantages of Assignments For Students?
We have collected some solid points that will help you understand the pros and cons of Assignments For Students in detail.
But first, let’s understand the topic:
What is Assignments For Students?
What are the advantages and disadvantages of assignments for students.
The following are the advantages and disadvantages of Assignments For Students:
Advantages | Disadvantages |
---|---|
Boosts understanding of topics | Can increase stress levels |
Encourages independent learning | Limits free time |
Enhances time management skills | May discourage creativity |
Improves research and writing abilities | Risks of plagiarism |
Reinforces classroom learning | Difficulty understanding instructions |

Advantages of Assignments For Students
Disadvantages of assignments for students.
You can view other “advantages and disadvantages of…” posts by clicking here .
If you have a related query, feel free to let us know in the comments below.
Also, kindly share the information with your friends who you think might be interested in reading it.
Leave a Reply Cancel reply
Your email address will not be published. Required fields are marked *
About The Education Hub
- Course info
- Your courses
- ____________________________
- Using our resources
- Login / Account
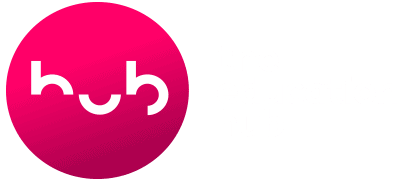
Vygotsky’s philosophy of education
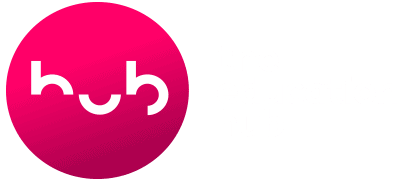
- Curriculum integration
- Health, PE & relationships
- Literacy (primary level)
- Practice: early literacy
- Literacy (secondary level)
- Mathematics
Diverse learners
- Gifted and talented
- Neurodiversity
- Speech and language differences
- Trauma-informed practice
- Executive function
- Movement and learning
- Science of learning
- Self-efficacy
- Self-regulation
- Social connection
- Social-emotional learning
- Principles of assessment
- Assessment for learning
- Measuring progress
- Self-assessment
Instruction and pedagogy
- Classroom management
- Culturally responsive pedagogy
- Co-operative learning
- High-expectation teaching
- Philosophical approaches
- Planning and instructional design
- Questioning
Relationships
- Home-school partnerships
- Student wellbeing NEW
- Transitions
Teacher development
- Instructional coaching
- Professional learning communities
- Teacher inquiry
- Teacher wellbeing
- Instructional leadership
- Strategic leadership
Learning environments
- Flexible spaces
- Neurodiversity in Primary Schools
- Neurodiversity in Secondary Schools
Lev Vygotsky (1896-1934) was part of a group of revolutionary scholars who were working at the beginning of the twentieth century to create a new school of Soviet psychology. Vygotsky himself produced an impressive corpus of works in the span of his short lifetime. He is credited with establishing the foundation of cultural psychology and cultural-historical theory, which emphasised that human change and development, particularly the development of our thinking, is influenced by society and culture. This theory has been widely explored in relation to education, and has also provided support for fields such as positive psychology. His work was repressed under the Stalin regime and a lot of his theories were not widely disseminated until the 1980s. Because Vygotsky developed his work in a radically different social, cultural and political context, it is important to appropriate and recontextualise his work for our own time and situation.
The m ain features of Vygotsky’s theory of education
Like Piaget , Vygotsky emphasised learning as a meaning-making process, although Vygotsky’s cultural-historical approach is characterised by an insistence that human thinking be understood as both an individual and a socio-cultural phenomenon. While he emphasised social, cultural and historical influences on thinking, Vygotsky also maintained a focus on the individual, with his concept of the zone of proximal development , for example (more on this below).
According to Vygotsky’s theory:
Human action is situated in sociocultural, historical settings, and is mediated by tools and signs . All human actions, including thinking, are mediated by material and symbolic objects (tools and signs) that are culturally constructed and socially used. For example, a verbal explanation of a word meaning, or a procedure for a science experiment, works to mediate (intervene in, influence or change) the student’s thinking. The ways in which students think, solve problems, and use concepts are related to their social and cultural context.
Students ’ development ha s a social origin. Vygotsky saw new cognitive capabilities for students, particularly higher mental processes such as problem-solving, logic and concept formation, as emerging first in interaction with others before being taken up by the student independently. For example, a teacher may help a student to solve a mathematical word problem by working through a set of questions to identify what they know and what they need to learn. The next time the student encounters a similar problem, he or she runs through the same questions in their mind, and the questions become a tool for the student’s thinking.
Mediation of various kinds is crucial for human pyschological and social development. All kinds of social processes and cultural resources are used by individuals in their thinking, and schools are a primary source for introducing students to many different mediational means, such as tools and artefacts, symbol systems, and specialised discourse. For example, discipline-specific concepts and language can help students to talk about the phenomena they notice in science, while number grids and squares in mathematics are tools that can help children to see the relationships between numbers more easily.
Learning precedes development . Vygotsky saw learning as leading development (rather than being dependent on it). Learning creates the conditions needed for development, so teaching should be aimed at the next stage of a child’s development.
Cognitive development at any point in time is limited to a particular range known as the zo ne of proximal development (ZPD) . The ZPD describes the learning an individual student is capable of, which is just beyond what he or she can achieve independently (it is ‘proximal’). Students can only learn knowledge and skills that are in their particular ZPD, and will require the support and help of a more capable peer, the teacher or other mediational resources such as dictionaries, number lines and diagrams.
Scientific concepts are built on, and transform, everyday concepts . Everyday or spontaneous concepts (‘dog’, ‘lizard’) are based on our everyday experience of the world as it appears through our senses, while scientific concepts (‘mammals’, ‘reptiles’) are more systematic, so they can be more easily reflected upon and manipulated. For example, an understanding of the geometric concept of ‘circle’ is based on students’ experiences of coins, wheels, and pizza, but enables a more generalised understanding of the shape.
What e mpirical evidence is there for this philosophy in practice?
Research does show that, generally, mediation improves language and thinking skills 1 . In relation specifically to Vygotsky’s theory of mediation, studies have found that mediating learning can result in improved thinking skills, performance and ability to learn independently 2 , as well as independent problem solving and dealing with new challenges 3 . There is also some evidence to support the use of the ZPD in teaching 4 .
Vygotsky’s i nfluence on teaching practice
Vygotsky’s theory and the idea of the ZPD in particuar have had an impact on a variety of educational practices, including peer tutoring and scaffolding. Vygotsky’s theory can also be considered to have had an impact on key practices such as assessment for learning, while most teachers are aware of capitalising on student’s culture, community and environment as an influence on their learning and development, described here as a ‘funds of knowledge’ approach.
Scaffolding
The aim of scaffolding (which was not Vygotsky’s term) is to offer assistance to ensure that a student is successful in their learning, so it has strong parallels to Vygotsky’s concept of mediation in the ZPD. Rather than adapting the task, scaffolding supports students to complete it in one or more of the following ways:
- modelling the thinking or behaviours needed
- simplifying the student’s role through intervention
- structuring problem-solving by reducing the degrees of freedom
- highlighting the critical features of the task
- providing a sounding board for students to discuss their ideas
At the earliest stages of the ZPD a student needs a sequence of simple and precise instructions. They come to understand tasks and directives as a result of performing them under guidance (‘performance before competence’). As they gain understanding, they may only need encouragement or prompts. In the school setting, scaffolding is often understood as the provision of frameworks (such as opening sentences or a series of headings), worked examples, clear performance criteria, or guidelines to help students to structure their learning. It is important to ensure that these more fixed and predetermined scaffolds are specifically responsive to each student’s ZPD as Vygotsky intended.
Peer tutoring
Peer tutoring enables more students to be supported to reach a higher level of competence than would be possible if the teacher was the only mediator. Students need to be paired carefully, and may need coaching so that they are able to effectively assist each other. Peer tutoring might also involve students having different roles in the same task. For example, in learning a second language, it might be possible to pair a student who has strengths in vocabulary with a student who has strengths in grammar.
Funds of knowledge
A ‘funds of knowledge’ approach to teaching establishes social relationships with families to facilitate an understanding of how students’ home lives can help mediate their learning in the classroom. Funds of knowledge are the strategies, adaptations and knowledge that families develop to assist them in their daily lives, and which can be drawn upon as a basis for learning academic concepts and procedures in the classroom. For example, if teachers learn that a family is involved in the cultivation and gathering of medicinal plants, they might relate these botanical funds of knowledge to the classroom science curriculum.
Assessment for learning ( AfL )
AfL is a pedagogy in which teachers and students evaluate the student’s current performance together, and agree strategies to address gaps in what they need to know or be doing to perform better. As such it represents an example of the student moving ahead of their current level of performance with the support of a more knowledgeable adult. However, where AfL conversations are constrained within pre-set goals and targets regulated by summative assessment routines and the need to help students to achieve particular grades and outcomes, there may be less overlap with Vygotsky’s concept of ZPD, which is more individual, co-constructed and open to the intentions and motivations of the learner.
Reciprocal teaching
Reciprocal teaching is related to the concept of the ZPD. It is used in reading instruction, where a portion of text is read aloud or silently, before a ‘learning leader’ helps the group to comprehend what was read by engaging in specific reading strategies (questioning, clarifying, summarising and predicting). The teacher is the first learning leader and models strategy use, before each student has the responsibility of being learning leader. The aim is for students to monitor their own comprehension and to internalise some strategies for improving comprehension.
Dialogic teaching
Vygotsky’s theory has been used to inspire a focus on interactive and collaborative organisations of teaching and learning that encourage students to learn from social interactions with peers and with the teacher. Dialogic teaching focuses on the co-construction of knowledge in social settings where students learn to use specific reasoning and argumentation strategies particular to specific domains of knowledge and to verbally elaborate, compare and discuss their developing concepts.
References & further reading
DeVries, R. (2000). Vygostky, Piaget and education: A reciprocal assimilation of theories and educational practices. New Ideas in Psychology, 18, 187-213.
Flavian, H. (2019). Mediation and thinking development in schools: Theories and practices for educators. Emerald Publishing Ltd.
Moll, L. (2014). L. S. Vygotsky and education. Routledge.
Moore, A. (2012). Teaching and learning: Pedagogy, curriculum and culture. Routledge.
Vygotsky, L. S. (1978). Mind in society: The development of higher psychological processes . Cambridge, MA: Harvard University Press.
Vygotsky, L. S. (1934/1986). Thought and language . Cambridge, MA: MIT Press.
[1] Flavian, 2019.
[2] Flavian, 2019.
[3] Flavian, 2019.
[4] Moll, 2014.
By Dr Vicki Hargraves
PREPARED FOR THE EDUCATION HUB BY
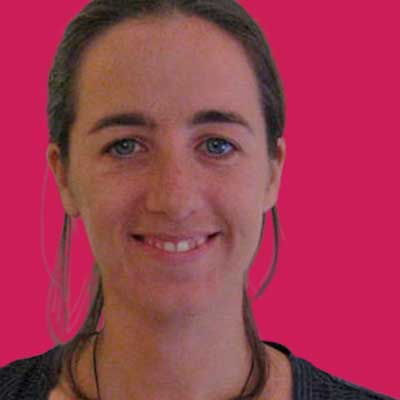
Dr Vicki Hargraves
Vicki runs our early childhood webinar series and also is responsible for the creation of many of our early childhood research reviews. Vicki is a teacher, mother, writer, and researcher living in Marlborough. She recently completed her PhD using philosophy to explore creative approaches to understanding early childhood education. She is inspired by the wealth of educational research that is available and is passionate about making this available and useful for teachers.
Download this resource as a PDF
Please provide your email address and confirm you are downloading this resource for individual use or for use within your school or early childhood education centre only, as per our Terms of Use . Other users should contact us to ask about for permission to use our resources.
Interested in * —Please choose an option— Early childhood education (ECE) Schools Both ECE and schools I agree to abide by The Education Hub's Terms of Use.
Did you find this article useful?
If you enjoyed this content, please consider making a charitable donation.
Become a supporter for as little as $1 a week – it only takes a minute and enables us to continue to provide research-informed content for teachers that is free, high-quality and independent.
Become a supporter
Get unlimited access to all our webinars
Buy a webinar subscription for yourself, your school or centre and enjoy savings of up to 25%, the education hub has changed the way it provides webinar content, to enable us to continue creating our high-quality content for teachers., an annual subscription of just nz$60+gst per person provides access to all our live webinars for a whole year, plus the ability to watch any of the recordings in our archive. alternatively, you can buy access to individual webinars for just $9.95+gst each., we welcome group enrolments, and offer discounts of up to 25%. simply follow the instructions to indicate the size of your group, and we'll calculate the price for you. , unlimited annual subscription.
- All live webinars for 12 months
- Access to our archive of over 80 webinars
- Personalised certificates
- Group savings of up to 25%
The Education Hub’s mission is to bridge the gap between research and practice in education. We want to empower educators to find, use and share research to improve their teaching practice, and then share their innovations. We are building the online and offline infrastructure to support this to improve opportunities and outcomes for students. New Zealand registered charity number: CC54471

We’ll keep you updated
Click here to receive updates on new resources.
Interested in * —Please choose an option— Early childhood education (ECE) Schools Both ECE and schools
Follow us on social media
Like what we do please support us.
© The Education Hub 2024 All rights reserved | Site design: KOPARA
- Terms of use
- Privacy policy
Privacy Overview
Thanks for visiting our site. To show your support for the provision of high-quality research-informed resources for school teachers and early childhood educators, please take a moment to register.
Thanks, Nina
Assignments
- by teachmint@wp
What is assignment in education? Assignment meaning is the tasks given to students by their teachers and tutors to complete in a defined time. They can also be referred to as the work given to someone as a part of learning. Assignments can be in the form of written, practical, art or fieldwork, or even online. Their purpose is to ensure that students understand the subject matter thoroughly.
Generally, students are assigned a task as a part of their homework. The allocation of assignments is not only restricted to a class or subject but this method can be applied at any stage of life. They are a great way to judge the ability and understanding of an individual towards a subject matter.
The culture of providing homework starts from kindergarten in various forms. Small children are asked to read and write what they learn in the class. Students in the higher class are given work as a part of their revision exercise and help them prepare for exams. Individuals doing honors write journals as a part of an assignment that determines their knowledge in that subject.
The structure of assignments depends upon the nature of the task and subject. They must be well-researched, including case studies or examples within a proper framework. These studies are useful for students to achieve the desired examination results. It also helps them to concentrate better on education. Knowing what is assignment in education helps teachers assess students better.
Importance of Assignments
Giving assignments to the students is a crucial part of student assessment. The importance of giving assignments to the students is discussed in detail below:
- Learning practical skills Assignments enable students to develop new skills. In order to complete the assignments, students learn new skills which help them in their academic careers.
- Enhances time management skills Whenever assignments are allocated to the students, a time limit is set by teachers within which the assignment has to be submitted. What is assignment in education enable students to complete their tasks and learn timely submission of work.
- Learning researching skills Students tend to do a lot of research about the questions given in the assignment. Assignment meaning is to help them develop their research skills and come in handy in their future careers.
- Enhance the Writing Caliber Many students have a problem of not being able to put their thoughts into words. Writing assignments can help them develop writing skills and be expressive in real life as well.
Since assignments are deadline-based, they help students take responsibility and manage time. The work assigned to students may be individual or group activities, or both, to develop teamwork in them.
The above-mentioned information gives us a context of assignment meaning and its importance to the students. Assignments provide a basis for the student assessment and should be given timely.
Try Teachmint, an integrated school platform for efficient school management .
Learn more about Teachmint plans here.
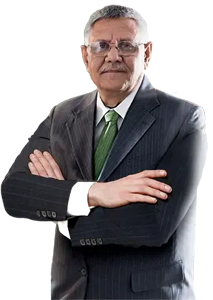
Digitize your school in minutes with Teachmint’s integrated platform
" * " indicates required fields
for Education
- Google Classroom
- Google Workspace Admin
- Google Cloud
Check for missing citations quickly and easily with originality reports
Originality reports use the power of Google Search to help students properly integrate external inspiration into their writing – while making it easy for instructors to check for potential plagiarism.
Help teachers quickly assess authenticity
Compare student work against hundreds of billions of web pages and over 40 million books with a single click
Highlight potential plagiarism and link to external sources within your grading interface
Get answers quickly and avoid manually searching for web matches
Help students turn in their best work
Enable students to check their own work for recommended citations up to three times before submitting
Help students learn to build on outside ideas and properly incorporate them into their work
Run originality reports in multiple languages .
Coming soon Backfill results for your school’s corpus of work in originality reports.
Compare student work without compromising privacy
Compare student-to-student matches against your domain-owned repository of past work when you sign up for the Teaching and Learning Upgrade or Google Workspace for Education Plus
Admins can add or delete files from the school repository manually
You always own your data — it’s our responsibility to keep it more secure
Coming soon Support for Microsoft Word documents in originality reports.
Learn about access to originality reports
Google workspace for education fundamentals.
Instructors using Classroom and Assignments in Google Workspace for Education Fundamentals can enable up to five originality reports per class at no cost.
- Get Google Workspace
Teaching and Learning Upgrade and Education Plus
Instructors with the Teaching and Learning Upgrade or Education Plus licenses get unlimited originality reports and the ability to make student-to-student comparisons on your domain-owned repository of past submissions.
- Contact sales
Explore resources to get up and running
Discover helpful resources to assist you with originality reports and get helpful answers to common questions on originality reports.
Originality reports overview
Learn more about originality reports for educators and students.
- Download overview
How to use originality reports
This tutorial shows how you and your students can use originality reports.
- Watch video
Originality reports help
Explore helpful answers to common questions on originality reports.
Originality reports and privacy
Learn how originality reports protect the privacy of all users, adhering to rigorous compliance standards.
Use originality reports with Classroom
Access originality reports through Classroom, a tool that helps students and teachers organize assignments, boost collaboration, and foster better communication.
- Explore Classroom
Use originality reports with Assignments
Do you have another learning management system? Access originality reports through Assignments, an add-on application for your LMS to distribute, analyze, and grade student work.
- Explore Assignments
Get unlimited access to originality reports with the Teaching and Learning Upgrade and Education Plus
You're now viewing content for a different region..
For content more relevant to your region, we suggest:
Sign up here for updates, insights, resources, and more.
Pardon Our Interruption
As you were browsing something about your browser made us think you were a bot. There are a few reasons this might happen:
- You've disabled JavaScript in your web browser.
- You're a power user moving through this website with super-human speed.
- You've disabled cookies in your web browser.
- A third-party browser plugin, such as Ghostery or NoScript, is preventing JavaScript from running. Additional information is available in this support article .
To regain access, please make sure that cookies and JavaScript are enabled before reloading the page.
Thank you for visiting nature.com. You are using a browser version with limited support for CSS. To obtain the best experience, we recommend you use a more up to date browser (or turn off compatibility mode in Internet Explorer). In the meantime, to ensure continued support, we are displaying the site without styles and JavaScript.
- View all journals
- Explore content
- About the journal
- Publish with us
- Sign up for alerts
- Open access
- Published: 06 August 2024
AI and ethics: Investigating the first policy responses of higher education institutions to the challenge of generative AI
- Attila Dabis ORCID: orcid.org/0000-0003-4924-7664 1 &
- Csaba Csáki ORCID: orcid.org/0000-0002-8245-1002 1
Humanities and Social Sciences Communications volume 11 , Article number: 1006 ( 2024 ) Cite this article
314 Accesses
1 Altmetric
Metrics details
- Science, technology and society
This article addresses the ethical challenges posed by generative artificial intelligence (AI) tools in higher education and explores the first responses of universities to these challenges globally. Drawing on five key international documents from the UN, EU, and OECD, the study used content analysis to identify key ethical dimensions related to the use of generative AI in academia, such as accountability, human oversight, transparency, or inclusiveness. Empirical evidence was compiled from 30 leading universities ranked among the top 500 in the Shanghai Ranking list from May to July 2023, covering those institutions that already had publicly available responses to these dimensions in the form of policy documents or guidelines. The paper identifies the central ethical imperative that student assignments must reflect individual knowledge acquired during their education, with human individuals retaining moral and legal responsibility for AI-related wrongdoings. This top-down requirement aligns with a bottom-up approach, allowing instructors flexibility in determining how they utilize generative AI especially large language models in their own courses. Regarding human oversight, the typical response identified by the study involves a blend of preventive measures (e.g., course assessment modifications) and soft, dialogue-based sanctioning procedures. The challenge of transparency induced the good practice of clear communication of AI use in course syllabi in the first university responses examined by this study.
Similar content being viewed by others
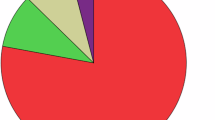
Exploring the impact of artificial intelligence on higher education: The dynamics of ethical, social, and educational implications
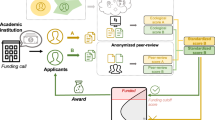
Intersectionality of social and philosophical frameworks with technology: could ethical AI restore equality of opportunities in academia?
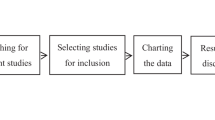
Research on flipped classrooms in foreign language teaching in Chinese higher education
Introduction.
The competition in generative artificial intelligence (AI) ignited by the arrival of ChatGPT, the conversational platform based on a large language model (LLM) in late November 2022 (OpenAI, 2022 ) had a shocking effect even on those who are not involved in the industry (Rudolph et al. 2023 ). Within four months, on 22 March 2023, an open letter was signed by several hundred IT professionals, corporate stakeholders, and academics calling on all AI labs to immediately pause the training of AI systems more powerful than GPT-4 (i.e., those that may trick a human being into believing it is conversing with a peer rather than a machine) for at least six months (Future of Life Institute, 2023 ).
Despite these concerns, competition in generative AI and LLMs does not seem to lose momentum, forcing various social systems to overcome the existential distress they might feel about the changes and the uncertainty of what the future may bring (Roose, 2023 ). Organisations and individuals from different sectors of the economy and various industries are looking for adaptive strategies to accommodate the emerging new normal. This includes lawmakers, international organisations, employers, and employees, as well as academic and higher education institutions (Ray, 2023 ; Wach et al. 2023 ). This fierce competition generates gaps in real-time in everyday and academic life, the latter of which is also trying to make sense of the rapid technological advancement and its effects on university-level education (Perkins, 2023 ). Naturally, these gaps can only be filled, and relevant questions answered much slower by academia, making AI-related research topics timely.
This article aims to reduce the magnitude of these gaps and is intended to help leaders, administrators, teachers, and students better understand the ramifications of AI tools on higher education institutions. It will do so by providing a non-exhaustive snapshot of how various universities around the world responded to generative AI-induced ethical challenges in their everyday academic lives within six-eights months after the arrival of ChatGPT. Thus, the research had asked what expectations and guidelines the first policies introduced into existing academic structures to ensure the informed, transparent, responsible and ethical use of the new tools of generative AI (henceforth GAI) by students and teachers. Through reviewing and evaluating first responses and related difficulties the paper helps institutional decision-makers to create better policies to address AI issues specific to academia. The research reported here thus addressed actual answers to the question of what happened at the institutional (policy) level as opposed to what should happen with the use of AI in classrooms. Based on such a descriptive overview, one may contemplate normative recommendations and their realistic implementability.
Given the global nature of the study’s subject matter, the paper presents examples from various continents. Even though it was not yet a widespread practice to adopt separate, AI-related guidelines, the research focused on universities that had already done so quite early. Furthermore, as best practices most often accrue from the highest-ranking universities, the analysis only considered higher education institutions that were represented among the top 500 universities in the Shanghai Ranking list (containing 3041 Universities at the time), a commonly used source to rank academic excellence. Footnote 1 The main sources of this content analysis are internal documents (such as Codes of Ethics, Academic Regulations, Codes of Practice and Procedure, Guidelines for Students and Teachers or similar policy documents) from those institutions whose response to the GAI challenge was publicly accessible.
The investigation is organised around AI-related ethical dilemmas as concluded from relevant international documents, such as the instruments published by the UN, the EU, and the OECD (often considered soft law material). Through these sources, the study inductively identifies the primary aspects that these AI guidelines mention and can be connected to higher education. Thus it only contains concise references to the main ethical implications of the manifold pedagogical practices in which AI tools can be utilised in the classroom. The paper starts with a review of the challenges posed by AI technology to higher education with special focus on ethical dilemmas. Section 3 covers the research objective and the methodology followed. Section 4 presents the analysis of the selected international documents and establishes a list of key ethical principles relevant in HE contexts and in parallel presents the analysis of the examples distilled from the institutional policy documents and guidelines along that dimension. The paper closes with drawing key conclusions as well as listing limitations and ideas for future research.
Generative AI and higher education: Developments in the literature
General ai-related challenges in the classroom from a historical perspective.
Jacque Ellul fatalistically wrote already in 1954 that the “infusion of some more or less vague sentiment of human welfare” cannot fundamentally alter technology’s “rigorous autonomy”, bringing him to the conclusion that “technology never observes the distinction between moral and immoral use” (Ellul, 1964 , p. 97). Footnote 2 Jumping ahead nearly six decades, the above quote comes to the fore, among others, when evaluating the moral and ethical aspects of the services offered by specific software programs, like ChatGPT. While they might be trained to give ethical answers, these moral barriers can be circumvented by prompt injection (Blalock, 2022 ), or manipulated with tricks (Alberti, 2022 ), so generative AI platforms can hardly be held accountable for the inaccuracy of their responses Footnote 3 or how the physical user who inserted a prompt will make use of the output. Indeed, the AI chatbot is now considered to be a potentially disruptive technology in higher education practices (Farazouli et al. 2024 ).
Educators and educational institution leaders have from the beginning sought solutions on how “to use a variety of the strategies and technologies of the day to help their institutions adapt to dramatically changing social needs” (Miller, 2023 , p. 3). Education in the past had always had high hopes for applying the latest technological advances (Reiser, 2001 ; Howard and Mozejko, 2015 ), including the promise of providing personalised learning or using the latest tools to create and manage courses (Crompton and Burke, 2023 ).
The most basic (and original) educational settings include three components: the blackboard with chalk, the instructor, and textbooks as elementary “educational technologies” at any level (Reiser, 2001 ). Beyond these, one may talk about “educational media” which, once digital technology had entered the picture, have progressed from Computer Based Learning to Learning Management Systems to the use of the Internet, and lately to online shared learning environments with various stages in between including intelligent tutoring system, Dialogue-based Tutoring System, and Exploratory Learning Environment and Artificial Intelligence (Paek and Kim, 2021 ). And now the latest craze is about the generative form of AI often called conversational chatbot (Rudolph et al. 2023 ).
The above-mentioned promises appear to be no different in the case of using generative AI tools in education (Baskara, 2023a ; Mhlanga, 2023 ; Yan et al. 2023 ). The general claim is that GAI chatbots have transformative potential in HE (Mollick and Mollick, 2022 ; Ilieva et al. 2023 ). It is further alleged, that feedback mechanisms supposedly provided by GAI can be used to provide personalised guidance to students (Baskara, 2023b ). Some argue, that “AI education should be expanded and improved, especially by presenting realistic use cases and the real limitations of the technology, so that students are able to use AI confidently and responsibly in their professional future” (Almaraz-López et al. 2023 , p. 1). It is still debated whether the hype is justified, yet the question still remains, how to address the issues arising in the wake of the educational application of GAI tools (Ivanov, 2023 ; Memarian and Doleck, 2023 ).
Generative AI tools, such as their most-known representative, ChatGPT impact several areas of learning and teaching. From the point of view of students, chatbots may help with so-called Self-Regulated or Self-Determined Learning (Nicol and Macfarlane‐Dick, 2006 ; Baskara, 2023b ), where students either dialogue with chatbots or AI help with reviewing student work, even correcting it and giving feedback (Uchiyama et al. 2023 ). There are innovative ideas on how to use AI to support peer feedback (Bauer et al. 2023 ). Some consider that GAI can provide adaptive and personalised environments (Qadir, 2023 ) and may offer personalised tutoring (see, for example, Limo et al. ( 2023 ) on ChatGPT as a virtual tutor for personalized learning experiences). Furthermore, Yan et al. ( 2023 ) lists nine different categories of educational tasks that prior studies have attempted to automate using LLMs: Profiling and labelling (various educational or related content), Detection, Assessment and grading, Teaching support (in various educational and communication activities), Prediction, Knowledge representation, Feedback, Content generation (outline, questions, cases, etc.), Recommendation.
From the lecturers’ point of view, one of the most argued impacts is that assessment practices need to be revisited (Chaudhry et al. 2023 ; Gamage et al. 2023 ; Lim et al. 2023 ). For example, ChatGPT-written responses to exam questions may not be distinguished from student-written answers (Rudolph et al. 2023 ; Farazouli et al. 2024 ). Furthermore, essay-type works are facing special challenges (Sweeney, 2023 ). On the other hand, AI may be utilised to automate a range of educational tasks, such as test question generation, including open-ended questions, test correction, or even essay grading, feedback provision, analysing student feedback surveys, and so on (Mollick and Mollick, 2022 ; Rasul et al. 2023 ; Gimpel et al. 2023 ).
There is no convincing evidence, however, that either lecturers or dedicated tools are able to distinguish AI-written and student-written text with high enough accuracy that can be used to prove unethical behaviour in all cases (Akram, 2023 ). This led to concerns regarding the practicality and ethicality of such innovations (Yan et al. 2023 ). Indeed, the appearance of ChatGPT in higher education has reignited the (inconclusive) debate on the potential and risks associated with AI technologies (Ray, 2023 ; Rudolph et al. 2023 ).
When new technologies appear in or are considered for higher education, debates about their claimed advantages and potential drawbacks heat up as they are expected to disrupt traditional practices and require teachers to adapt to their potential benefits and drawbacks (as collected by Farrokhnia et al. 2023 ). One key area of such debates is the ethical issues raised by the growing accessibility of generative AI and discursive chatbots.
Key ethical challenges posed by AI in higher education
Yan et al. ( 2023 ), while investigating the practicality of AI in education in general, also consider ethicality in the context of educational technology and point out that related debates over the last decade (pre-ChatGPT, so to say), mostly focused on algorithmic ethics, i.e. concerns related to data mining and using AI in learning analytics. At the same time, the use of AI by teachers or, especially, by students has received less attention (or only under the scope or traditional human ethics). However, with the arrival of generative AI chatbots (such as ChatGPT), the number of publications about their use in higher education grew rapidly (Rasul et al. 2023 ; Yan et al. 2023 ).
The study by Chan ( 2023 ) offers a (general) policy framework for higher education institutions, although it focuses on one location and is based on the perceptions of students and teachers. While there are studies that collect factors to be considered for the ethical use of AI in HE, they appear to be restricted to ChatGPT (see, for example, Mhlanga ( 2023 )). Mhlanga ( 2023 ) presents six factors: respect for privacy, fairness, and non-discrimination, transparency in the use of ChatGPT, responsible use of AI (including clarifying its limitations), ChatGPT is not a substitute for human teachers, and accuracy of information. The framework by Chan ( 2023 ) is aimed at creating policies to teach students about GAI and considers three dimensions: pedagogical, governance, and operational. Within those dimensions, ten key areas identified covering ethical concerns such as academic integrity versus academic misconduct and related ethical dilemmas (e.g. cheating or plagiarism), data privacy, transparency, accountability and security, equity in access to AI technologies, critical AI literacy, over-reliance on AI technologies (not directly ethical), responsible use of AI (in general), competencies impeded by AI (such as leadership and teamwork). Baskara ( 2023b ), while also looking at ChatGPT only, considers the following likely danger areas: privacy, algorithmic bias issues, data security, and the potential negative impact of ChatGPT on learners’ autonomy and agency, The paper also questions the possible negative impact of GAI on social interaction and collaboration among learners. Although Yan et al. ( 2023 ) considers education in general (not HE in particular) during its review of 118 papers published since 2017 on the topic of AI ethics in education, its list of areas to look at is still relevant: transparency (of the models used), privacy (related to data collection and use by AI tools), equality (such as availability of AI tools in different languages), and beneficence (e.g. avoiding bias and avoiding biased and toxic knowledge from training data). While systematically reviewing recent publications about AI’s “morality footprint” in higher education, Memarian and Doleck ( 2023 ) consider the Fairness, Accountability, Transparency, and Ethics (FATE) approach as their framework of analyses. They note that “Ethics” appears to be the most used term as it serves as a general descriptor, while the other terms are typically only used in their descriptive sense, and their operationalisation is often lacking in related literature.
Regarding education-related data analytics, Khosravi et al. ( 2022 ) argue that educational technology that involves AI should consider accountability, explainability, fairness, interpretability and safety as key ethical concerns. Ferguson et al. ( 2016 ) also looked at learning analytics solutions using AI and warned of potential issues related to privacy, beneficence, and equality. M.A. Chaudhry et al. ( 2022 ) emphasise that enhancing the comprehension of stakeholders of a new educational AI system is the most important task, which requires making all information and decision processes available to those affected, therefore the key concern is related to transparency according to their arguments.
As such debates continue, it is difficult to identify an established definition of ethical AI in HE. It is clear, however, that the focus should not be on detecting academic misconduct (Rudolph et al. 2023 ). Instead, practical recommendations are required. This is especially true as even the latest studies focus mostly on issues related to assessment practices (Chan, 2023 ; Farazouli et al. 2024 ) and often limit their scope to ChatGPT (Cotton et al. 2024 ) (this specific tool still dominates discourses of LLMs despite the availability of many other solutions since its arrival). At the same time, the list of issues addressed appears to be arbitrary, and most publications do not look at actual practices on a global scale. Indeed, reviews of actual current practices of higher education institutions are rare, and this aspect is not yet the focus of recent HE AI ethics research reports.
As follows from the growing literature and the debate shaping up about the implications of using GAI tools in HE, there was a clear need for a systematic review of how first responses in actual academic policies and guidelines in practice have represented and addressed known ethical principles.
Research objective and methodology
In order to contribute to the debate on the impact of GAI on HE, this study aimed to review how leading institutions had reacted to the arrival of generative AI (such as ChatGPT) and what policies or institutional guidelines they have put in place shortly after. The research intended to understand whether key ethical principles were reflected in the first policy responses of HE institutions and, if yes, how they were handled.
As potential principles can diverge and could be numerous, as well as early guidelines may cover wide areas, the investigation is intended to be based on a few broad categories instead of trying to manage a large set of ideals and goals. To achieve this objective, the research was executed in three steps:
It was started with identifying and collecting general ethical ideals, which were then translated and structured for the context of higher education. A thorough content analysis was performed with the intention to put emphasis on positive values instead of simply focusing on issues or risks and their mitigation.
Given those positive ideals, this research collected actual examples of university policies and guidelines already available: this step was executed from May to July 2023 to find early responses addressing such norms and principles developed by leading HE institutions.
The documents identified were then analysed to understand how such norms and principles had been addressed by leading HE institutions.
As a result, this research managed to highlight and contrast differing practical views, and the findings raise awareness about the difficulties of creating relevant institutional policies. The research considered the ethics of using GAI and not expectations towards their development. The next two sections provide details of the two steps.
Establishing ethical principles for higher education
While the review of relevant ethical and HE literature (as presented above) was not fully conclusive, it highlighted the importance and need for some ideals specific to HE. Therefore, as a first step, this study sought to find highly respected sources of such ethical dimensions by executing a directed content analysis of relevant international regulatory and policy recommendations.
In order to establish what key values and ideas drive the formation of future AI regulations in general, Corrêa et al. ( 2023 ) investigated 200 publications discussing governance policies and ethical guidelines for using AI as proposed by various organisations (including national governments and institutions, civil society and academic organisations, private companies, as well as international bodies). The authors were also interested in whether there are common patterns or missing ideals and norms in this extensive set of proposals and recommendations. As the research was looking for key principles and normative attributes that could form a common ground for the comparison of HE policies, this vast set of documents was used to identify internationally recognised bodies that have potential real influence in this arena and decided to consider the guidelines and recommendations they have put forward for the ethical governance of AI. Therefore, for the purpose of this study, the following sources were selected (some organisations, such as the EU were represented by several bodies):
European Commission ( 2021 ): Regulation of the European Parliament and of the Council Laying Down Harmonised Rules on Artificial Intelligence (Artificial Intelligence Act) and Amending Certain Union Legislative Acts (2021/0106 (COD)) . Footnote 4
European Parliament Committee on Culture and Education ( 2021 ): Report on artificial intelligence in education, culture and the audiovisual sector (2020/2017(INI)) . Footnote 5
High-Level Expert Group on Artificial Intelligence (EUHLEX) ( 2019 ): Ethics Guidelines for Trustworthy AI . Footnote 6
UNESCO ( 2022 ): Recommendation on the Ethics of Artificial Intelligence (SHS/BIO/PI/2021/1) . Footnote 7
OECD ( 2019 ): Recommendation of the Council on Artificial Intelligence (OECD/LEGAL/0449) . Footnote 8
The ethical dilemmas established by these international documents (most of which is considered soft law material) were then used to inductively identify the primary aspects around which the investigation of educational AI principles may be organised.
Among the above documents, the EUHLEX material is the salient one as it contains a Glossary that defines and explains, among others, the two primary concepts that will be used in this paper: “artificial intelligence” and “ethics”. As this paper is, to a large extent, based on the deducted categorisation embedded in these international documents, it will follow suit in using the above terms as EUHLEX did, supporting it with the definitions contained in the other four referenced international documents. Consequently, artificial intelligence (AI) systems are referred to in this paper as software and hardware systems designed by humans that “act in the physical or digital dimension by perceiving their environment through data acquisition, interpreting the collected structured or unstructured data, reasoning on the knowledge, or processing the information, derived from this data and deciding the best action(s) to take to achieve the given goal” (EUHLEX, 2019 ). With regards to ethics, the EUHLEX group defines this term, in general as an academic discipline which is a subfield of philosophy, dealing with questions like “What is a good action?”, “What is the value of a human life?”, “What is justice?”, or “What is the good life?”. It also mentions that academia distinguishes four major fields: (i) Meta-ethics, (ii) normative ethics, (iii) descriptive ethics, and (iv) applied ethics ” (EUHLEX, 2019 , p. 37). Within these, AI ethics belongs to the latter group of applied ethics that focuses on the practical issues raised by the design, development, implementation, and use of AI systems. By extension, the application of AI systems in higher education also falls under the domain of applied ethics.
The selection of sample universities
The collection of cases started with the AI guidelines compiled by the authors as members of the AI Committee at their university from May to July 2023. The AI Committee consisted of 12 members and investigated over 150 cases to gauge international best practices of GAI use in higher education when formulating a policy recommendation for their own university leadership. Given the global nature of the subject matter, examples from various continents were collected. From this initial pool authors narrowed the scope to the Top 500 higher education institutions of the Shanghai Ranking list for this study, as best practices most often accrue from the highest-ranking universities. Finally, only those institutions were included which, at the time of data collection, have indeed had publicly available policy documents or guidelines with clearly identifiable ethical considerations (such as relevant internal documents, Codes of Ethics, Academic Regulations, Codes of Practice and Procedure, or Guidelines for Students and Teachers). By the end of this selection process, 30 samples proved to be substantiated enough to be included in this study (presented in Table 1 ).
All documents were contextually analysed and annotated by both authors individually looking for references or mentions of ideas, actions or recommendations related to the ethical principles identified during the first step of the research. These comments were then compared and commonalities analysed regarding the nature and goal of the ethical recommendation.
Principles and practices of responsible use of AI in higher education
Ai-related ethical codes forming the base of this investigation.
A common feature of the selected AI ethics documents issued by international organisations is that they enumerate a set of ethical principles based on fundamental human values. The referenced international documents have different geographical- and policy scopes, yet they overlap in their categorisation of the ethical dimensions relevant to this research, even though they might use discrepant language to describe the same phenomenon (a factor we took into account when establishing key categories). For example, what EUHLEX dubs as “Human agency and oversight” is addressed by UNESCO under the section called “Human oversight and determination”, yet they essentially cover the same issues and recommended requirements. Among the many principles enshrined in these documents, the research focuses on those that can be directly linked to the everyday education practices of universities in relation to AI tools, omitting those that, within this context, are less situation-dependent and should normally form the overarching basis of the functioning of universities at all times, such as: respecting human rights and fundamental freedoms, refraining from all forms of discrimination, the right to privacy and data protection, or being aware of environmental concerns and responsibilities regarding sustainable development. As pointed out by Nikolinakos ( 2023 ), such principles and values provide essential guidance not only for development but also during the deployment and use of AI systems. Synthesising the common ethical codes in these instruments has led to the following cluster of ethical principles that are directly linked to AI-related higher education practices:
Accountability and responsibility;
Human agency and oversight;
Transparency and explainability
Inclusiveness and diversity.
The following subsections will give a comprehensive definition of these ethical areas and relate them to higher education expectations. Each subsection will first explain the corresponding ethical cluster, then present the specific university examples, concluding with a summary of the identified best practice under that particular cluster.
Accountability and responsibility
Definition in ethical codes and relevance.
The most fundamental requirements, appearing in almost all relevant documents, bring forward the necessity that mechanisms should be implemented to ensure responsibility and accountability for AI systems and their outcomes. These cover expectations both before and after their deployment, including development and use. They entail the basic requirements of auditability (i.e. the enablement of the assessment of algorithms), clear roles in the management of data and design processes (as a means for contributing to the trustworthiness of AI technology), the minimalisation and reporting of negative impacts (focusing on the possibility of identifying, assessing, documenting and reporting on the potential negative impacts of AI systems), as well as the ability of redress (understood as the capability to utilise mechanisms that offer legal and practical remedy when unjust adverse impact occurs) (EUHLEX, 2019 , pp. 19–20).
Additionally, Points 35–36 of the UNESCO recommendations remind us that it is imperative to “attribute ethical and legal responsibility for any stage of the life cycle of AI systems, as well as in cases of remedy related to AI systems, to physical persons or to existing legal entities. AI system can never replace ultimate human responsibility and accountability” (UNESCO, 2022 , p. 22).
The fulfilment of this fundamental principle is also expected from academic authors, as per the announcements of some of the largest publishing houses in the world. Accordingly, AI is not an author or co-author, Footnote 9 and AI-assisted technologies should not be cited as authors either, Footnote 10 given that AI-generated content cannot be considered capable of initiating an original piece of research without direction from human authors. The ethical guidelines of Wiley ( 2023 ) stated that ”[AI tools] also cannot be accountable for a published work or for research design, which is a generally held requirement of authorship, nor do they have legal standing or the ability to hold or assign copyright.” Footnote 11 This research angle carries over to teaching as well since students are also expected to produce outputs that are the results of their own work. Furthermore, they also often do their own research (such as literature search and review) in support of their projects, homework, thesis, and other forms of performance evaluation.
Accountability and responsibility in university first responses
The rapidly changing nature of the subject matter poses a significant challenge for scholars to assess the state of play of human responsibility. This is well exemplified by the reversal of hearts by some Australian universities (see Rudolph et al. ( 2023 ) quoting newspaper articles) who first disallowed the use of AI by students while doing assignments, just to reverse that decision a few months later and replace it by a requirement of disclosing the use of AI in homeworks. Similarly, Indian governments have been oscillating between a non-regulatory approach to foster an “innovation-friendly environment” for their universities in the summer of 2023 (Liu, 2023 ), only to roll back on this pledge a few months later (Dhaor, 2023 ).
Beyond this regulatory entropy, a fundamental principle enshrined in university codes of ethics across the globe is that students need to meet existing rules of scientific referencing and authorship. Footnote 12 In other words, they should refrain from any form of plagiarism in all their written work (including essays, theses, term papers, or in-class presentations). Submitting any work and assessments created by someone or something else (including AI-generated content) as if it was their own usually amounts to either a violation of scientific referencing, plagiarism or is considered to be a form of cheating (or a combination of these), depending on the terminology used by the respective higher education institution.
As a course description of Johns Hopkins puts it, “academic honesty is required in all work you submit to be graded …., you must solve all homework and programming assignments without the help of outside sources (e.g., GAI tools)” (Johns Hopkins University, 2023 ).
The Tokyo Institute of Technology applies a more flexible approach, as they “trust the independence of the students and expect the best use” of AI systems from them based on good sense and ethical standards. They add, however, that submitting reports that rely almost entirely on the output of GenAI is “highly improper, and its continued use is equivalent to one’s enslavement to the technology” (Tokyo Institute of Technology, 2023 ).
In the case of York University, the Senate’s Academic Standards, Curriculum, and Pedagogy Committee clarified in February 2023 that students are not authorised to use “text-, image-, code-, or video-generating AI tools when completing their academic work unless explicitly permitted by a specific instructor in a particular course” (York University Senate, 2023 ).
In the same time frame (6 February 2023), the University of Oxford stated in a guidance material for staff members that “the unauthorised use of AI tools in exams and other assessed work is a serious disciplinary offence” not permitted for students (University of Oxford, 2023b ).
Main message and best practice: honesty and mutual trust
In essence, students are not allowed to present AI-generated content as their own, Footnote 13 and they should have full responsibility and accountability for their own papers. Footnote 14 This is in line with the most ubiquitous principle enshrined in almost all university guidelines, irrespective of AI, that students are expected to complete their tasks based on their own knowledge and skills obtained throughout their education.
Given that the main challenge here is unauthorised use and overreliance on GAI platforms, the best practice answer is for students to adhere to academic honesty and integrity, scientific referencing standards, existing anti-plagiarism rules, and complete university assignments without fully relying on GAI tools, using, first and foremost, their own skills. The only exception is when instructed otherwise by their professors. By extension, preventing overuse and unauthorised use of AI assists students in avoiding undermining their own academic capacity-building efforts.
Human agency and oversight
AI systems have the potential to manipulate and influence human behaviour in ways that are not easily detectable. AI systems must, therefore, follow human-centric design principles and leave meaningful opportunities for human choice and intervention. Such systems should not be able to unjustifiably subordinate, coerce, deceive, manipulate, condition or herd humans (EUHLEX, 2019 , p. 16).
Human oversight thus refers to the capability for human intervention in every decision cycle of the AI system and the ability of users to make informed, autonomous decisions regarding AI systems. This encompasses the ability to choose not to use an AI system in a particular situation or to halt AI-related operations via a “stop” button or a comparable procedure in case the user detects anomalies, dysfunctions and unexpected performance from AI tools (European Commission, 2021 , Art. 14).
The sheer capability of active oversight and intervention vis-á-vis GAI systems is strongly linked to ethical responsibility and legal accountability. As Liao puts it, “the sufficient condition for human beings being rightsholders is that they have a physical basis for moral agency.” (Liao, 2020 , pp. 496–497). Wagner complemented this with the essential point that entity status for non-human actors would help to shield other parties from liability, i.e., primarily manufacturers and users (Wagner, 2018 ). This, in turn, would result in risk externalisation, which serves to minimise or relativise a person’s moral accountability and legal liability associated with wrongful or unethical acts.
Users, in our case, are primarily students who, at times, might be tempted to make use of AI tools in an unethical way, hoping to fulfil their university tasks faster and more efficiently than they could without these.
Human agency and oversight in university first responses
The crucial aspect of this ethical issue is the presence of a “stop” button or a similar regulatory procedure to streamline the operation of GAI tools. Existing university guidelines in this question point clearly in the direction of soft sanctions, if any, given the fact that there is a lack of evidence that AI detection platforms are effective and reliable tools to tell apart human work from AI-generated ones. Additionally, these tools raise some significant implications for privacy and data security issues, which is why university guidelines are particularly cautious when referring to these. Accordingly, the National Taiwan University, the University of Toronto, the University of Waterloo, the University of Miami, the National Autonomous University of Mexico, and Yale, among others, do not recommend the use of AI detection platforms in university assessments. The University of Zürich further added the moral perspective in a guidance note from 13 July 2023, that “forbidding the use of undetectable tools on unsupervised assignments or demanding some sort of honour code likely ends up punishing the honest students” (University of Zürich, 2023 ). Apart from unreliability, the University of Cape Town also drew attention in its guide for staff that AI detection tools may “disproportionately flag text written by non-first language speakers as AI-generated” (University of Cape Town, 2023 , p. 8).
Macquarie University took a slightly more ambiguous stance when they informed their staff that, while it is not “proof” for anything, an AI writing detection feature was launched within Turnitin as of 5 April 2023 (Hillier, 2023 ), claiming that the software has a 97% detection rate with a 1% false positive rate in the tests that they had conducted (Turnitin, 2023 ). Apart from these, Boston University is among the few examples that recommend employing AI detection tools, but only in a restricted manner to ”evaluate the degree to which AI tools have likely been employed” and not as a source for any punitive measures against students (University of Boston, 2023 ). Remarkably, they complement the above with suggestions for a merit-based scoring system, whereby instructors shall treat work by students who declare no use of AI tools as the baseline for grading. A lower baseline is suggested for students who declare the use of AI tools (depending on how extensive the usage was), and for the bottom of this spectrum, the university suggests imposing a significant penalty for low-energy or unreflective reuse of material generated by AI tools and assigning zero points for merely reproducing the output from AI platforms.
A discrepant approach was adopted at the University of Toronto. Here, if an instructor indicates that the use of AI tools is not permitted on an assessment, and a student is later found to have used such a tool nevertheless, then the instructor should consider meeting with the student as the first step of a dialogue-based process under the Code of Behaviour on Academic Matters (the same Code, which categorises the use of ChatGPT and other such tools as “unauthorised aid” or as “any other form of cheating” in case, an instructor specified that no outside assistance was permitted on an assignment) (University of Toronto, 2019 ).
More specifically, Imperial College London’s Guidance on the Use of Generative AI tools envisages the possibility of inviting a random selection of students to a so-called “authenticity interview” on their submitted assignments (Imperial College London, 2023b ). This entails requiring students to attend an oral examination of their submitted work to ensure its authenticity, which includes questions about the subject or how they approached their assignment.
As a rare exception, the University of Helsinki represents one of the more rigorous examples. The “Guidelines for the Use of AI in Teaching at the University of Helsinki” does not lay down any specific procedures for AI-related ethical offences. On the contrary, as para. 7 stipulates the unauthorised use of GAI in any course examination “constitutes cheating and will be treated in the same way as other cases of cheating” (University of Helsinki, 2023 ). Footnote 15
Those teachers who are reluctant to make AI tools a big part of their courses should rather aim to develop course assessment methods that can plausibly prevent the use of AI tools instead of attempting to filter these afterwards. Footnote 16 For example, the Humboldt-Universität zu Berlin instructs that, if possible, oral or practical examinations or written examinations performed on-site are recommended as alternatives to “classical” written home assignments (Humboldt-Universität zu Berlin, 2023a ).
Monash University also mentions some examples in this regard (Monash University, 2023a ), such as: asking students to create oral presentations, videos, and multimedia resources; asking them to incorporate more personal reflections tied to the concepts studied; implementing programmatic assessment that focuses on assessing broader attributes of students, using multiple methods rather than focusing on assessing individual kinds of knowledge or skills using a single assessment method (e.g., writing an essay).
Similarly, the University of Toronto suggest instructors to: ask students to respond to a specific reading that is very new and thus has a limited online footprint; assign group work to be completed in class, with each member contributing; or ask students to create a first draft of an assignment by hand, which could be complemented by a call to explain or justify certain elements of their work (University of Toronto, 2023 ).
Main message and best practice: Avoiding overreaction
In summary, the best practice that can be identified under this ethical dilemma is to secure human oversight through a blend of preventive measures (e.g. a shift in assessment methods) and soft sanctions. Given that AI detectors are unreliable and can cause a series of data privacy issues, the sanctioning of unauthorised AI use should happen on a “soft basis”, as part of a dialogue with the student concerned. Additionally, universities need to be aware and pay due attention to potentially unwanted rebound effects of bona fide measures, such as the merit-based scoring system of the University of Boston. In that case, using different scoring baselines based on the self-declared use of AI could, in practice, generate incentives for not declaring any use of AI at all, thereby producing counter-effective results.
While explainability refers to providing intelligible insight into the functioning of AI tools with a special focus on the interplay between the user’s input and the received output, transparency alludes to the requirement of providing unambiguous communication in the framework of system use.
As the European Commission’s Regulation proposal ( 2021 ) puts it under subchapter 5.2.4., transparency obligations should apply for systems that „(i) interact with humans, (ii) are used to detect emotions or determine association with (social) categories based on biometric data, or (iii) generate or manipulate content (‘deep fakes’). When persons interact with an AI system or their emotions or characteristics are recognised through automated means, people must be informed of that circumstance. If an AI system is used to generate or manipulate image, audio or video content that appreciably resembles authentic content, there should be an obligation to disclose that the content is generated through automated means, subject to exceptions for legitimate purposes (law enforcement, freedom of expression). This allows persons to make informed choices or step back from a given situation.”
People (in our case, university students and teachers) should, therefore, be fully informed when a decision is influenced by or relies on AI algorithms. In such instances, individuals should be able to ask for further explanation from the decision-maker using AI (e.g., a university body). Furthermore, individuals should be afforded the choice to present their case to a dedicated representative of the organisation in question who should have the power to reviset the decision and make corrections if necessary (UNESCO, 2022 , p. 22). Therefore, in the context of courses and other related education events, teachers should be clear about their utilisation of AI during the preparation of the material. Furthermore, instructors must unambiguously clarify ethical AI use in the classroom. Clear communication is essential about whether students have permission to utilise AI tools during assignments and how to report actual use.
As both UN and EU sources point out, raising awareness about and promoting basic AI literacy should be fostered as a means to empower people and reduce the digital divides and digital access inequalities resulting from the broad adoption of AI systems (EUHLEX, 2019 , p. 23; UNESCO, 2022 , p. 34).
Transparency and explainability in university first responses
The implementation of this principle seems to revolve around the challenge of decentralisation of university work, including the respect for teachers’ autonomy.
Teachers’ autonomy entails that teachers can decide if and to what extent they will allow their students to use AI platforms as part of their respective courses. This, however, comes with the essential corollary, that they must clearly communicate their decision to both students and university management in the course syllabus. To support transparency in this respect, many universities decided to establish 3-level- or 4-level admissibility frameworks (and even those who did not establish such multi-level systems, e.g., the University of Toronto, urge instructors to explicitly indicate in the course syllabus the expected use of AI) (University of Toronto, 2023 ).
The University of Auckland is among the universities that apply a fully laissez passer laissez-faire approach in this respect, meaning that there is a lack of centralised guidance or recommendations on this subject. They rather confer all practical decision-making of GAI use on course directors, adding that it is ultimately the student’s responsibility to correctly acknowledge the use of Gen-AI software (University of Auckland, 2023 ). Similarly, the University of Helsinki gives as much manoeuvring space to their staff as to allow them to change the course of action during the semester. As para 1 of their earlier quoted Guidelines stipulates, teachers are responsible for deciding how GAI can be used on a given course and are free to fully prohibit their use if they think it impedes the achievement of the learning objectives.
Colorado State University, for example, provides its teachers with 3 types of syllabus statement options (Colorado State University, 2023 ): (a) the prohibitive statement: whereby any work created, or inspired by AI agents is considered plagiarism and will not be tolerated; (b) the use-with-permission statement: whereby generative AI can be used but only as an exception and in line with the teachers further instruction, and (c) the abdication statement: where the teacher acknowledges that the course grade will also be a reflection of the students ability to harness AI technologies as part of their preparation for their future in a workforce that will increasingly require AI-literacy.
Macquarie University applies a similar system and provides it’s professors with an Assessment Checklist in which AI use can be either “Not permitted” or “Some use permitted” (meaning that the scope of use is limited while the majority of the work should be written or made by the student.), or “Full use permitted (with attribution)”, alluding to the adaptive use of AI tools, where the generated content is edited, mixed, adapted and integrated into the student’s final submission – with attribution of the source (Macquarie University, 2023 ).
The same approach is used at Monash University where generative AI tools can be: (a) used for all assessments in a specific unit; (b) cannot be used for any assessments; (c) some AI tools may be used selectively (Monash University, 2023b ).
The University of Cape Town (UCT) applies a 3-tier system not just in terms of the overall approach to the use or banning of GAI, but also with regard to specific assessment approaches recommended to teachers. As far as the former is concerned, they differentiate between the strategies of: (a) Avoiding (reverting to in-person assessment, where the use of AI isn’t possible); (b) Outrunning (devising an assessment that AI cannot produce); and (c) Embracing (discussing the appropriate use of AI with students and its ethical use to create the circumstances for authentic assessment outputs). The assessment possibilities, in turn, are categorised into easy, medium, and hard levels. Easy tasks include, e.g., generic short written assignments. Medium level might include examples such as personalised or context-based assessments (e.g. asking students to write to a particular audience whose knowledge and values must be considered or asking questions that would require them to give a response that draws from concepts that were learnt in class, in a lab, field trip…etc). In contrast, hard assessments include projects involving real-world applications, synchronous oral assessments, or panel assessments (University of Cape Town, 2023 ).
4-tier-systems are analogues. The only difference is that they break down the “middle ground”. Accordingly, the Chinese University of Hong Kong clarifies that Approach 1 (by default) means the prohibition of all use of AI tools; Approach 2 entails using AI tools only with prior permission; Approach 3 means using AI tools only with explicit acknowledgement; and Approach 4 is reserved for courses in which the use of AI tools is freely permitted with no acknowledgement needed (Chinese University of Hong Kong, 2023 ).
Similarly, the University of Delaware provides course syllabus statement examples for teachers including: (1) Prohibiting all use of AI tools; (2) Allowing their use only with prior permission; (3) Allow their use only with explicit acknowledgement; (4) Freely allow their use (University of Delaware, 2023 ).
The Technical University of Berlin also proposes a 4-tier system but uses a very different logic based on the practical knowledge one can obtain by using GAI. Accordingly, they divide AI tools as used to: (a) acquire professional competence; (b) learn to write scientifically; (c) be able to assess AI tools and compare them with scientific methods; d) professional use of AI tools in scientific work. Their corresponding guideline even quotes Art. 5 of the German Constitution referencing the freedom of teaching ( Freiheit der Lehre ), entailing that teachers should have the ability to decide for themselves which teaching aids they allow or prohibit. Footnote 17
This detailed approach, however, is rather the exception. According to the compilation on 6 May 2023 by Solis ( 2023 ), among the 100 largest German universities, 2% applied a general prohibition on the use of ChatGPT, 23% granted partial permission, 12% generally permitted its use, while 63% of the universities had none or only vague guidelines in this respect.
Main message and best practice: raising awareness
Overall, the best practice answer to the dilemma of transparency is the internal decentralisation of university work and the application of a “bottom-up” approach that respects the autonomy of university professors. Notwithstanding the potential existence of regulatory frameworks that set out binding rules for all citizens of an HE institution, this means providing university instructors with proper manoeuvring space to decide on their own how they would like to make AI use permissible in their courses, insofar as they communicate their decision openly.
Inclusiveness and diversity
Para. 34 of the Report by the European Parliament Committee on Culture and Education ( 2021 ) highlights that inclusive education can only be reached with the proactive presence of teachers and stresses that “AI technologies cannot be used to the detriment or at the expense of in-person education, as teachers must not be replaced by any AI or AI-related technologies”. Additionally, para. 20 of the same document highlights the need to create diverse teams of developers and engineers to work alongside the main actors in the educational, cultural, and audiovisual sectors in order to prevent gender or social bias from being inadvertently included in AI algorithms, systems, and applications.
This approach also underlines the need to consider the variety of different theories through which AI has been developed as a precursor to ensuring the application of the principle of diversity (UNESCO, 2022 , pp. 33–35), and it also recognises that a nuanced answer to AI-related challenges is only possible if affected stakeholders have an equal say in regulatory and design processes. An idea closely linked to the principle of fairness and the pledge to leave no one behind who might be affected by the outcome of using AI systems (EUHLEX, 2019 , pp. 18–19).
Therefore, in the context of higher education, the principle of inclusiveness aims to ensure that an institution provides the same opportunities to access the benefits of AI technologies for all its students, irrespective of their background, while also considering the particular needs of various vulnerable groups potentially marginalised based on age, gender, culture, religion, language, or disabilities. Footnote 18 Inclusiveness also alludes to stakeholder participation in internal university dialogues on the use and impact of AI systems (including students, teachers, administration and leadership) as well as in the constant evaluation of how these systems evolve. On a broader scale, it implies communication with policymakers on how higher education should accommodate itself to this rapidly changing environment (EUHLEX, 2019 , p. 23; UNESCO, 2022 , p. 35).
Inclusiveness and diversity in university first responses
Universities appear to be aware of the potential disadvantages for students who are either unfamiliar with GAI or who choose not to use it or use it in an unethical manner. As a result, many universities thought that the best way to foster inclusive GAI use was to offer specific examples of how teachers could constructively incorporate these tools into their courses.
The University of Waterloo, for example, recommends various methods that instructors can apply on sight, with the same set of tools for all students during their courses, which in itself mitigates the effects of any discrepancies in varying student backgrounds (University of Waterloo, 2023 ): (a) Give students a prompt during class, and the resulting text and ask them to critique and improve it using track changes; (b) Create two distinct texts and have students explain the flaws of each or combine them in some way using track changes; (c) Test code and documentation accuracy with a peer; or (d) Use ChatGPT to provide a preliminary summary of an issue as a jumping-off point for further research and discussion.
The University of Pittsburgh ( 2023 ) and Monash added similar recommendations to their AI guidelines (Monash University, 2023c ).
The University of Cambridge mentions under its AI-deas initiative a series of projects aimed to develop new AI methods to understand and address sensory, neural or linguistic challenges such as hearing loss, brain injury or language barriers to support people who find communicating a daily challenge in order to improve equity and inclusion. As they put it, “with AI we can assess and diagnose common language and communication conditions at scale, and develop technologies such as intelligent hearing aids, real-time machine translation, or other language aids to support affected individuals at home, work or school.” (University of Cambridge, 2023 ).
The homepage of the Technical University of Berlin (Technische Universität Berlin) displays ample and diverse materials, including videos Footnote 19 and other documents, as a source of inspiration for teachers on how to provide an equitable share of AI knowledge for their students (Glathe et al. 2023 ). More progressively, the university’s Institute of Psychology offers a learning modul called “Inclusive Digitalisation”, available for students enrolled in various degree programmes to understand inclusion and exclusion mechanisms in digitalisation. This modul touches upon topics such as barrier-free software design, mechanisms and reasons for digitalised discrimination or biases in corporate practices (their homepage specifically alludes to the fact that input and output devices, such as VR glasses, have exclusively undergone testing with male test subjects and that the development of digital products and services is predominantly carried out by men. The practical ramifications of such a bias result in input and output devices that are less appropriate for women and children) (Technische Universität Berlin, 2023 ).
Columbia recommends the practice of “scaffolding”, which is the process of breaking down a larger assignment into subtasks (Columbia University, 2023 ). In their understanding, this method facilitates regular check-ins and enables students to receive timely feedback throughout the learning process. Simultaneously, the implementation of scaffolding helps instructors become more familiar with students and their work as the semester progresses, allowing them to take additional steps in the case of students who might need more attention due to their vulnerable backgrounds or disabilities to complete the same tasks.
The Humboldt-Universität zu Berlin, in its Recommendations, clearly links the permission of GAI use with the requirement of equal accessibility. They remind that if examiners require students to use AI for an examination, “students must be provided with access to these technologies free of charge and in compliance with data protection regulations” (Humboldt-Universität zu Berlin, 2023b ).
Concurringly, the University of Cape Town also links inclusivity to accessibility. As they put it, “there is a risk that those with poorer access to connectivity, devices, data and literacies will get unequal access to the opportunities being provided by AI”, leading to the conclusion that the planning of the admissible use of GAI on campus should be cognizant of access inequalities (University of Cape Town, 2023 ). They also draw their staff’s attention to a UNESCO guide material containing useful methods to incorporate ChatGPT into the course, including methods such as the “Socratic opponent” (AI acts as an opponent to develop an argument), the “study buddy” (AI helps the student reflect on learning material) or the “dynamic assessor” (AI provides educators with a profile of each student’s current knowledge based on their interactions with ChatGPT) (UNESCO International Institute for Higher Education in Latin America and the Caribbean, 2023 ).
Finally, the National Autonomous University of Mexico’s Recommendations suggest using GAI tools, among others, for the purposes of community development. They suggest that such community-building activities, whether online or in live groups, kill two birds with one stone. On the one hand, they assist individuals in keeping their knowledge up to date with a topic that is constantly evolving, while it offers people from various backgrounds the opportunity to become part of communities in the process where they can share their experiences and build new relations (National Autonomous University of Mexico, 2023 ).
Main message and best practice: Proactive central support and the pledge to leave no one behind
To conclude, AI-related inclusivity for students is best fostered if the university does not leave its professors solely to their own resources to come up with diverging initiatives. The best practice example for this dilemma thus lies in a proactive approach that results in the elaboration of concrete teaching materials (e.g., subscriptions to AI tools to ensure equal accessibility for all students, templates, video tutorials, open-access answers to FAQs…etc.), specific ideas, recommendations and to support specialised programmes and collaborations with an inclusion-generating edge. With centrally offered resources and tools institutions seem to be able to ensure accessability irrespective of students’ background and financial abilities.
Discussion of the First Responses
While artificial intelligence and even its generative form has been around for a while, the arrival of application-ready LLMs – most notably ChatGPT has changed the game when it comes to grammatically correct large-scale and content-specific text generation. This has invoked an immediate reaction from the higher education community as the question arose as to how it may affect various forms of student performance evaluation (such as essay and thesis writing) (Chaudhry et al. 2023 ; Yu, 2023 ; Farazouli et al. 2024 ).
Often the very first reaction (a few months after the announcement of the availability of ChatGPT) was a ban on these tools and a potential return to hand-written evaluation and oral exams. In the institutions investigated under this research, notable examples may be most Australian universities (such as Monash) or even Oxford. On the other hand, even leading institutions have immediately embraced this new tool as a great potential helper of lecturers – the top name here being Harvard. Very early responses thus ranged widely – and have changed fast over the first six-eight months “post-ChatGPT”.
Over time responses from the institutions investigated started to put out clear guidelines and even created dedicated policies or modified existing ones to ensure a framework of acceptable use. The inspiration leading these early regulatory efforts was influenced by the international ethics documents reviewed in this paper. Institutions were aware of and relied on those guidelines. The main goal of this research was to shed light on the questions of how much and in what ways they took them on board regarding first responses. Most first reactions were based on “traditional” AI ethics and understanding of AI before LLMs and the generative revolution. First responses by institutions were not based on scientific literature or arguments from journal publications. Instead, as our results demonstrated it was based on publicly available ethical norms and guidelines published by well-known international organizations and professional bodies.
Conclusions, limitations and future research
Ethical dilemmas discussed in this paper were based on the conceptualisation embedded in relevant documents of various international fora. Each ethical dimension, while multifaceted in itself, forms a complex set of challenges that are inextricably intertwined with one another. Browsing university materials, the overall impression is that Universities primarily aim to explore and harness the potential benefits of generative AI but not with an uncritical mindset. They are focusing on the opportunities while simultaneously trying to address the emerging challenges in the field.
Accordingly, the main ethical imperative is that students must complete university assignments based on the knowledge and skills they acquired during their university education unless their instructors determine otherwise. Moral and legal responsibility in this regard always rests with human individuals. AI agents possess neither the legal standing nor the physical basis for moral agency, which makes them incapable of assuming such responsibilities. This “top-down” requirement is most often complemented by the “bottom-up” approach of providing instructors with proper maneuvering space to decide how they would like to make AI use permissible in their courses.
Good practice in human oversight could thus be achieved through a combination of preventive measures and soft, dialogue-based procedures. This latter category includes the simple act of teachers providing clear, written communications in their syllabi and engaging in a dialogue with their students to provide unambiguous and transparent instructions on the use of generative AI tools within their courses. Additionally, to prevent the unauthorised use of AI tools, changing course assessment methods by default is more effective than engaging in post-assessment review due to the unreliability of AI detection tools.
Among the many ethical dilemmas that generative AI tools pose to social systems, this paper focused on those pertaining to the pedagogical aspects of higher education. Due to this limitation, related fields, such as university research, were excluded from the scope of the analysis. However, research-related activities are certainly ripe for scientific scrutiny along the lines indicated in this study. Furthermore, only a limited set of institutions could be investigated, those who were the ”first respondents” to the set of issues covered by this study. Hereby, this paper hopes to inspire further research on the impact of AI tools on higher education. Such research could cover more institutions, but it would also be interesting to revisit the same institutions again to see how their stance and approach might have changed over time considering how fast this technology evolves and how much we learn about its capabilities and shortcomings.
Data availability
Data sharing is not applicable to this article as no datasets were generated or analysed during the current study. All documents referenced in this study are publicly available on the corresponding websites provided in the Bibliography or in the footnotes. No code has been developed as part of this research.
For the methodology behind the Shanghai Rankings see: https://www.shanghairanking.com/methodology/arwu/2022 . Accessed: 14 November 2023.
While the original French version was published in 1954, the first English translation is dated 1964.
As the evaluation by Bang et al. ( 2023 ) found, ChatGPT is only 63.41% accurate on average in ten different reasoning categories under logical reasoning, non-textual reasoning, and common-sense reasoning, making it an unreliable reasoner.
Source: https://digital-strategy.ec.europa.eu/en/library/proposal-regulation-laying-down-harmonised-rules-artificial-intelligence . Accessed: 14 November 2023.
Source https://www.europarl.europa.eu/doceo/document/A-9-2021-0127_EN.html . Accessed: 14 November 2023.
Source: https://digital-strategy.ec.europa.eu/en/library/ethics-guidelines-trustworthy-ai . Accessed: 14 November 2023.
Source: https://unesdoc.unesco.org/ark:/48223/pf0000381137 . Accessed: 14 November 2023.
Source: https://legalinstruments.oecd.org/en/instruments/OECD-LEGAL-0449#mainText . Accessed: 14 November 2023.
The editors-in-chief of Nature and Science stated that ChatGPT does not meet the standard for authorship: „ An attribution of authorship carries with it accountability for the work, which cannot be effectively applied to LLMs…. We would not allow AI to be listed as an author on a paper we published, and use of AI-generated text without proper citation could be considered plagiarism,” (Stokel-Walker, 2023 ). See also (Nature, 2023 ).
While there was an initial mistake that credited ChatGPT as an author of an academic paper, Elsevier issued a Corrigendum on the subject in February 2023 (O’Connor, 2023 ). Elsevier then clarified in its “Use of AI and AI-assisted technologies in writing for Elsevier” announcement, issued in March 2023, that “Authors should not list AI and AI-assisted technologies as an author or co-author, nor cite AI as an author”. See https://www.elsevier.com/about/policies-and-standards/the-use-of-generative-ai-and-ai-assisted-technologies-in-writing-for-elsevier . Accessed 23 Nov 2023.
The ethical guidelines of Wiley was updated on 28 February 2023 to clarify the publishing house’s stance on AI-generated content.
See e.g.: Section 2.4 of Princeton University’s Academic Regulations (Princeton University, 2023 ); the Code of Practice and Procedure regarding Misconduct in Research of the University of Oxford (University of Oxford, 2023a ); Section 2.1.1 of the Senate Guidelines on Academic Honesty of York University, enumerating cases of cheating (York University, 2011 ); Imperial College London’s Academic Misconduct Policy and Procedures document (Imperial College London, 2023a ); the Guidelines for seminar and term papers of the University of Vienna (Universität Wien, 2016 ); Para 4. § (1) - (4) of the Anti-plagiarism Regulation of the Corvinus University of Budapest (Corvinus University of Budapest, 2018 ), to name a few.
15 Art. 2 (c)(v) of the early Terms of Use of OpenAI Products (including ChatGPT) dated 14 March 2023 clarified the restrictions of the use of their products. Accordingly, users may not represent the output from their services as human-generated when it was not ( https://openai.com/policies/mar-2023-terms/ . Accessed 14 Nov 2023). Higher education institutions tend to follow suit with this policy. For example, the List of Student Responsibilities enumerated under the “Policies and Regulations” of the Harvard Summer School from 2023 reminds students that their “academic integrity policy forbids students to represent work as their own that they did not write, code, or create” (Harvard University, 2023 ).
A similar view was communicated by Taylor & Francis in a press release issued on 17 February 2023, in which they clarified that: “Authors are accountable for the originality, validity and integrity of the content of their submissions. In choosing to use AI tools, authors are expected to do so responsibly and in accordance with our editorial policies on authorship and principles of publishing ethics” (Taylor and Francis, 2023 ).
This is one of the rare examples where the guideline was adopted by the university’s senior management, in this case, the Academic Affairs Council.
It should be noted that abundant sources recommend harnessing AI tools’ opportunities to improve education instead of attempting to ban them. Heaven, among others, advocated on the pages of the MIT Technology Review the use of advanced chatbots such as ChatGPT as these could be used as “powerful classroom aids that make lessons more interactive, teach students media literacy, generate personalised lesson plans, save teachers time on admin” (Heaven, 2023 ).
This university based its policies on the recommendations of the German Association for University Didactics (Deutsche Gesellschaft für Hochschuldidaktik). Consequently, they draw their students’ attention to the corresponding material, see: (Glathe et al. 2023 ).
For a detailed review of such groups affected by AI see the Artificial Intelligence and Democratic Values Index by the Center for AI and Digital Policy at https://www.caidp.org/reports/aidv-2023/ . Accessed 20 Nov 2023.
See for example: https://www.youtube.com/watch?v=J9W2Pd9GnpQ . Accessed: 14 November 2023.
Akram A (2023) An empirical study of AI generated text detection tools. ArXiv Prepr ArXiv231001423. https://doi.org/10.48550/arXiv.2310.01423
Alberti S (2022) Silas Alberti on X: ChatGPT is trained to not be evil. X Formerly Twitter, 1 December 2022. https://t.co/ZMFdqPs17i . Accessed 23 Nov 2023
Almaraz-López C, Almaraz-Menéndez F, López-Esteban C (2023) Comparative study of the attitudes and perceptions of university students in business administration and management and in education toward Artificial Intelligence. Educ. Sci. 13(6):609. https://doi.org/10.3390/educsci13060609
Article Google Scholar
Bang Y, Cahyawijaya S, Lee N et al. (2023) A multitask, multilingual, multimodal evaluation of ChatGPT on reasoning, hallucination, and interactivity. arXiv. https://doi.org/10.48550/arXiv.2302.04023
Baskara FXR (2023a) ChatGPT as a virtual learning environment: multidisciplinary simulations. In: Proceeding of the 3rd International Conference on Innovations in Social Sciences Education and Engineering, Paper 017. https://conference.loupiasconference.orag/index.php/icoissee3/index
Baskara FXR (2023b) The promises and pitfalls of using ChatGPT for self-determined learning in higher education: An argumentative review. Pros. Semin. Nas. Fakultas Tarb. dan. Ilmu Kegur. IAIM Sinjai 2:95–101. https://doi.org/10.47435/sentikjar.v2i0.1825
Bauer E, Greisel M, Kuznetsov I et al. (2023) Using natural language processing to support peer‐feedback in the age of artificial intelligence: A cross‐disciplinary framework and a research agenda. Br. J. Educ. Technol. 54(5):1222–1245. https://doi.org/10.1111/bjet.13336
Blalock D (2022) Here are all the ways to get around ChatGPT’s safeguards: [1/n]. X Formerly Twitter, 13 December 2022. https://twitter.com/davisblalock/status/1602600453555961856 . Accessed 23 Nov 2023
Chan CKY (2023) A comprehensive AI policy education framework for university teaching and learning. Int J. Educ. Technol. High. Educ. 20(1):1–25. https://doi.org/10.1186/s41239-023-00408-3
Chaudhry IS, Sarwary SAM, El Refae GA, Chabchoub H (2023) Time to revisit existing student’s performance evaluation approach in higher education sector in a new era of ChatGPT—A case study. Cogent Educ. 10(1):2210461. https://doi.org/10.1080/2331186x.2023.2210461
Chaudhry MA, Cukurova M, Luckin R (2022) A transparency index framework for AI in education. In: International Conference on Artificial Intelligence in Education. Springer, Cham, Switzerland, pp 195–198. https://doi.org/10.35542/osf.io/bstcf
Chinese University of Hong Kong (2023) Use of Artificial Intelligence tools in teaching, learning and assessments - A guide for students. https://www.aqs.cuhk.edu.hk/documents/A-guide-for-students_use-of-AI-tools.pdf . Accessed 23 Nov 2023
Colorado State University (2023) What should a syllabus statement on AI look like? https://tilt.colostate.edu/what-should-a-syllabus-statement-on-ai-look-like/ . Accessed 23 Nov 2023
Columbia University (2023) Considerations for AI tools in the classroom. https://ctl.columbia.edu/resources-and-technology/resources/ai-tools/ . Accessed 23 Nov 2023
Corrêa NK, Galvão C, Santos JW et al. (2023) Worldwide AI ethics: A review of 200 guidelines and recommendations for AI governance. Patterns 4(10):100857. https://doi.org/10.1016/j.patter.2023.100857
Article PubMed PubMed Central Google Scholar
Corvinus University of Budapest (2018) Anti-Plagiarism rules. https://www.uni-corvinus.hu/contents/uploads/2020/11/I.20_Plagiumszabalyzat_2018_junius_19_EN.6b1.pdf . Accessed 23 Nov 2023
Cotton DR, Cotton PA, Shipway JR (2024) Chatting and cheating: Ensuring academic integrity in the era of ChatGPT. Innov. Educ. Teach. Int 61(2):228–239. https://doi.org/10.1080/14703297.2023.2190148
Crompton H, Burke D (2023) Artificial intelligence in higher education: the state of the field. Int J. Educ. Technol. High. Educ. 20(1):1–22. https://doi.org/10.1186/s41239-023-00392-8
Dhaor A (2023) India will regulate AI, ensure data privacy, says Rajeev Chandrasekhar. Hindustan Times, 12 October 2023. https://www.hindustantimes.com/cities/noida-news/india-will-regulate-ai-ensure-data-privacy-says-rajeev-chandrasekhar-101697131022456.html . Accessed 23 Nov 2023
Ellul J (1964) The technological society. Vintage Books
EUHLEX (2019) Ethics guidelines for trustworthy AI | Shaping Europe’s digital future. https://digital-strategy.ec.europa.eu/en/library/ethics-guidelines-trustworthy-ai . Accessed 23 Nov 2023
European Commission (2021) Proposal for a Regulation laying down harmonised rules on artificial intelligence | Shaping Europe’s digital future. https://digital-strategy.ec.europa.eu/en/library/proposal-regulation-laying-down-harmonised-rules-artificial-intelligence . Accessed 23 Nov 2023
European Parliament - Committee on Culture and Education (2021) Report on artificial intelligence in education, culture and the audiovisual sector | A9-0127/2021. https://www.europarl.europa.eu/doceo/document/A-9-2021-0127_EN.html . Accessed 23 Nov 2023
Farazouli A, Cerratto-Pargman T, Bolander-Laksov K, McGrath C (2024) Hello GPT! Goodbye home examination? An exploratory study of AI chatbots impact on university teachers’ assessment practices. Assess. Eval. High. Educ. 49(3):363–375. https://doi.org/10.1080/02602938.2023.2241676
Farrokhnia M, Banihashem SK, Noroozi O, Wals A (2023) A SWOT analysis of ChatGPT: Implications for educational practice and research. Innov. Educ. Teach. Int 61(3):460–474. https://doi.org/10.1080/14703297.2023.2195846
Ferguson R, Hoel T, Scheffel M, Drachsler H (2016) Guest editorial: Ethics and privacy in learning analytics. J. Learn Anal. 3(1):5–15. https://doi.org/10.18608/jla.2016.31.2
Future of Life Institute (2023) Pause giant AI experiments: An open letter. https://futureoflife.org/open-letter/pause-giant-ai-experiments/ . Accessed 15 Nov 2023
Gamage KA, Dehideniya SC, Xu Z, Tang X (2023) ChatGPT and higher education assessments: more opportunities than concerns? J Appl Learn Teach 6(2). https://doi.org/10.37074/jalt.2023.6.2.32
Gimpel H, Hall K, Decker S, et al. (2023) Unlocking the power of generative AI models and systems such as GPT-4 and ChatGPT for higher education: A guide for students and lecturers. Hohenheim Discussion Papers in Business, Economics and Social Sciences 2023, 02:2146. http://opus.uni-hohenheim.de/frontdoor.php?source_opus=2146&la=en
Glathe A, Mörth M, Riedel A (2023) Vorschläge für Eigenständigkeitserklärungen bei möglicher Nutzung von KI-Tools. European University Viadrina. https://opus4.kobv.de/opus4-euv/files/1326/Forschendes-Lernen-mit-KI_SKILL.pdf . Accessed 23 Nov 2023
Harvard University (2023) Student Responsibilities. Harvard Summer School 2023. https://summer.harvard.edu/academic-opportunities-support/policies-and-regulations/student-responsibilities/ . Accessed 23 Nov 2023
Heaven WD (2023) ChatGPT is going to change education, not destroy it. MIT Technology Review. https://www.technologyreview.com/2023/04/06/1071059/chatgpt-change-not-destroy-education-openai/ . Accessed 14 Nov 2023
Hillier M (2023) Turnitin Artificial Intelligence writing detection. https://teche.mq.edu.au/2023/03/turnitin-artificial-intelligence-writing-detection/ . Accessed 23 Nov 2023
Howard SK, Mozejko A (2015) Considering the history of digital technologies in education. In: Henderson M, Romeo G (eds) Teaching and digital technologies: Big issues and critical questions. Cambridge University Press, Port Melbourne, Australia, pp 157–168. https://doi.org/10.1017/cbo9781316091968.017
Humboldt-Universität zu Berlin (2023a) ChatGPT & Co: Empfehlungen für das Umgehen mit Künstlicher Intelligenz in Prüfungen. https://www.hu-berlin.de/de/pr/nachrichten/september-2023/nr-2397-1 . Accessed 23 Nov 2023
Humboldt-Universität zu Berlin (2023b) Empfehlungen zur Nutzung von Künstlicher Intelligenz in Studienleistungen und Prüfungen an der Humboldt-Universität zu Berlin. https://www.hu-berlin.de/de/pr/nachrichten/september-2023/hu_empfehlungen_ki-in-pruefungen_20230905.pdf . Accessed 23 Nov 2023
Ilieva G, Yankova T, Klisarova-Belcheva S et al. (2023) Effects of generative chatbots in higher education. Information 14(9):492. https://doi.org/10.3390/info14090492
Imperial College London (2023a) Academic misconduct policy and procedure. https://www.imperial.ac.uk/media/imperial-college/administration-and-support-services/registry/academic-governance/public/academic-policy/academic-integrity/Academic-Misconduct-Policy-and-Procedure-v1.3-15.03.23.pdf . Accessed 14 Nov 2023
Imperial College London (2023b) College guidance on the use of generative AI tools. https://www.imperial.ac.uk/about/leadership-and-strategy/provost/vice-provost-education/generative-ai-tools-guidance/ . Accessed 23 Nov 2023
Ivanov S (2023) The dark side of artificial intelligence in higher education. Serv. Ind. J. 43(15–16):1055–1082. https://doi.org/10.1080/02642069.2023.2258799
Johns Hopkins University (2023) CSCI 601.771: Self-supervised Models. https://self-supervised.cs.jhu.edu/sp2023/ . Accessed 23 Nov 2023
Khosravi H, Shum SB, Chen G et al. (2022) Explainable artificial intelligence in education. Comput Educ. Artif. Intell. 3:100074. https://doi.org/10.1016/j.caeai.2022.100074
Liao SM (2020) The moral status and rights of Artificial Intelligence. In: Liao SM (ed) Ethics of Artificial Intelligence. Oxford University Press, pp 480–503. https://doi.org/10.1093/oso/9780190905033.003.0018
Lim T, Gottipati S, Cheong M (2023) Artificial Intelligence in today’s education landscape: Understanding and managing ethical issues for educational assessment. Research Square Preprint. https://doi.org/10.21203/rs.3.rs-2696273/v1
Limo FAF, Tiza DRH, Roque MM et al. (2023) Personalized tutoring: ChatGPT as a virtual tutor for personalized learning experiences. Soc. Space 23(1):293–312. https://socialspacejournal.eu/article-page/?id=176
Google Scholar
Liu S (2023) India’s AI Regulation Dilemma. The Diplomat, 27 October 2023. https://thediplomat.com/2023/10/indias-ai-regulation-dilemma/ . Accessed 23 Nov 2023
Macquarie University (2023) Academic integrity vs the other AI (Generative Artificial Intelligence). https://teche.mq.edu.au/2023/03/academic-integrity-vs-the-other-ai-generative-artificial-intelligence/ . Accessed 14 Nov 2023
Memarian B, Doleck T (2023) Fairness, Accountability, Transparency, and Ethics (FATE) in Artificial Intelligence (AI), and higher education: A systematic review. Comput Educ Artif Intell 100152. https://doi.org/10.1016/j.caeai.2023.100152
Mhlanga D (2023) Open AI in Education, the Responsible and Ethical Use of ChatGPT Towards Lifelong Learning. SSRN Electron J 4354422. https://doi.org/10.2139/ssrn.4354422
Miller GE (2023) eLearning and the Transformation of Higher Education. In: Miller GE, Ives K (eds) Leading the eLearning Transformation of Higher Education. Routledge, pp 3–23. https://doi.org/10.4324/9781003445623-3
Mollick ER, Mollick L (2022) New modes of learning enabled by AI chatbots: Three methods and assignments. SSRN Electron J 4300783. https://doi.org/10.2139/ssrn.4300783
Monash University (2023a) Generative AI and assessment: Designing assessment for achievement and demonstration of learning outcomes. https://www.monash.edu/learning-teaching/teachhq/Teaching-practices/artificial-intelligence/generative-ai-and-assessment . Accessed 23 Nov 2023
Monash University (2023b) Policy and practice guidance around acceptable and responsible use of AI technologies. https://www.monash.edu/learning-teaching/teachhq/Teaching-practices/artificial-intelligence/policy-and-practice-guidance-around-acceptable-and-responsible-use-of-ai-technologies . Accessed 23 Nov 2023
Monash University (2023c) Choosing assessment tasks. https://www.monash.edu/learning-teaching/teachhq/Assessment/choosing-assessment-tasks . Accessed 23 Nov 2023
National Autonomous University of Mexico (2023) Recomendaciones para el uso de Inteligencia Artificial Generativa en la docencia. https://cuaed.unam.mx/descargas/recomendaciones-uso-iagen-docencia-unam-2023.pdf . Accessed 14 Oct 2023
Nature (2023) Tools such as ChatGPT threaten transparent science; here are our ground rules for their use. Nature 613:612. https://doi.org/10.1038/d41586-023-00191-1 . Editorial
Article CAS Google Scholar
Nicol DJ, Macfarlane‐Dick D (2006) Formative assessment and self‐regulated learning: A model and seven principles of good feedback practice. Stud. High. Educ. 31(2):199–218. https://doi.org/10.1080/03075070600572090
Nikolinakos NT (2023) Ethical Principles for Trustworthy AI. In: Nikolinakos NT (ed) EU Policy and Legal Framework for Artificial Intelligence, Robotics and Related Technologies -The AI Act. Springer International Publishing, Cham, Switzerland, pp 101–166. https://doi.org/10.1007/978-3-031-27953-9
O’Connor S (2023) Corrigendum to “Open artificial intelligence platforms in nursing education: Tools for academic progress or abuse?” [Nurse Educ. Pract. 66 (2023) 103537]. Nurse Educ. Pr. 67:103572. https://doi.org/10.1016/j.nepr.2023.103572
OECD (2019) Recommendation of the Council on Artificial Intelligence. https://legalinstruments.oecd.org/en/instruments/OECD-LEGAL-0449#mainText . Accessed 23 Nov 2023
OpenAI (2022) Introducing ChatGPT. https://openai.com/blog/chatgpt . Accessed 14 Nov 2022
Paek S, Kim N (2021) Analysis of worldwide research trends on the impact of artificial intelligence in education. Sustainability 13(14):7941. https://doi.org/10.3390/su13147941
Perkins M (2023) Academic Integrity considerations of AI Large Language Models in the post-pandemic era: ChatGPT and beyond. J. Univ. Teach. Learn Pr. 20(2):07. https://doi.org/10.53761/1.20.02.07
Princeton University (2023) Academic Regulations: Rights, rules, responsibilities. https://rrr.princeton.edu/2023/students-and-university/24-academic-regulations . Accessed 23 Nov 2023
Qadir J (2023) Engineering education in the era of ChatGPT: Promise and pitfalls of generative AI for education. In: 2023 IEEE Global Engineering Education Conference (EDUCON). IEEE, pp 1–9. https://doi.org/10.1109/educon54358.2023.10125121
Rasul T, Nair S, Kalendra D et al. (2023) The role of ChatGPT in higher education: Benefits, challenges, and future research directions. J. Appl Learn Teach. 6(1):41–56. https://doi.org/10.37074/jalt.2023.6.1.29
Ray PP (2023) ChatGPT: A comprehensive review on background, applications, key challenges, bias, ethics, limitations and future scope. Internet Things Cyber-Phys. Syst. 3:121–154. https://doi.org/10.1016/j.iotcps.2023.04.003
Reiser RA (2001) A history of instructional design and technology: Part I: A history of instructional media. Educ. Technol. Res Dev. 49(1):53–64. https://doi.org/10.1007/BF02504506
Roose K (2023) GPT-4 is exciting and scary. New York Times, 15 March 2023. https://www.nytimes.com/2023/03/15/technology/gpt-4-artificial-intelligence-openai.html . Accessed 23 Nov 2023
Rudolph J, Tan S, Tan S (2023) War of the chatbots: Bard, Bing Chat, ChatGPT, Ernie and beyond. The new AI gold rush and its impact on higher education. J. Appl Learn Teach. 6(1):364–389. https://doi.org/10.37074/jalt.2023.6.1.23
Solis T (2023) Die ChatGPT-Richtlinien der 100 größten deutschen Universitäten. Scribbr, 6 May 2023. https://www.scribbr.de/ki-tools-nutzen/chatgpt-universitaere-richtlinien/ . Accessed 23 Nov 2023
Stokel-Walker C (2023) ChatGPT listed as author on research papers: Many scientists disapprove. Nature 613:620–621. https://doi.org/10.1038/d41586-023-00107-z
Article ADS CAS PubMed Google Scholar
Sweeney S (2023) Who wrote this? Essay mills and assessment – Considerations regarding contract cheating and AI in higher education. Int J. Manag Educ. 21(2):100818. https://doi.org/10.1016/j.ijme.2023.100818
Taylor and Francis (2023) Taylor & Francis clarifies the responsible use of AI tools in academic content creation. Taylor Francis Newsroom, 17 February 2023. https://newsroom.taylorandfrancisgroup.com/taylor-francis-clarifies-the-responsible-use-of-ai-tools-in-academic-content-creation/ . Accessed 23 Nov 2023
Technische Universität Berlin (2023) Inklusive Digitalisierung Modul. https://moseskonto.tu-berlin.de/moses/modultransfersystem/bolognamodule/beschreibung/anzeigen.html?nummer=51021&version=2&sprache=1 . Accessed 05 Aug 2024
Tokyo Institute of Technology (2023) Policy on Use of Generative Artificial Intelligence in Learning. https://www.titech.ac.jp/english/student/students/news/2023/066592.html . Accessed 23 Nov 2023
Turnitin (2023) Turnitin announces AI writing detector and AI writing resource center for educators. https://www.turnitin.com/press/turnitin-announces-ai-writing-detector-and-ai-writing-resource-center-for-educators . Accessed 14 Nov 2023
Uchiyama S, Umemura K, Morita Y (2023) Large Language Model-based system to provide immediate feedback to students in flipped classroom preparation learning. ArXiv Prepr ArXiv230711388. https://doi.org/10.48550/arXiv.2307.11388
UNESCO (2022) Recommendation on the ethics of Artificial Intelligence. https://unesdoc.unesco.org/ark:/48223/pf0000381137 . Accessed 23 Nov 2023
UNESCO International Institute for Higher Education in Latin America and the Caribbean (2023) ChatGPT and Artificial Intelligence in higher education. https://www.iesalc.unesco.org/wp-content/uploads/2023/04/ChatGPT-and-Artificial-Intelligence-in-higher-education-Quick-Start-guide_EN_FINAL.pdf . Accessed 14 Nov 2023
Universität Wien (2016) Guidelines for seminar and term papers. https://bda.univie.ac.at/fileadmin/user_upload/p_bda/Teaching/PaperGuidlines.pdf . Accessed 23 Nov 2023
University of Auckland (2023) Advice for students on using Generative Artificial Intelligence in coursework. https://www.auckland.ac.nz/en/students/forms-policies-and-guidelines/student-policies-and-guidelines/academic-integrity-copyright/advice-for-student-on-using-generative-ai.html . Accessed 24 Nov 2023
University of Boston (2023) Using Generative AI in coursework. https://www.bu.edu/cds-faculty/culture-community/gaia-policy/ . Accessed 23 Nov 2023
University of Cambridge (2023) Artificial Intelligence and teaching, learning and assessment. https://www.cambridgeinternational.org/support-and-training-for-schools/artificial-intelligence/ . Accessed 23 Nov 2023
University of Cape Town (2023) Staff Guide - Assessment and academic integrity in the age of AI. https://docs.google.com/document/u/0/d/1o5ZIOBjPsP6Nh2VIlM56_kcuqB-Y7xTf/edit?pli=1&usp=embed_facebook . Accessed 14 Nov 2023
University of Delaware (2023) Considerations for using and addressing advanced automated tools in coursework and assignments. https://ctal.udel.edu/advanced-automated-tools/ . Accessed 14 Nov 2023
University of Helsinki (2023) Using AI to support learning | Instructions for students. https://studies.helsinki.fi/instructions/article/using-ai-support-learning . Accessed 24 Nov 2023
University of Oxford (2023a) Code of practice and procedure on academic integrity in research. https://hr.admin.ox.ac.uk/academic-integrity-in-research . Accessed 23 Nov 2023
University of Oxford (2023b) Unauthorised use of AI in exams and assessment. https://academic.admin.ox.ac.uk/article/unauthorised-use-of-ai-in-exams-and-assessment . Accessed 23 Nov 2023
University of Pittsburgh (2023) Generative AI Resources for Faculty. https://teaching.pitt.edu/generative-ai-resources-for-faculty/ . Accessed 23 Nov 2023
University of Toronto (2019) Code of behaviour on academic matters. https://governingcouncil.utoronto.ca/secretariat/policies/code-behaviour-academic-matters-july-1-2019 . Accessed 23 Nov 2023
University of Toronto (2023) ChatGPT and Generative AI in the classroom. https://www.viceprovostundergrad.utoronto.ca/strategic-priorities/digital-learning/special-initiative-artificial-intelligence/ . Accessed 20 Nov 2023
University of Waterloo (2023) Artificial Intelligence at UW. https://uwaterloo.ca/associate-vice-president-academic/artificial-intelligence-uw . Accessed 23 Nov 2023
University of Zürich (2023) ChatGPT. https://ethz.ch/en/the-eth-zurich/education/educational-development/ai-in-education/chatgpt.html . Accessed 23 Nov 2023
Wach K, Duong CD, Ejdys J et al. (2023) The dark side of generative artificial intelligence: A critical analysis of controversies and risks of ChatGPT. Entrep. Bus. Econ. Rev. 11(2):7–24. https://doi.org/10.15678/eber.2023.110201
Wagner G (2018) Robot liability. SSRN Electron J 3198764. https://doi.org/10.2139/ssrn.3198764
Wiley (2023) Best practice guidelines on research integrity and publishing ethics. https://authorservices.wiley.com/ethics-guidelines/index.html . Accessed 20 Nov 2023
Yan L, Sha L, Zhao L et al. (2023) Practical and ethical challenges of large language models in education: A systematic scoping review. Br. J. Educ. Technol. 55(1):90–112. https://doi.org/10.1111/bjet.13370
York University (2011) Senate Policy on Academic Honesty. https://www.yorku.ca/secretariat/policies/policies/academic-honesty-senate-policy-on/ . Accessed 23 Nov 2023
York University Senate (2023) Academic Integrity and Generative Artificial Intelligence Technology. https://www.yorku.ca/unit/vpacad/academic-integrity/wp-content/uploads/sites/576/2023/03/Senate-ASCStatement_Academic-Integrity-and-AI-Technology.pdf . Accessed 23 Nov 2023
Yu H (2023) Reflection on whether Chat GPT should be banned by academia from the perspective of education and teaching. Front Psychol. 14:1181712. https://doi.org/10.3389/fpsyg.2023.1181712
Download references
The authors have received no funding, grants, or other support for the research reported here. Open access funding provided by Corvinus University of Budapest.
Author information
Authors and affiliations.
Covinus University of Budapest, Budapest, Hungary
Attila Dabis & Csaba Csáki
You can also search for this author in PubMed Google Scholar
Contributions
AD had established the initial idea and contributed to the collection of ethical standards as well as to the collection of university policy documents. Also contributed to writing the initial draft and the final version. CsCs had reviewed and clarified the initial concept and then developed the first structure including methodological considerations. Also contributed to the collection of university policy documents as well as to writing the second draft and the final version.
Corresponding author
Correspondence to Attila Dabis .
Ethics declarations
Competing interests.
The authors declare no competing interests.
Ethical approval
This research did not involve any human participants or animals and required no ethical approval.
Informed consent
This article does not contain any studies with human participants performed by any of the authors. No consent was required as no private data was collected or utilized.
Additional information
Publisher’s note Springer Nature remains neutral with regard to jurisdictional claims in published maps and institutional affiliations.
Rights and permissions
Open Access This article is licensed under a Creative Commons Attribution 4.0 International License, which permits use, sharing, adaptation, distribution and reproduction in any medium or format, as long as you give appropriate credit to the original author(s) and the source, provide a link to the Creative Commons licence, and indicate if changes were made. The images or other third party material in this article are included in the article’s Creative Commons licence, unless indicated otherwise in a credit line to the material. If material is not included in the article’s Creative Commons licence and your intended use is not permitted by statutory regulation or exceeds the permitted use, you will need to obtain permission directly from the copyright holder. To view a copy of this licence, visit http://creativecommons.org/licenses/by/4.0/ .
Reprints and permissions
About this article
Cite this article.
Dabis, A., Csáki, C. AI and ethics: Investigating the first policy responses of higher education institutions to the challenge of generative AI. Humanit Soc Sci Commun 11 , 1006 (2024). https://doi.org/10.1057/s41599-024-03526-z
Download citation
Received : 21 February 2024
Accepted : 29 July 2024
Published : 06 August 2024
DOI : https://doi.org/10.1057/s41599-024-03526-z
Share this article
Anyone you share the following link with will be able to read this content:
Sorry, a shareable link is not currently available for this article.
Provided by the Springer Nature SharedIt content-sharing initiative
Quick links
- Explore articles by subject
- Guide to authors
- Editorial policies

- Society ›
Education & Science
Education in Russia - statistics & facts
General education in russia, higher education in russia, key insights.
Detailed statistics
Government spending on education as a GDP share in Russia 2010-2022
Number of universities worldwide in 2023, by country
Education Index - comparison of selected countries 2022
Editor’s Picks Current statistics on this topic
Government spending on education in Russia 2022, by level
Number of higher education students in Russia 2010-2022
Educational Institutions & Market
Highest earning EdTech platforms in Russia 2023
Further recommended statistics
- Premium Statistic Number of universities worldwide in 2023, by country
- Premium Statistic Leading countries by R&D spending worldwide 2022
- Premium Statistic Top host destination of international students worldwide 2022
- Premium Statistic International student share of higher-ed population worldwide in 2022, by country
- Premium Statistic Field of study of international students worldwide 2022, by country
- Basic Statistic Pupil-teacher ratio in Russia 2022, by education level
- Premium Statistic Supplementary professional education market size in Russia 2017-2022
Estimated number of universities worldwide as of July 2023, by country
Leading countries by R&D spending worldwide 2022
Leading countries by gross research and development (R&D) expenditure worldwide in 2022 (in billion PPP U.S. dollars)
Top host destination of international students worldwide 2022
Top host destination of international students worldwide in 2022, by number of students
International student share of higher-ed population worldwide in 2022, by country
Countries with the largest amount of international students as a share of the total higher education population in 2022
Field of study of international students worldwide 2022, by country
Field of study of international students worldwide in 2022, by country
Pupil-teacher ratio in Russia 2022, by education level
Average number of students per teacher in Russia in 2022, by educational stage
Supplementary professional education market size in Russia 2017-2022
Market volume of supplementary professional education in Russia from 2017 to 2022 (in million academic hours)
Education spending
- Basic Statistic Spending on education in Russia 2019-2022, by source
- Basic Statistic Government spending on education as a GDP share in Russia 2010-2022
- Basic Statistic Government spending on education in Russia 2022, by level
- Basic Statistic Public education spending per student in Russia 2022, by segment
- Basic Statistic Average consumer prices on education services in Russia 2022
Spending on education in Russia 2019-2022, by source
Education spending in Russia from 2019 to 2022, by source (in billion Russian rubles)
Share of government expenditure on education in gross domestic product (GDP) in Russia from 2010 to 2022
Government expenditure on education in Russia in 2022, by segment (in billion Russian rubles)
Public education spending per student in Russia 2022, by segment
Government expenditure on education per student in Russia in 2022, by stage (in 1,000 Russian rubles)
Average consumer prices on education services in Russia 2022
Average consumer prices on selected types of education services in Russia in 2022 (in Russian rubles)
Preschool & general education
- Premium Statistic Children enrolled in preschool education in Russia 2015-2022
- Basic Statistic Share of children enrolled in preschool education in Russia 2022, by region
- Basic Statistic Number of school students in Russia 2022/2023, by educational stage
- Basic Statistic Number of school students in Russia 2015-2022, by type of area
- Basic Statistic General education institution count in Russia 2014-2022
- Basic Statistic Number of village schools in Russia 2010-2022, by ownership
- Basic Statistic Leading schools in Russia 2022, by graduate competitiveness
- Basic Statistic Unified State Exam average score in Russia 2022, by subject
- Basic Statistic Unified State Exam participants in Russia 2022, by subject
- Basic Statistic Attitude toward the Unified State Exam in Russia 2009-2023
Children enrolled in preschool education in Russia 2015-2022
Number of children enrolled in preschool institutions in Russia from 2015 to 2022 (in millions)
Share of children enrolled in preschool education in Russia 2022, by region
Number of children enrolled in preschool education institutions as a share of total children aged 1-6 years in Russia in 2022, by federal district
Number of school students in Russia 2022/2023, by educational stage
Number of students enrolled in general education institutions in Russia as of the beginning of school year 2022/2023, by stage (in 1,000s)
Number of school students in Russia 2015-2022, by type of area
Number of students in state (municipal) schools in Russia from school year 2015/2016 to 2022/2023, by type of area (in millions)
General education institution count in Russia 2014-2022
Number of primary, basic general, and general secondary education institutions in Russia from school year 2014/2015 to 2022/2023 (in 1,000s)
Number of village schools in Russia 2010-2022, by ownership
Number of state (municipal) and private schools in rural areas in Russia from school year 2010/2011 to 2022/2023
Leading schools in Russia 2022, by graduate competitiveness
Ranking of secondary schools in Russia in 2022, by graduate competitiveness (in points)
Unified State Exam average score in Russia 2022, by subject
Average score in the Unified State Exam achieved by high school graduates in Russia in 2022, by subject (in points)
Unified State Exam participants in Russia 2022, by subject
Number of high school graduates taking the Unified State Exam in Russia in 2022, by subject (in 1,000s)
Attitude toward the Unified State Exam in Russia 2009-2023
What is your opinion on the modern schoolchildren's certification system, the Unified State Exam?
Vocational & higher education
- Basic Statistic Vocational education student count in Russia 2016-2023
- Premium Statistic Number of higher education students in Russia 2010-2022
- Basic Statistic Number of university students in Russia 2014-2022, by degree
- Basic Statistic Number of university students in Russia 2022, by gender and age
- Basic Statistic University graduates employed in their field in Russia 2019-2022
- Basic Statistic Employed university graduates in Russia 2022, by sector & field of study
- Premium Statistic Number of doctoral students in Russia 2019-2023
- Premium Statistic University admission share in Russia 2010-2022, by funding type
- Basic Statistic Leading Russian universities by QS ranking 2024
Vocational education student count in Russia 2016-2023
Number of students enrolled in vocational education programs in Russia from 2016 to 2023 (in 1,000s)
Number of students enrolled in higher education in Russia from 2010 to 2022 (in 1,000s)
Number of university students in Russia 2014-2022, by degree
Number of students enrolled in higher education institutions in Russia from academic year 2014/2015 to 2022/2023, by degree (in 1,000s)
Number of university students in Russia 2022, by gender and age
Number of higher education students in Russia in academic year 2022/2023, by age and gender
University graduates employed in their field in Russia 2019-2022
Number of university graduates working and not working in their field of study in Russia from 2019 to 2022 (in 1,000s)
Employed university graduates in Russia 2022, by sector & field of study
Number of university graduates from 2019 to 2021 who were employed in Russia in 2022, by sector and field of study (in 1,000s)
Number of doctoral students in Russia 2019-2023
Number of doctoral students in Russia from 2019 to 2023
University admission share in Russia 2010-2022, by funding type
Distribution of admissions into higher education institutions in Russia from school year 2010/2011 to 2022/2023, by tuition funding type
Leading Russian universities by QS ranking 2024
Leading universities in Russia by rank in the QS World University Rankings 2024
Teaching personnel
- Basic Statistic Education employment in Russia 2023, by segment
- Basic Statistic Number of teachers in Russia 2022, by educational stage
- Basic Statistic School teacher count in Russia 2022, by specialization
- Basic Statistic University employee age distribution in Russia 2022, by position
- Basic Statistic Monthly salary of teachers in Russia 2022, by education segment
Education employment in Russia 2023, by segment
Number of employees in the education sector in Russia in the academic year 2022/2023, by segment (n 1,000s)
Number of teachers in Russia 2022, by educational stage
Number of teaching personnel in education system in Russia in 2022, by segment (n 1,000s)
School teacher count in Russia 2022, by specialization
Number of school teachers in schools in Russia in school year 2022/2023, by specialization
University employee age distribution in Russia 2022, by position
Distribution of higher education employees in Russia in school year 2022/2023, by age group and position
Monthly salary of teachers in Russia 2022, by education segment
Average monthly salary of teaching personnel in Russia in 2022, by educational stage (In Russian rubles)
- Premium Statistic Online education revenue in Russia 2018-2028, by segment
- Premium Statistic Online education users in Russia 2018-2028, by segment
- Premium Statistic Highest earning EdTech platforms in Russia 2023
- Premium Statistic Most visited online education platforms in Russia 2023
- Premium Statistic Most visited educational websites in Russia 2023
Online education revenue in Russia 2018-2028, by segment
Revenue of online education in Russia from 2018 to 2028, by segment (in million U.S. dollars)
Online education users in Russia 2018-2028, by segment
Number of users of online education in Russia from 2018 to 2028, by segment (in millions)
Leading EdTech platforms in Russia in 4th quarter 2023, by revenue (in billion Russian rubles)
Most visited online education platforms in Russia 2023
Leading online education platforms in Russia in August 2023, by monthly visits (in millions)
Most visited educational websites in Russia 2023
Leading educational websites in Russia in August 2023, by monthly visits (in millions)
Further reports
Get the best reports to understand your industry.
- Education in Germany
- Education worldwide
- Education in Turkey
- Higher education worldwide
Mon - Fri, 9am - 6pm (EST)
Mon - Fri, 9am - 5pm (SGT)
Mon - Fri, 10:00am - 6:00pm (JST)
Mon - Fri, 9:30am - 5pm (GMT)
Advertisement
19 Facts About Tim Walz, Harris’s Pick for Vice President
Mr. Walz, the governor of Minnesota, worked as a high school social studies teacher and football coach, served in the Army National Guard and chooses Diet Mountain Dew over alcohol.
- Share full article

By Simon J. Levien and Maggie Astor
- Published Aug. 6, 2024 Updated Aug. 9, 2024, 8:04 a.m. ET
Until recently, Gov. Tim Walz of Minnesota was a virtual unknown outside of the Midwest, even among Democrats. But his stock rose fast in the days after President Biden withdrew from the race, clearing a path for Ms. Harris to replace him and pick Mr. Walz as her No. 2.
Here’s a closer look at the Democrats’ new choice for vice president.
1. He is a (very recent) social media darling . Mr. Walz has enjoyed a groundswell of support online from users commenting on his Midwestern “dad vibes” and appealing ordinariness.
2. He started the whole “weird” thing. It was Mr. Walz who labeled former President Donald J. Trump and his running mate, Senator JD Vance of Ohio, “weird” on cable television just a couple of weeks ago. The description soon became a Democratic talking point.
3. He named a highway after Prince and signed the bill in purple ink. “I think we can lay to rest that this is the coolest bill signing we’ll ever do,” he said as he put his name on legislation declaring a stretch of Highway 5 the “Prince Rogers Nelson Memorial Highway” after the musician who had lived in Minnesota.
4. He reminds you of your high school history teacher for a reason. Mr. Walz taught high school social studies and geography — first in Alliance, Neb., and then in Mankato, Minn. — before entering politics.
5. He taught in China in 1989 and speaks some Mandarin. He went to China for a year after graduating from college and taught English there through a program affiliated with Harvard University.
We are having trouble retrieving the article content.
Please enable JavaScript in your browser settings.
Thank you for your patience while we verify access. If you are in Reader mode please exit and log into your Times account, or subscribe for all of The Times.
Thank you for your patience while we verify access.
Already a subscriber? Log in .
Want all of The Times? Subscribe .

COMMENTS
Easily distribute, analyze, and grade student work with Assignments for your LMS. Assignments is an application for your learning management system (LMS). It helps educators save time grading and guides students to turn in their best work with originality reports — all through the collaborative power of Google Workspace for Education. Get ...
Google for Education offers tools for teaching and learning, such as Google Workspace, Classroom, and Chromebooks. Find out how to use these tools together, get support, and access free educational resources.
Designing Assignments for Learning. The rapid shift to remote teaching and learning meant that many instructors reimagined their assessment practices. Whether adapting existing assignments or creatively designing new opportunities for their students to learn, instructors focused on helping students make meaning and demonstrate their learning ...
Create assignments for individual learners, an entire household, or a classroom-with insights to celebrate each student's achievements and milestones. ... Education.com has multiple resources organized for any learning tool you might need as a teacher, parent, and student, and I love the ability to be able to sort by grade, subject ...
Your teaching philosophy should be 2-3 pages in length and written in first person and in present tense. It should state your goal of education and several ideas you have about how to reach that goal. You will want to include examples and descriptions so your reader can "see" you in your classroom—these may be specific teaching strategies ...
See how Assignments can help you easily distribute, analyze, and grade student work. Learn more. Assignments, an application for your learning management system, gives educators a faster, simpler way to distribute, analyze, and grade student work - all while using the collaborative power of Google Workspace.
How to Assign Worksheets, Games or Exercises: - Select the content you'd like to assign, for example a worksheet, game, exercise, or Guided Lesson. - Click on the "Assign Digitally" button. - From here, you have two options: Add to an existing Assignment or Add to a new Assignment. - If you select " Create new Assignment ...
Assignments brings together the capabilities of Google Docs, Drive and Search into a new tool for collecting and grading student work. It helps you save time with streamlined assignment workflows, ensure student work is authentic with originality reports, and give constructive feedback with comment banks. You can use Assignments as a standalone ...
Assignments is an add-on application for learning management systems (LMSs) to help you distribute, analyze, and grade student work with Google Workspace for Education. For file submissions, Assignments make Google Docs, Google Sheets, Google Slides, and Google Drive compatible with your LMS.
About Assignments LTI™. Assignments is an add-on application for learning management systems (LMSs) that helps you distribute, analyze, and grade student work with Google Workspace for Education. Assignments makes Google Docs and Google Drive compatible with your LMS for file submissions. You can use Assignments to save time distributing and ...
Powerful Data. Track student progress to learn how to better help them succeed. Assignments help you identify, preview, and assign activities that best fit your child, while you're sitting at home or on the go. Activities show up in the Assignments section of Brainzy, the child-facing app. You can keep track of progress in My Assignments.
Field assignments ; Field assignments involve collecting data, interviews, and observations. It may also involve a visit to historical sites, watching games, and observing phenomena like the launch of a rocket. It is a type of education assignment that brings the student face to face with an actual situation.
Free Worksheets and Printables for Kids. 26,693 results. Interactive Worksheets bring printable worksheets to life! Students can complete worksheets online, and get instant feedback to improve. Open an Interactive Worksheet, and create a direct link to share with students. They'll enter their code to access the worksheet, complete it online ...
Advantages of Assignments For Students. Boosts understanding of topics - Assignments help students dive deeper into topics, providing a clear and thorough understanding that goes beyond surface-level knowledge.; Encourages independent learning - They promote self-learning, pushing students to study and solve problems on their own, fostering self-reliance.
The main features of Vygotsky's theory of education. Like Piaget, Vygotsky emphasised learning as a meaning-making process, although Vygotsky's cultural-historical approach is characterised by an insistence that human thinking be understood as both an individual and a socio-cultural phenomenon. While he emphasised social, cultural and ...
Ratings. PDU3701 Notes Final - Summary Philosophy of Education. THEORY OF CRITICAL RATIONALISM, Main Objective / Aim / Importance of. Multiple Choice Questions Summary... This is a summary on the notes that the lecturer will use. PDU3701 assignment 2 - Summary Philosophy of Education. The Six Major Levels of Bloom's Taxonomy of the Cognitive ...
Keep track of assignment deadlines, exam dates, and course milestones using a planner or digital calendar. Reflect on your strengths and areas for improvement, and adjust your study plan accordingly to stay on course. Seek Support When Needed: Don't hesitate to reach out for support when you encounter challenges or obstacles along the way ...
Knowing what is assignment in education helps teachers assess students better. Importance of Assignments. Giving assignments to the students is a crucial part of student assessment. The importance of giving assignments to the students is discussed in detail below: Learning practical skills Assignments enable students to develop new skills. In ...
Environmental education ( EE) refers to organized efforts to teach how natural environments function, and particularly, how human beings can manage behavior and ecosystems to live sustainably. It is a multi-disciplinary field integrating disciplines such as biology, chemistry, physics, ecology, earth science, atmospheric science, mathematics ...
The UNESCO IITE Analytical survey Volume 3: is a continuation of the fi rst two reports of the Institute's project "ICT in primary education" - Analytical survey Volume 1: Exploring the origins, settings and initiatives (2012), and Analytical survey Volume 2: Policy, Practices, and Recommendations (2014).
Education, which is responsible for hiring and assigning teachers to schools based on subject-specific teaching needs. 2.4 Random Assignment of Teachers and Students to Classes Students and teachers are assigned to classes within each school in a way that results in students' and teachers' random matches.
Enable students to check their own work for recommended citations up to three times before submitting. Help students learn to build on outside ideas and properly incorporate them into their work. Run originality reports in multiple languages. Coming soon Backfill results for your school's corpus of work in originality reports.
The education technology company Chegg, which was a website dedicated to helping students with assignments using a database of collected worksheets and assignments, became one of the most prominent business victims to ChatGPT and other large language models, with CEO Dan Rosensweig stating, in response to his company's stock price nearly being ...
Assignment The practice question assignment described in this article introduces a novel way to promote the generation effect among online pediatric nurse practitioner (PNP) students. The assignment allows students to explore learning using AI technology, promotes critical thinking skills, compels interaction with course content, and fosters ...
Ace your courses with our free study and lecture notes, summaries, exam prep, and other resources
The paper identifies the central ethical imperative that student assignments must reflect individual knowledge acquired during their education, with human individuals retaining moral and legal ...
All four "on assignment" teachers for extensive support needs programs are gone. Fresno Unified is reassigning 13 full-time equivalent educators back into classroom teaching to save $2.1 million.
The University of Wisconsin-Platteville's approach to welcoming veterans into its student body reflects a deep commitment to recognizing the extensive practical experience and dedication that these men and women bring to campus. Understanding the unique challenges of transitioning from military to academic life, UW-Platteville goes beyond conventional support to ensure that veterans, active ...
The popularity of higher education was lower than in the 2000s, though it increased slightly in recent years. Around 4.1 million students were enrolled in Russian universities in 2022, compared to ...
4. He reminds you of your high school history teacher for a reason. Mr. Walz taught high school social studies and geography — first in Alliance, Neb., and then in Mankato, Minn. — before ...